Identifying distinct subgroups of suicidal ideations: A pre-registered ecological momentary assessment study in psychiatric patients
crossref(2022)
摘要
**Background.** Suicidal ideations (SI) are amongst the strongest predictors for suicide attempts, yet reliable prediction models for suicide risk are still lacking. One explanation for this is that endorsement and expression of SI are not uniform and may vary when indexed over time. Using intensive longitudinal assessments of SI with ecological momentary assessments (EMA), prior research identified five distinct subgroups that differed in mean SI severity and variability over time. **Methods.** We first aimed to replicate these results using latent profile analysis (LPA) on EMA data (5 assessments/day over 28 days: 34% response rate of all beeps completed) of 50 psychiatric patients (32 females, 64%, age *M*=35.26, *SD*=12.54). We then used longitudinal clustering (LC), an advanced statistical approach that considers the longitudinal, dynamic nature of EMA, to replicate the subgroups. **Results.** Using LPA, we replicated four of the five groups with somewhat different characterizations compared to prior findings. Using LC, we identified subgroups representing the nature of the observed SI better and showed more anticipated clinical characteristics. Among those, hopelessness and prior SI were most convincing, i.e., the subgroup with the least severe SI subgroup were characterized by least hopelessness and the most severe SI subgroup by more hopelessness. **Conclusion.** Applying LC to EMA collected from patients with SI allows to identify valid and reliable SI subgroups with more distinct clinical characteristics, an important step towards a better understanding of SI and basis for improving prediction and prevention.
更多查看译文
AI 理解论文
溯源树
样例
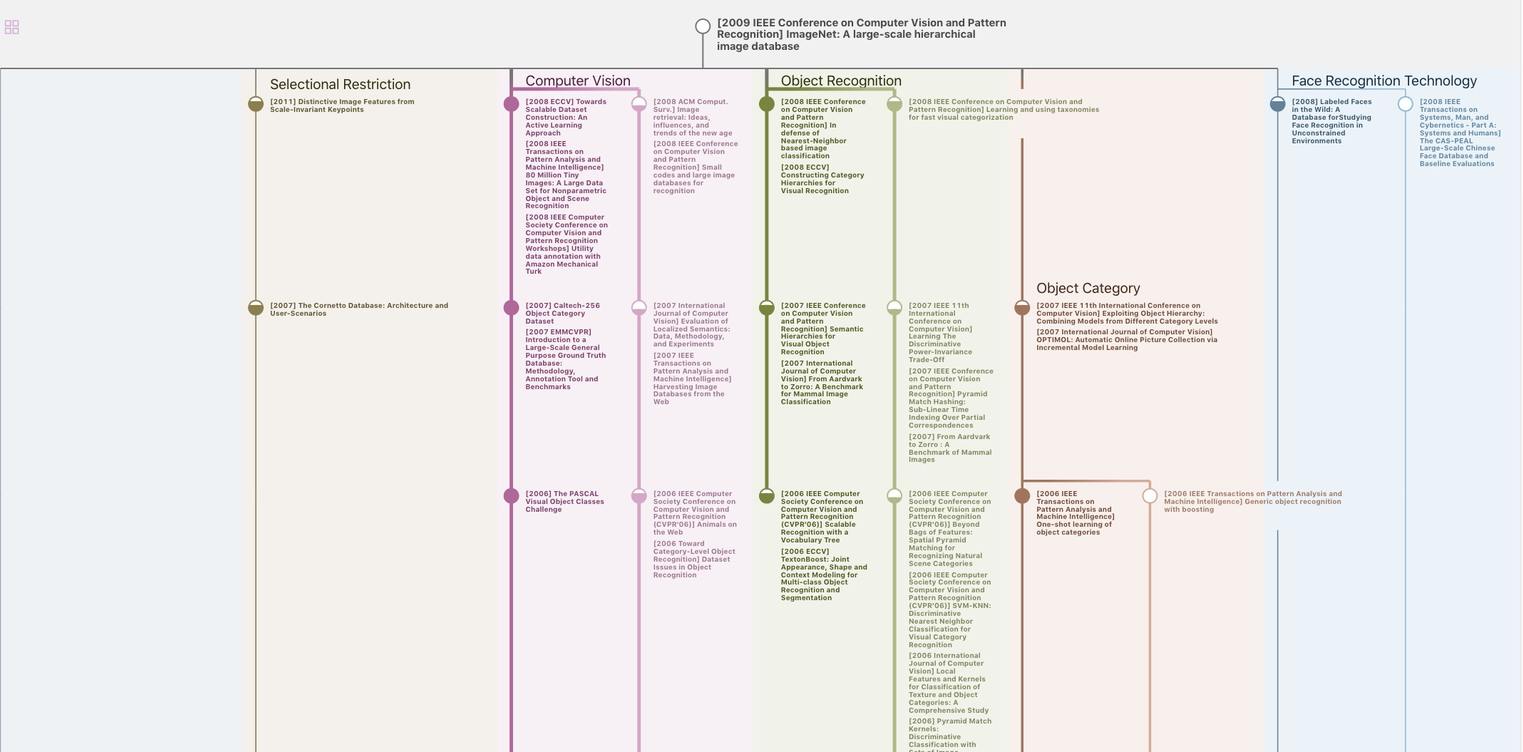
生成溯源树,研究论文发展脉络
Chat Paper
正在生成论文摘要