Context-Aware Attention Encoder-Decoder Network for Connected Heavy-Duty Vehicle Aggressive Driving Identification Under Naturalistic Driving Conditions
IEEE TRANSACTIONS ON INTELLIGENT TRANSPORTATION SYSTEMS(2024)
摘要
Driving behavior analysis and identification are of great significance for improving traffic safety and reducing fuel consumption. Existing methods primarily focused on the driving behavior of light-duty vehicles based on analysis methods using simulation or questionnaire data, while heavy-duty vehicles, which bear significant responsibility for fatal accidents, are seldom investigated. This study develops a context-aware attention encoder-decoder deep framework for aggressive driving identification, utilizing real massive multi-source heterogeneous data collected under naturalistic driving conditions. The proposed framework incorporates vehicle-related, driving-related, weather-related and environment-related data. Through the BiLSTM based encoder-decoder deep architecture, high-level representations of driving behavior are learned from driving signals layer by layer, and temporal dependencies are captured from both forward and backward directions. By learning context-aware personalized latent semantic vectors at different time step, the model is capable of adaptively focusing on the important information for prediction. To our knowledge, the aggressive driving of heavy-duty vehicles under connected and naturalistic driving conditions has been rarely explored. This study contributes to the current understanding in this field. The proposed framework is evaluated based on the multi-source heterogeneous driving behavior data generated from over 13000 vehicles in a connected environment under naturalistic driving conditions. Empirical results from extensive experiments validate that the proposed model outperforms competing models, providing a promising approach with high effectiveness and robustness for aggressive driving behavior identification.
更多查看译文
关键词
Driving behavior,deep learning,attention mechanism,context-aware,heavy-duty vehicle
AI 理解论文
溯源树
样例
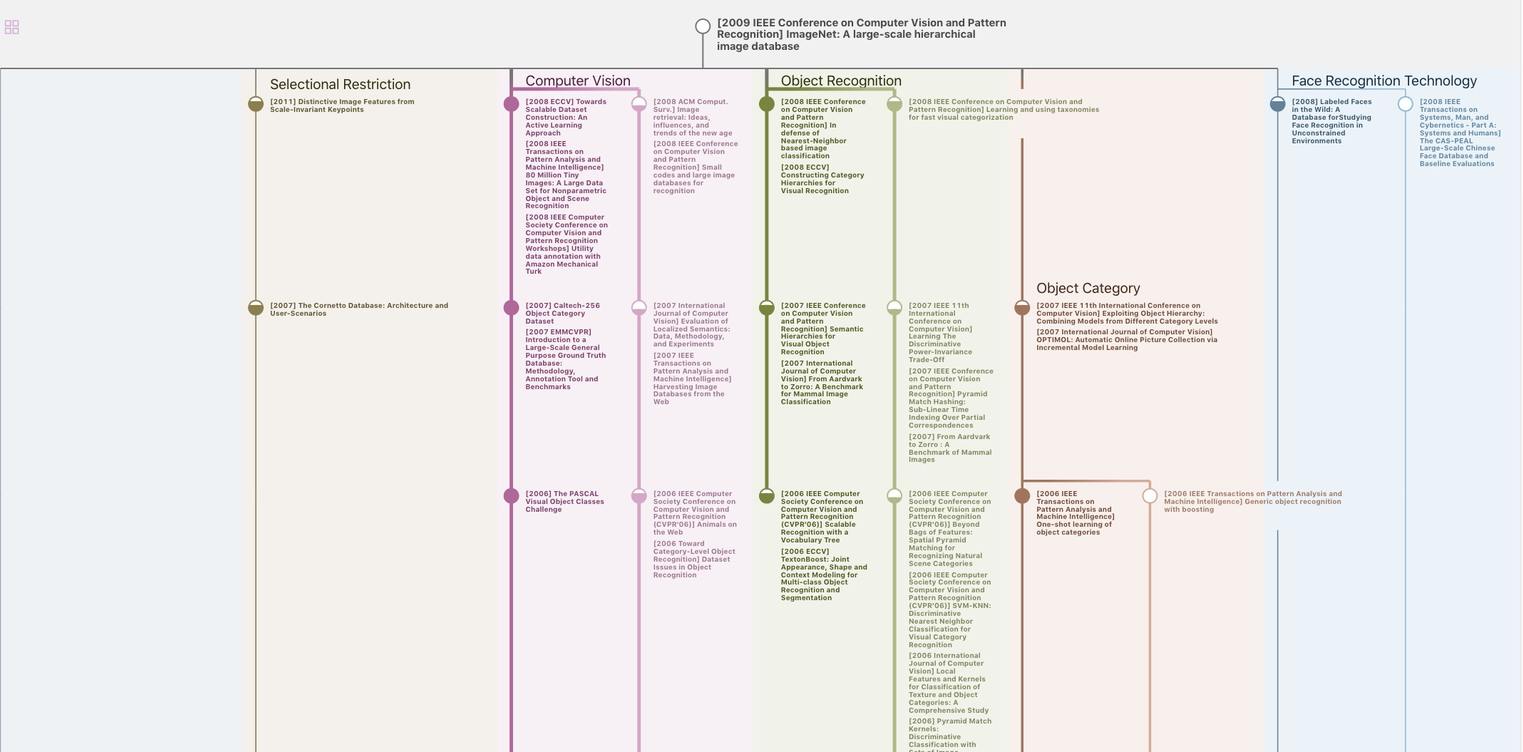
生成溯源树,研究论文发展脉络
Chat Paper
正在生成论文摘要