Performance Evaluation Analysis of Spark Streaming Backpressure for Data-Intensive Pipelines
crossref(2022)
摘要
In the past decades, a significant rise in the adoption of streaming applications has changed the decision-making process for the industry and academia sectors. This movement led to the emergence of a plurality of Big Data technologies such as Apache Storm, Spark, Heron, Samza, Flink, and other systems to provide in-memory processing for real-time Big Data analysis at high throughput. Spark Streaming represents one of the most popular open-source implementations which handles an ever-increasing data ingestion and processing by using the Unified Memory Manager to manage memory occupancy between storage and processing regions dynamically, which is the focus of this study. The problem behind memory management for data-intensive stream processing pipelines is that the incoming data is faster than the downstream operators can consume. Consequently, the backpressure of Spark acts in the opposite direction of downstream operators. In such a case, the incoming data overwhelms the memory manager and provokes memory leak issues. As a result, it affects the performance of applications generating, e.g., high latency, low throughput, or even data loss. In such a case, the initial intuition motivating our work is that memory management became the critical factor in keeping processing at scale and system stability of Spark. This work provides a deep dive into Spark backpressure, evaluates its structure, presents the main characteristics to support data-intensive streaming pipelines, and investigates the current in-memory-based performance issues.
更多查看译文
AI 理解论文
溯源树
样例
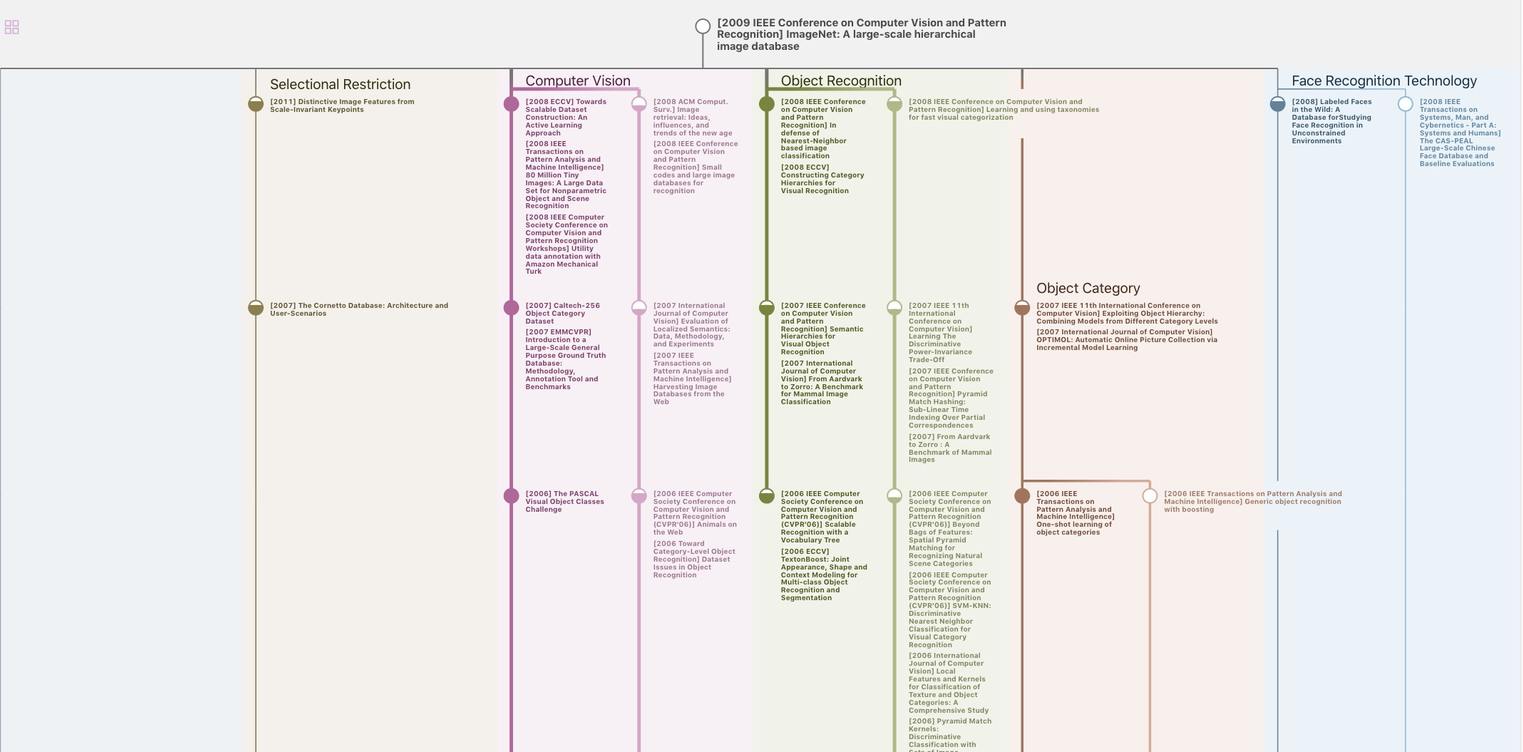
生成溯源树,研究论文发展脉络
Chat Paper
正在生成论文摘要