Detecting Glaucoma from Fundus Photographs Using Deep Learning without Convolutions: Transformer for Improved Generalization
crossref(2022)
Purpose: To compare the diagnostic accuracy and explainability of a new Vision Transformer deep learning technique, Data-efficient image Transformer (DeiT), and Resnet-50, trained on fundus photographs from the Ocular Hypertension Treatment Study (OHTS) to detect primary open-angle glaucoma (POAG) and to identify the salient areas of the photographs most important for each model's decision-making process.
Study Design: Evaluation of a diagnostic technology
Subjects, Participants, and/or Controls: 66,715 photographs from 1,636 OHTS participants and an additional five external datasets of 16137 photographs of healthy and glaucoma eyes.
Methods, Intervention, or Testing: DeiT models were trained to detect five ground truth OHTS POAG classifications: OHTS Endpoint Committee POAG determinations due to disc changes (Model 1), visual field changes (Model 2), or either disc or visual field changes (Model 3) and reading center determinations based on disc (Model 4) and visual fields (Model 5). The best-performing DeiT models were compared to ResNet-50 on OHTS and five external datasets.
Main Outcome Measures: Diagnostic performance was compared using areas under the receiver operating characteristic curve (AUROC) and sensitivities at fixed specificities. The explainability of the DeiT and ResNet-50 models was compared by evaluating the attention maps derived directly from DeiT to 3 gradient-weighted class activation map generation strategies.
Results: Compared to our best-performing ResNet-50 models, the DeiT models demonstrated similar performance on the OHTS test sets for all five-ground truth POAG labels; AUROC ranged from 0.82 (Model 5) to 0.91 (Model 1). However, the AUROC of DeiT was consistently higher than ResNet-50 on the five external datasets. For example, AUROC for the main OHTS endpoint (Model 3) was between 0.08 and 0.20 higher in the DeiT compared to ResNet-50 models. The saliency maps from the DeiT highlight localized areas of the neuroretinal rim, suggesting the use of important clinical features for classification, while the same maps in the ResNet-50 models show a more diffuse, generalized distribution around the optic disc,
Conclusions: Vision transformer has the potential to improve the generalizability and explainability of deep learning models for the detection of eye disease and possibly other medical conditions that rely on imaging modalities for clinical diagnosis and management.
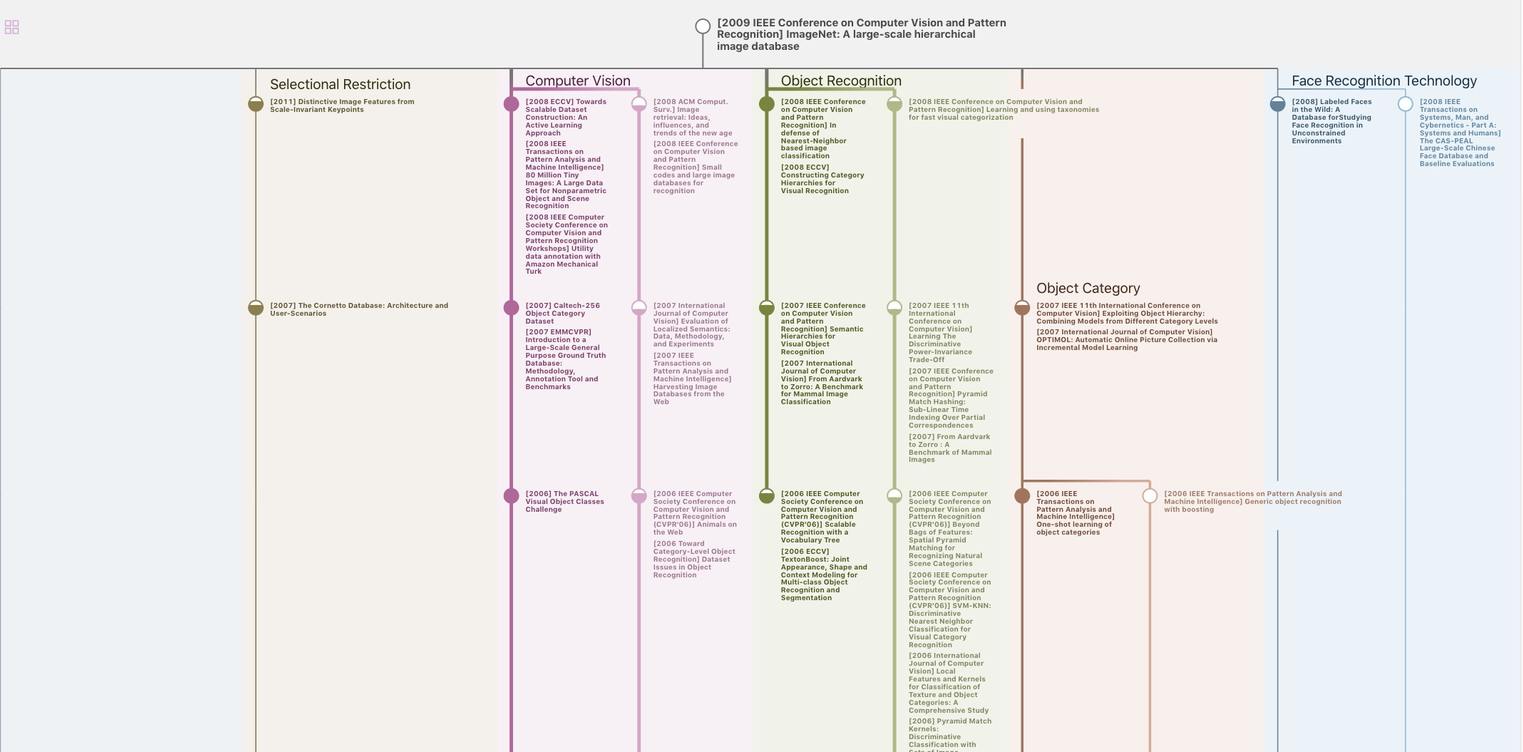