Knowledge Distillation for Translating Erroneous Speech Transcriptions
Journal of Natural Language Processing(2022)
摘要
Recent studies consider knowledge distillation as a promising method for speech translation (ST) using end-to-end models. However, its usefulness in cascade ST with automatic speech recognition (ASR) and machine translation (MT) models has not yet been clarified. An ASR output typically contains speech recognition errors. An MT model trained only on human transcripts performs poorly on error-containing ASR results. Thus, it should be trained considering the presence of ASR errors during inference. In this paper, we propose using knowledge distillation for training of the MT model for cascade ST to achieve robustness against ASR errors. We distilled knowledge from a teacher model based on human transcripts to a student model based on erroneous transcriptions. Our experimental results showed that the proposed method improves the translation performance on erroneous transcriptions. Further investigation by combining knowledge distillation and fine-tuning consistently improved the performance on two different datasets: MuST-C English--Italian and Fisher Spanish--English.
更多查看译文
关键词
knowledge,speech
AI 理解论文
溯源树
样例
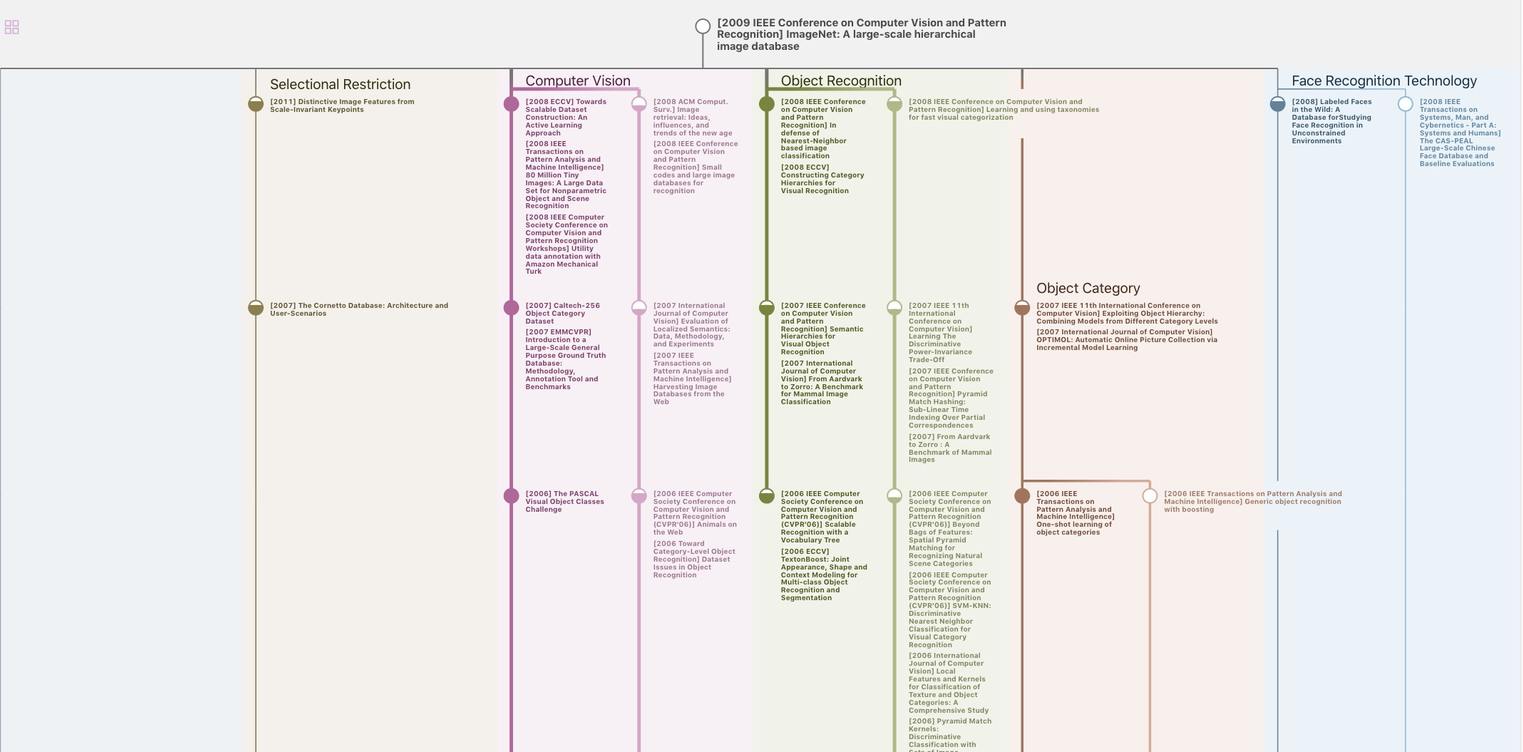
生成溯源树,研究论文发展脉络
Chat Paper
正在生成论文摘要