Understanding greenhouse gas (GHG) column concentrations in Munich using WRF
crossref(2022)
摘要
Abstract. To address ambitious goals of carbon neutrality set at national and city scales, a number of atmospheric networks have been deployed to monitor greenhouse gas (GHG) concentrations in and around cities. To convert these measurements into estimates of emissions from cities, atmospheric models are used to simulate the transport of various tracer gases and help interpret these measurements. We set up a modelling framework using the Weather Research and Forecasting (WRF) model applied at a high spatial resolution (up to 400 m) to simulate the atmospheric transport of GHGs and interpret the observations provided by the Munich Urban Carbon Column Network (MUCCnet). Building on previous analyses using similar measurements performed within a campaign for the city of Berlin and its surroundings (Zhao et al., 2019), our modelling framework has been improved regarding the initialization of tagged tracers, model settings, and input data. To assess the model performance, we validate the modelled output against two local weather stations and observed column GHG concentrations provided by MUCCnet from 1 to 30 August 2018. The modelled wind matches well with the measurements from the weather stations, with wind speeds slightly overestimated. The measured slant column concentrations of GHGs and their variability are generally reproduced by the model, with a bias in CO2 of around 3.7 ppm that can be attributed to the initial and boundary conditions used. The differential column method (DCM) has been applied to cancel out the influence from the background concentrations of CO2. We optimize its application by selecting suitable days on which the assumption of the DCM holds true: a relatively uniform air mass travels over the city, passing from an upwind site to a downwind site. In particular, the Stochastic Time-Inverted Lagrangian Transport model (STILT) is used here and driven by our WRF modelled meteorological fields to obtain footprints (i.e., the potential areas of influence for signals observed at specific points), further used for interpreting measurement results. Combining these footprints with knowledge of local emission sources, we find evidence of CH4 sources near Munich that are missing or underestimated in the emission inventory used. This demonstrates the potential of this data-model framework to constrain local sources and improve emission inventories.
更多查看译文
AI 理解论文
溯源树
样例
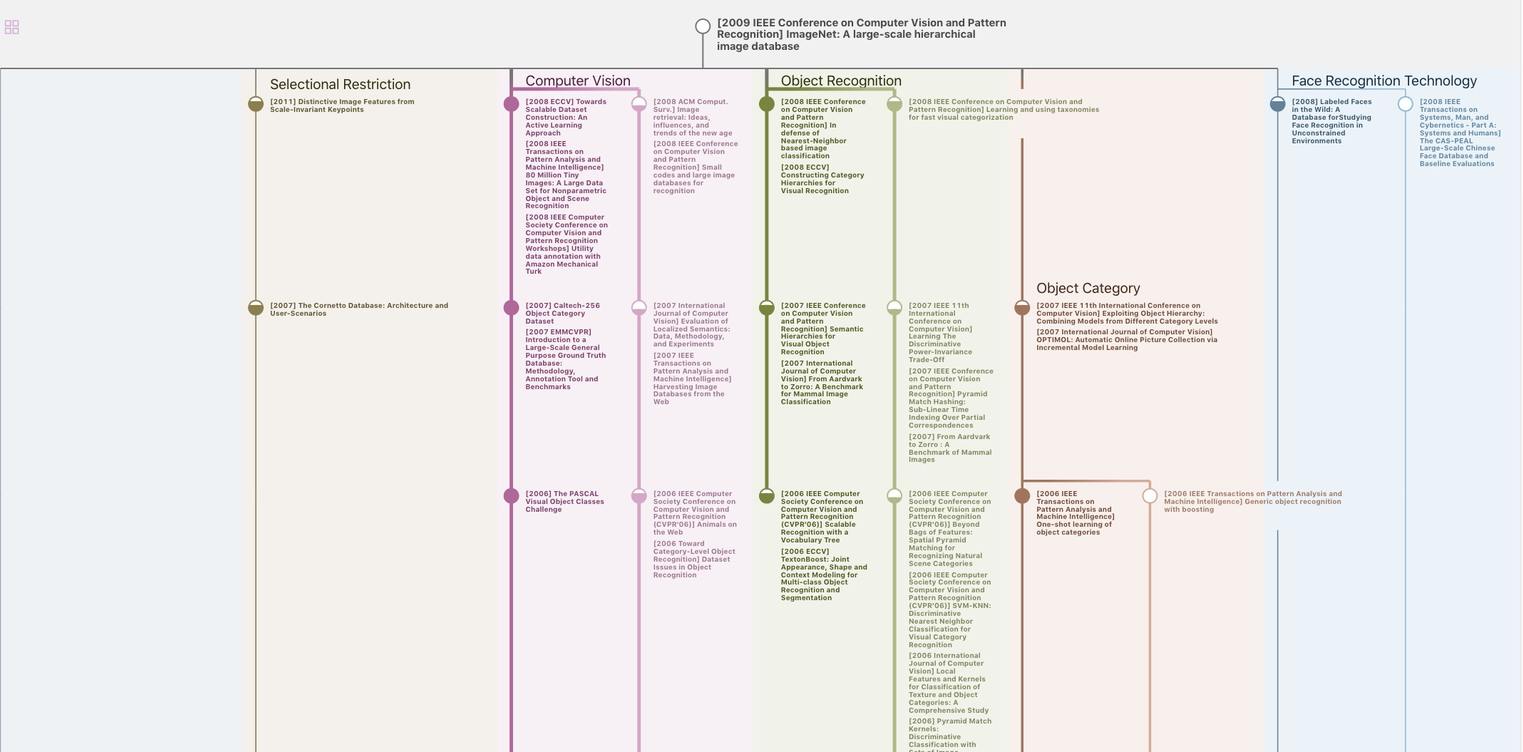
生成溯源树,研究论文发展脉络
Chat Paper
正在生成论文摘要