Lifelong nnUNet: a framework for standardized medical continual learning
Research Square (Research Square)(2022)
摘要
Abstract As the acceptability of Deep Learning solutions grows, both medical practitioners and regulatory bodies are exploring ways to safely introduce image segmentation solutions in clinical practice. One frontier to overcome when translating promising research into the clinical open-world is the shift from static to lifelong learning. Continual learning, the practice of training models sequentially with shifting population dynamics, is seeing growing interest but is still at its infancy in healthcare. We present Lifelong nnUNet, a standardized framework for medical image segmentation that places state-of-the art continual segmentation at the hands of researchers and clinicians. Build on top of the popular nnUNet and equipped with all necessary modules for training and testing continual models, we ensure broad applicability and lower the barrier for evaluating new solutions in a sequential fashion. We introduce benchmark results on three medical segmentation use cases with open-source datasets, and provide the tools to quickly test five continual learning methods on further applications.
更多查看译文
关键词
standardized medical continual learning
AI 理解论文
溯源树
样例
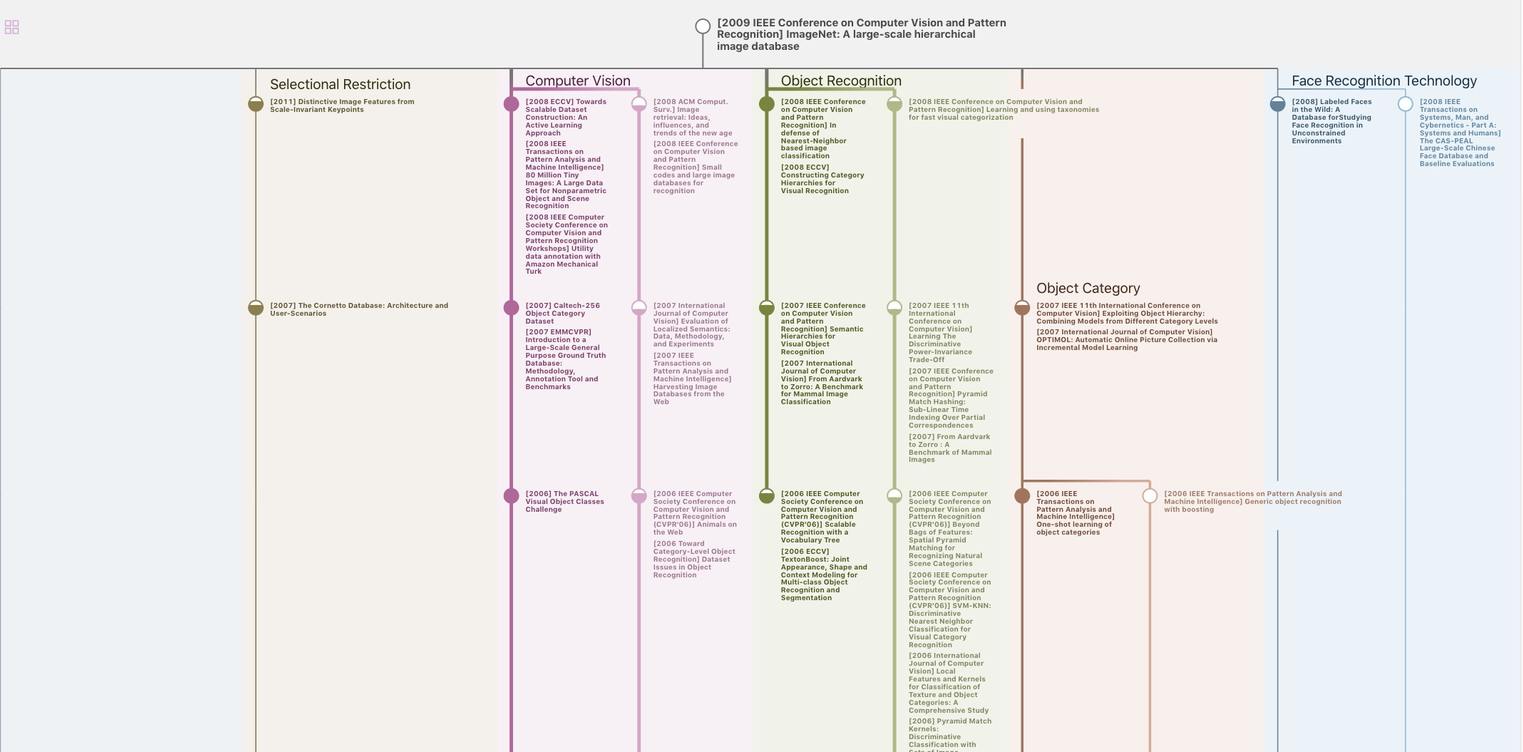
生成溯源树,研究论文发展脉络
Chat Paper
正在生成论文摘要