Identification of Distinct Clinical Phenotypes of Cardiogenic Shock Using Machine Learning Consensus Clustering Approach
crossref(2022)
摘要
Abstract Background: Cardiogenic shock (CS) is a heterogeneous condition with multiple underlying causes and associated outcomes. Discriminating between different patterns of CS is still challenging. We tested the hypothesis that the machine learning (ML) consensus clustering method would identify CS phenotypes with unique clinical profiles and outcomes.Methods: Patients diagnosed with CS on admission from the electronic ICU (eICU) Collaborative Research Database were included in the current study. We performed an unsupervised ML consensus clustering analysis among 21,925 patients with CS. The optimal number of clusters was determined by examining the consensus matrix (CM) heat map, cumulative distribution function (CDF), cluster-consensus plots, and the proportion of ambiguously clustered pairs (PAC) analysis. We calculated the standardized mean difference (SMD) of each variable and used the cutoff of ±0.3 to identify each cluster’s key features. We assessed the association of the phenotypes with multiple clinical endpoints using logistic regression (LR) analysis.Results: The consensus cluster analysis identified two clusters (Cluster 1: n=9,848; Cluster 2: n=12,077). The key features of patients in Cluster 1, compared with Cluster 2, included: lower blood pressure, lower eGFR (estimated glomerular filtration rate), higher BUN (blood urea nitrogen), higher creatinine, lower albumin, higher potassium, lower bicarbonate, lower red blood cell (RBC), higher red blood cell distribution width (RDW), higher SOFA score, higher APS III score, and higher APACHE IV score on admission. The results of LR analysis showed that the Cluster 2 was associated with lower in-hospital mortality (odds ratio [OR]: 0.374; 95% confidence interval [CI]: 0.347-0.402; P < 0.001), ICU mortality (OR: 0.349; 95% CI: 0.318-0.382; P < 0.001), and the incidence of acute kidney injury (AKI) after admission (OR: 0.478; 95% CI: 0.452-0.505; P < 0.001).Conclusions: ML consensus clustering analysis synthesized the pattern of clinical and laboratory data to reveal distinct CS phenotypes with different clinical outcomes.
更多查看译文
AI 理解论文
溯源树
样例
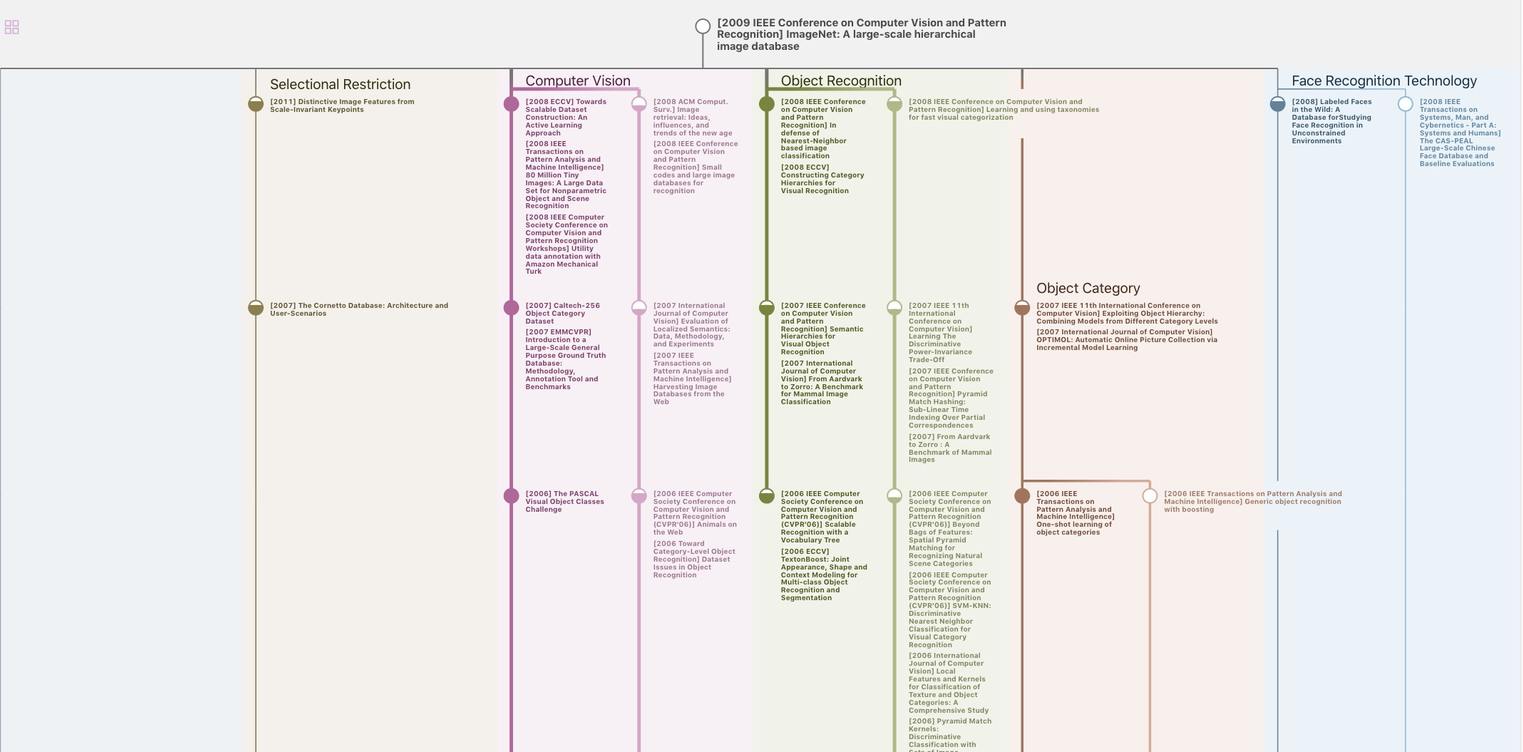
生成溯源树,研究论文发展脉络
Chat Paper
正在生成论文摘要