A protocol to evaluate unsupervised text clustering to screen and categorize studies in systematic reviews
Research Square (Research Square)(2022)
摘要
Abstract Background: The evidence base is steadily increasing regarding the time savings of using machine learning (ML) within evidence synthesis, particularly supervised methods such as classification. Unsupervised methods such as clustering have been less explored. Yet clustering – a method to uncover groups of similar data, from a large and heterogenous dataset – may be a particularly relevant tool within the study identification and data extraction processes of reviews, during which researchers must often read thousands of studies to identify the handful relevant to their review. This protocol is for a mixed methods evaluation of clustering implemented within systematic reviews.Research questions: We will answer the following questions: 1) Can clustering while screening at title/abstract level provide useful automatic categorizations of studies that help reviewers extra data to categorize studies? 2) What are reviewers’ perceptions of and experiences with clustering in relation to acceptability and feasibility in future reviews? Methods: We will identify one or two systematic reviews as models to answer these research questions. Reviewers will use the clustering algorithm Lingo3G built into EPPI Reviewer during the screening process. The main outcome to answer research question 1 is content validity, as an indicator of usefulness, defined by similarities between automatic clusters and reviewer-generated categories. We will display multi-class confusion matrices and use these to estimate precision, recall, and F1-score for each useful cluster. Research question 2 will be answered qualitatively, through semi-structured interviews with participating reviewers before and after having been trained on clustering and implementing it in the model review(s), followed by a focus group presenting results and exploring reviewer trust. Conclusion: The planned evaluation will provide important information as to the feasibility of an unsupervised ML method, clustering, in bridging and aligning two currently linear steps in evidence synthesis. We invite interested evidence synthesis organizations to follow this protocol and subsequently share data with us, or to tailor it to produce a more relevant evaluation.
更多查看译文
关键词
systematic reviews,unsupervised text,studies
AI 理解论文
溯源树
样例
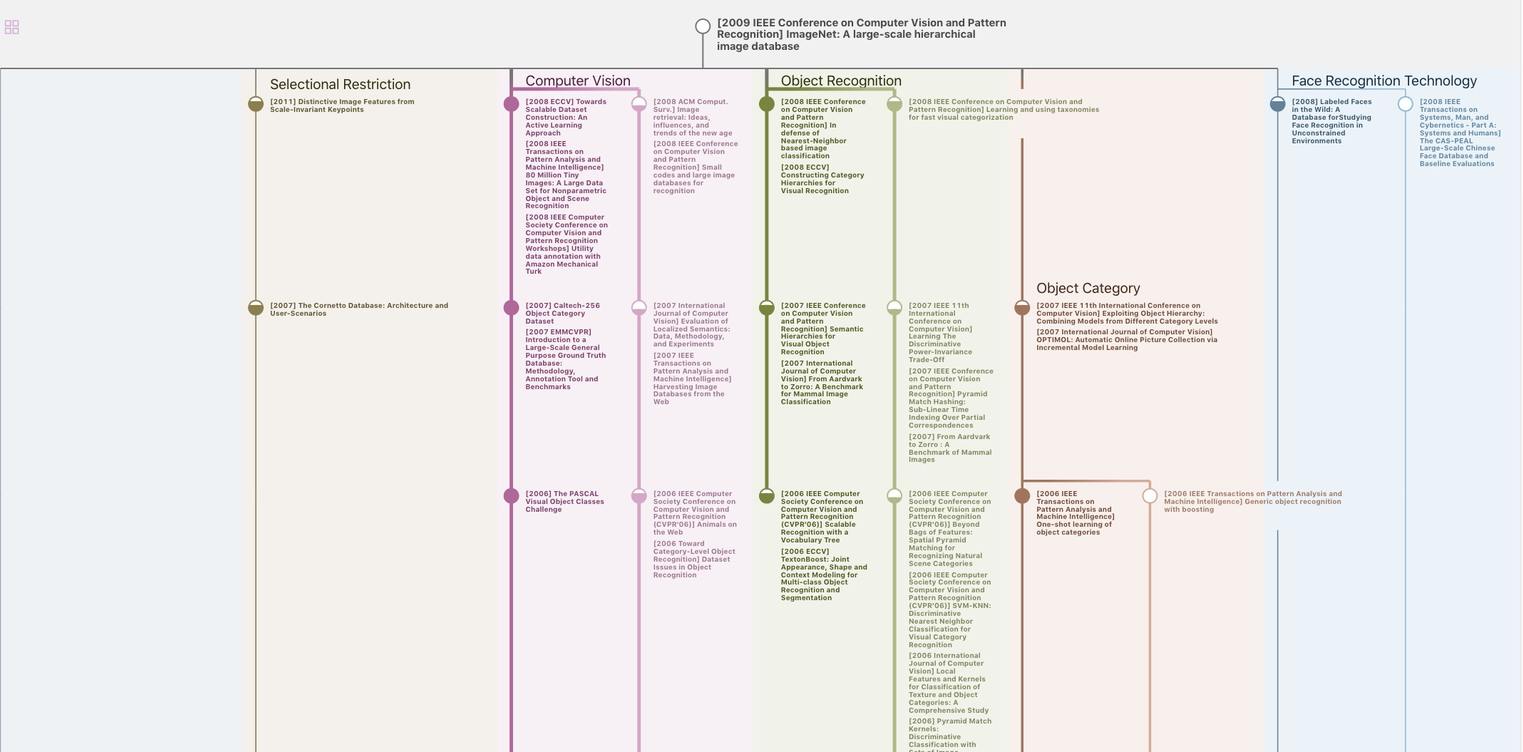
生成溯源树,研究论文发展脉络
Chat Paper
正在生成论文摘要