ML-based water quality modeling at national level in a data-scarce region
AGILE: GIScience Series(2022)
摘要
Abstract. Water quality (WQ) modeling can be used for gaining insight into WQ issues in order to implement effective mitigation efforts. Process-based nutrient models are very complex, requiring a lot of input parameters and computationally expensive calibration. Recently, ML approaches have shown to achieve an accuracy comparable to the process-based models and even outperform them when describing nonlinear relationships. We used observations from 242 Estonian catchments, amounting to 469 yearly total nitrogen (TN) and 470 total phosphorus (TP) measurements covering the period 2016–2020 to train random forest (RF) models for predicting annual N and P concentrations. We used a total of 82 predictor variables, including land use and land cover (LULC), soil, climate and topography parameters and applied a feature selection strategy to reduce the number of dependent features in the models. The SHAP method was used for deriving the most relevant predictors. The performance of our models is comparable to previous process-based models used in the Baltic region. However, as input data used in our models is easier to obtain, the models offer superior applicability in areas, where data availability is insufficient for process-based approaches.
更多查看译文
关键词
water quality modeling,water quality,ml-based,data-scarce
AI 理解论文
溯源树
样例
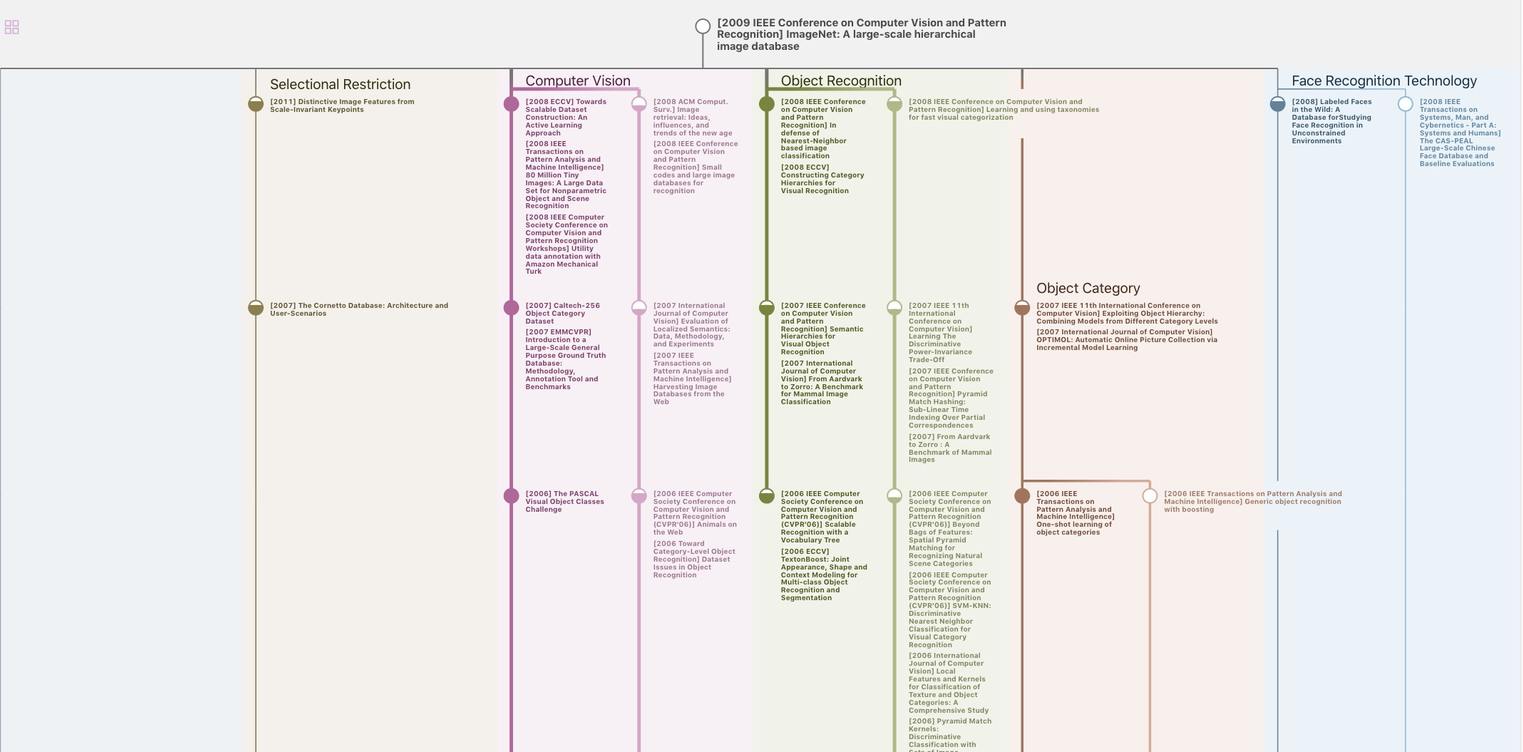
生成溯源树,研究论文发展脉络
Chat Paper
正在生成论文摘要