Evaluation of an automated microscope using machine learning for the detection of malaria in travelers returned to the UK
Research Square (Research Square)(2022)
摘要
Abstract BackgroundLight microscopy remains a standard method for the detection of malaria parasites in clinical cases but training of staff to a WHO level 2 or higher requires considerable time. Moreover, excessive workflow can rapidly lead to fatigue and impact performance, even for a highly skilled technician. An automated microscopy tool that can perform consistent, accurate diagnoses could be an effective aid in clinics with limited access to highly skilled microscopists, where the daily number of cases are excessively high for the number of available technicians or in multi-site studies where consistency between sites is essential. The EasyScan GO is an automated scanning microscope combined with machine learning software designed to detect malaria parasites in field-prepared Giemsa-stained blood films. This study evaluates the performance of the EasyScan GO to determine its ability to detect, quantify and identify the species of parasite present in Giemsa-stained blood films compared with expert light microscopy.MethodsTravelers returning to the UK and testing positive for malaria parasite infection were screened for eligibility and enrolled into the study. Surplus blood samples from enrolled patients were subsequently used to make Giemsa-stained thick and thin slide smears that were assessed by expert light microscopy and the EasyScan GO to determine parasite density and species. Blood samples were also assessed by real time PCR and nested PCR (Snounou) to confirm parasite density and species present and to resolve any discrepancy between manual microscopy and the EasyScan GO.ResultsWhen compared to light microscopy, the EasyScan GO exhibited a sensitivity of 90% (95% CI: 82–95%) and a specificity of 89% (95% CI: 86–90%). Of the 95 samples labelled positive by both light microscopy and the EasyScan GO, manual microscopy identified 83 as Plasmodium falciparum (P. falciparum) and 12 as non-P. falciparum. The EasyScan GO correctly reported P. falciparum for 82 out of the 83 P. falciparum samples and non-P. falciparum for 11 out of 12 non-P. falciparum samples. However, it failed to distinguish between non-P. falciparum species, reporting all non-P. falciparum samples as P. vivax. EasyScan GO calculated parasite densities were within +/-25% of the light microscopy densities for 33% of samples between 200 and 2000 p/µL, falling short of WHO level 1 (expert) manual microscopy competency (50% of samples should be within +/-25% of the true parasitaemia).ConclusionsThis study shows that the EasyScan GO can be proficient in detecting malaria parasites in Giemsa-stained blood films relative to expert manual light microscopy and can accurately distinguish between P. falciparum and non-P. falciparum species. Performance of the device at low parasite densities, distinguishing between non-P. falciparum species and accurate quantitation of parasitaemias require further development and evaluation. Nonetheless, the data shown here suggests that the EasyScan GO could provide assistance as a tool for malaria diagnosis in settings where work load is frequently higher than manageable or where access to expert microscopy is difficult.
更多查看译文
关键词
malaria,microscope,machine learning,detection
AI 理解论文
溯源树
样例
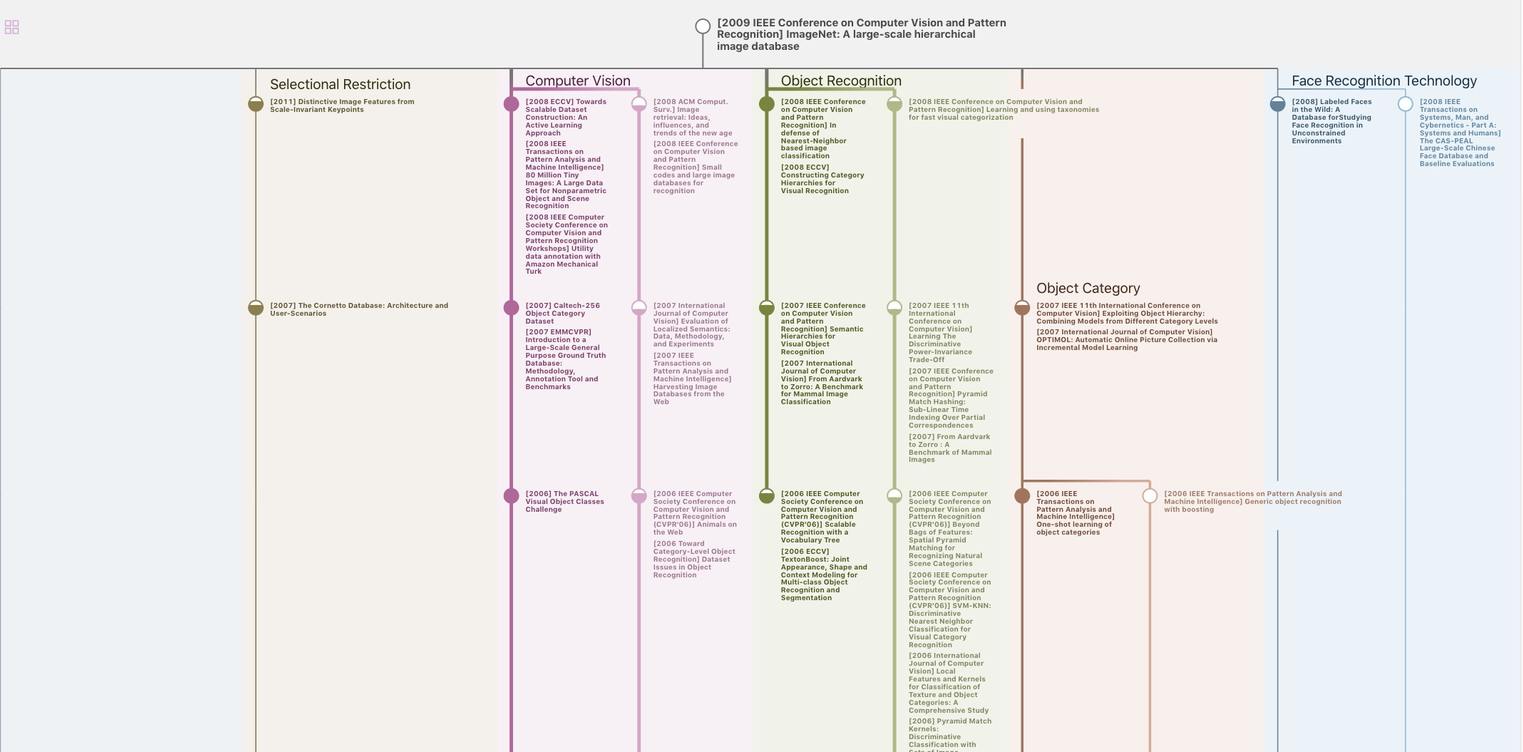
生成溯源树,研究论文发展脉络
Chat Paper
正在生成论文摘要