External validation of inpatient neonatal mortality prediction models in high-mortality settings
Research Square (Research Square)(2022)
摘要
Abstract Objectives: To evaluate the predictive accuracy of two neonatal prediction models in predicting all cause in-hospital mortality following patient admission. These models (The Neonatal Essential Treatment Score (NETS) which used treatments prescribed at admission, and the Score for Essential Neonatal Symptoms and Signs (SENSS) which used basic clinical signs), were derived in high-mortality, low-resource settings and are reflective of low-income countries’ clinical settings. Study design and settings: We used retrospectively collected routine clinical data recorded by duty clinicians at admission from 15 hospitals to externally validate and update the SENSS and NETS models to predict in-hospital mortality. Model performance was evaluated by assessing discrimination and calibration. Results: At initial external validation, the calibration intercept for NETS and SENSS was -1.109 (95% CI: -1.148 to -1.069) and -0.703 (95% CI: -0.738 to -0.669) respectively; The calibration slope for NETS and SENSS was 0.466 (95% CI: 0.451 to 0.480) and 0.565 (95% CI: 0.552 to 0.577) respectively. After model updating, SENSS and NETS calibration intercepts improved to 0.349 (95% CI: 0.321 to 0.377) and 0.032 (95% CI: -0.002 to 0.066) respectively; Calibration slopes improved to 1.029 (95% CI: 1.006 to 1.051) and 0.799 (95% CI: 0.774 to 0.823) for SENSS and NETS respectively. Final SENSS and NETS model discrimination was 0.834 (95% CI: 0.829 to 0.839) and 0.775 (95% CI: 0.768 to 0.782) respectively. Conclusion: It is possible to predict in-hospital mortality using either treatments or signs and symptoms derived from routine neonatal data from varied low-resource hospitals settings. The models’ performance after updating and external validation across different geographical regions supports their use for case-mix adjustment in similar routine hospital settings.
更多查看译文
关键词
neonatal,prediction,external validation,high-mortality
AI 理解论文
溯源树
样例
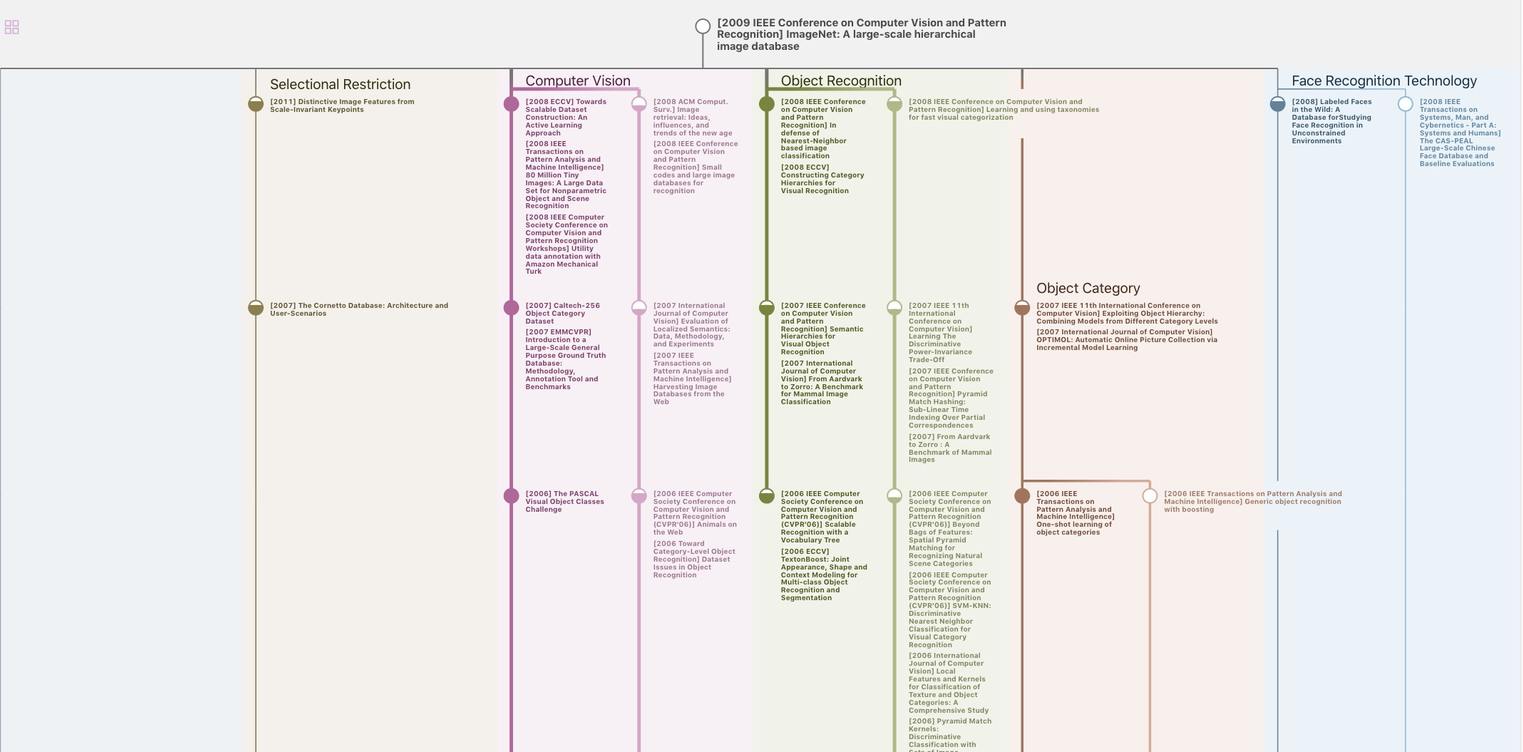
生成溯源树,研究论文发展脉络
Chat Paper
正在生成论文摘要