Shannon entropy is a more comprehensive and principled morphological productivity measure than the standard alternatives
crossref(2022)
摘要
Existing corpus-based measures of morphological productivity all exhibit problematic dependences on sample size. Here, we show that another measure, the Shannon entropy of a type frequency distribution, has a different relationship with sample size, one that allows meaningful analysis in a wider range of circumstances. Once the sample gets large enough, entropy stabilises at interpretable values. In contrast to the existing measures, this behaviour allows the entropy scores of samples of different sizes to be sensibly compared. Entropy's stabilisation is due to an intriguing property of type frequency distributions, namely their self-similarity: even when sample size changes, the shape of the distribution itself does not. We also include empirical comparisons of entropy to three standard productivity measures—type count, potential productivity, and S—and provide a tentative conceptual validation of entropy as a productivity measure, showing with a Bayesian regression model that entropy picks up on important aspects of what it means for a morpheme to be productive.
更多查看译文
AI 理解论文
溯源树
样例
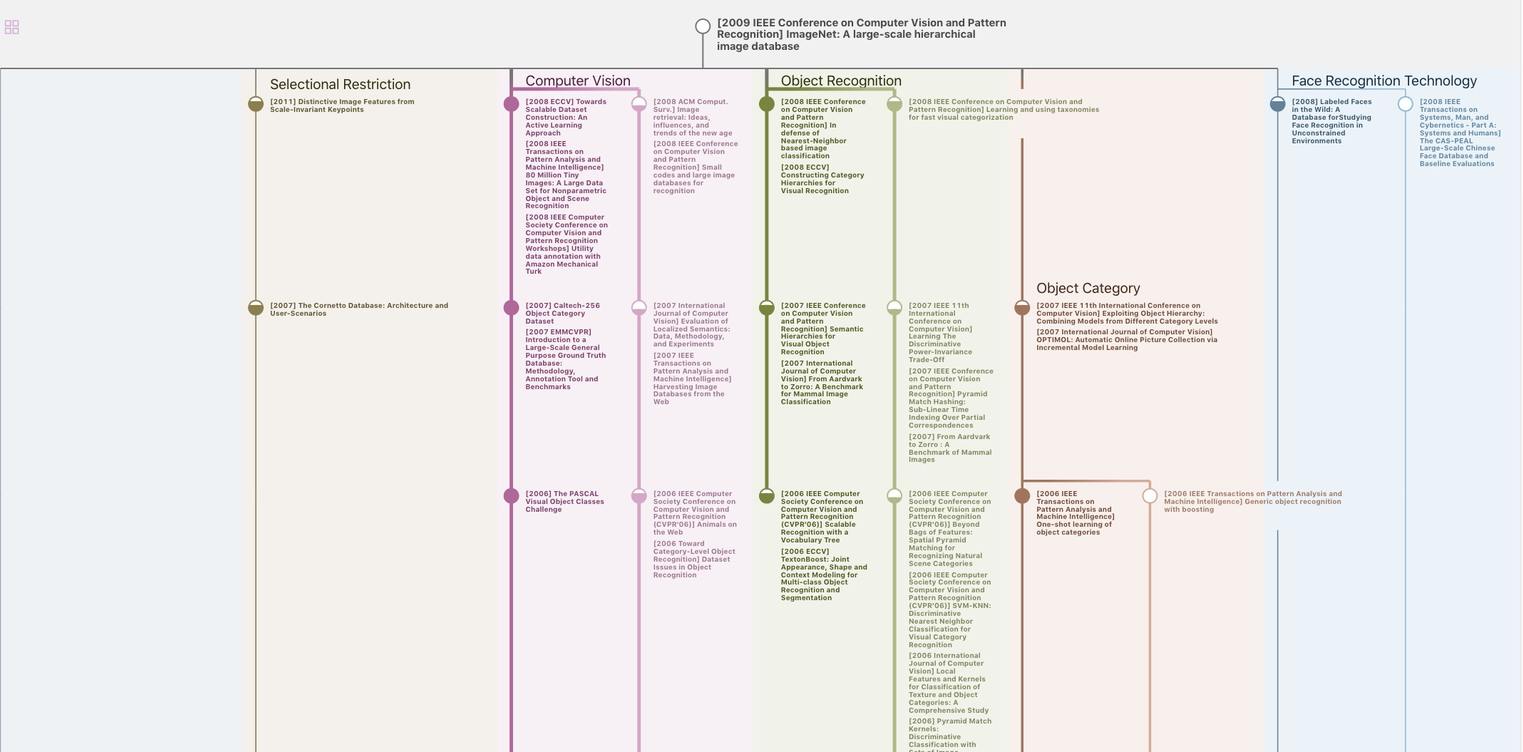
生成溯源树,研究论文发展脉络
Chat Paper
正在生成论文摘要