Abstract 2723: Model-based cancer therapy selection by linking tumor vulnerabilities to drug mechanism
Cancer Research(2022)
摘要
Abstract Selection of the treatments most likely to combat a patient’s tumor is a central aim of precision oncology. We are currently developing a functional precision oncology program at the University of Utah and Huntsman Cancer Institute that combines the multi-omic characterization of a patient's tumor with the functional screening of relevant drug candidates in patient-derived organoid tumor models to identify which drugs are uniquely capable of combating the patient’s cancer. Here we present our model-based approach for utilizing the multi-omic tumor data to computationally predict the patient’s response to each drug. In this approach, we first identify the genomic and transcriptomic vulnerabilities of the tumor as genes harboring somatic DNA mutations or copy number changes (from paired tumor/normal WGS/WES DNA sequencing data), as well as genes whose expression levels have been significantly altered in the tumor (based on bulk or single-cell RNA sequencing data). Second, using targeting information from drug-gene interaction databases (DGIdb) we compile a list of genes targeted by each drug relevant in the patient’s treatment. Third, we utilize gene interaction database knowledge (KEGG) to construct a gene interaction graph to link each drug’s gene targets with all of the tumor’s vulnerability genes. We then identify all gene interaction paths connecting specific drug target genes with specific vulnerability genes; and score each path and combine all path-specific scores for the target-vulnerability gene pair. Subsequently, we further combine all pairwise scores across all drug target genes and all tumor vulnerability genes and determine the statistical significance of the resulting score by sampling the background distribution of such scores across random drugs, target genes, and vulnerability genes. We have applied our algorithm to predict drug response in advanced breast cancer patients as well as using publicly available tumor cell line data (GDSC2). We have found a high level of concordance between our computational prediction and organoid/cell line screening results, clearly separating sensitive and non-sensitive models. Using Bayesian probability, we compare the drug-specific score distributions of sensitive and non-sensitive models and are able to identify sensitive cell lines/patients with high accuracy. By encapsulating available information on direct gene-gene interactions, the drug’s direct gene targets and the collected omic vulnerabilities of the tumor, our model is not only capable of predicting sensitivity to both targeted and chemotherapy agents, but can also provide a mechanistic understanding for the targeting of the tumor. Our approach will be validated and fine-tuned on a large cohort of breast and brain cancer patients as well as patient PDX models, interrogating gene-target interactions to identify novel relevant target genes/pathways. Citation Format: Szabolcs Tarapcsak, Yi Qiao, Xiaomeng Huang, Tony Di Sera, Matthew H. Bailey, Bryan E. Welm, Alana L. Welm, Gabor T. Marth. Model-based cancer therapy selection by linking tumor vulnerabilities to drug mechanism [abstract]. In: Proceedings of the American Association for Cancer Research Annual Meeting 2022; 2022 Apr 8-13. Philadelphia (PA): AACR; Cancer Res 2022;82(12_Suppl):Abstract nr 2723.
更多查看译文
关键词
cancer therapy selection,tumor vulnerabilities,cancer therapy,drug mechanism,model-based
AI 理解论文
溯源树
样例
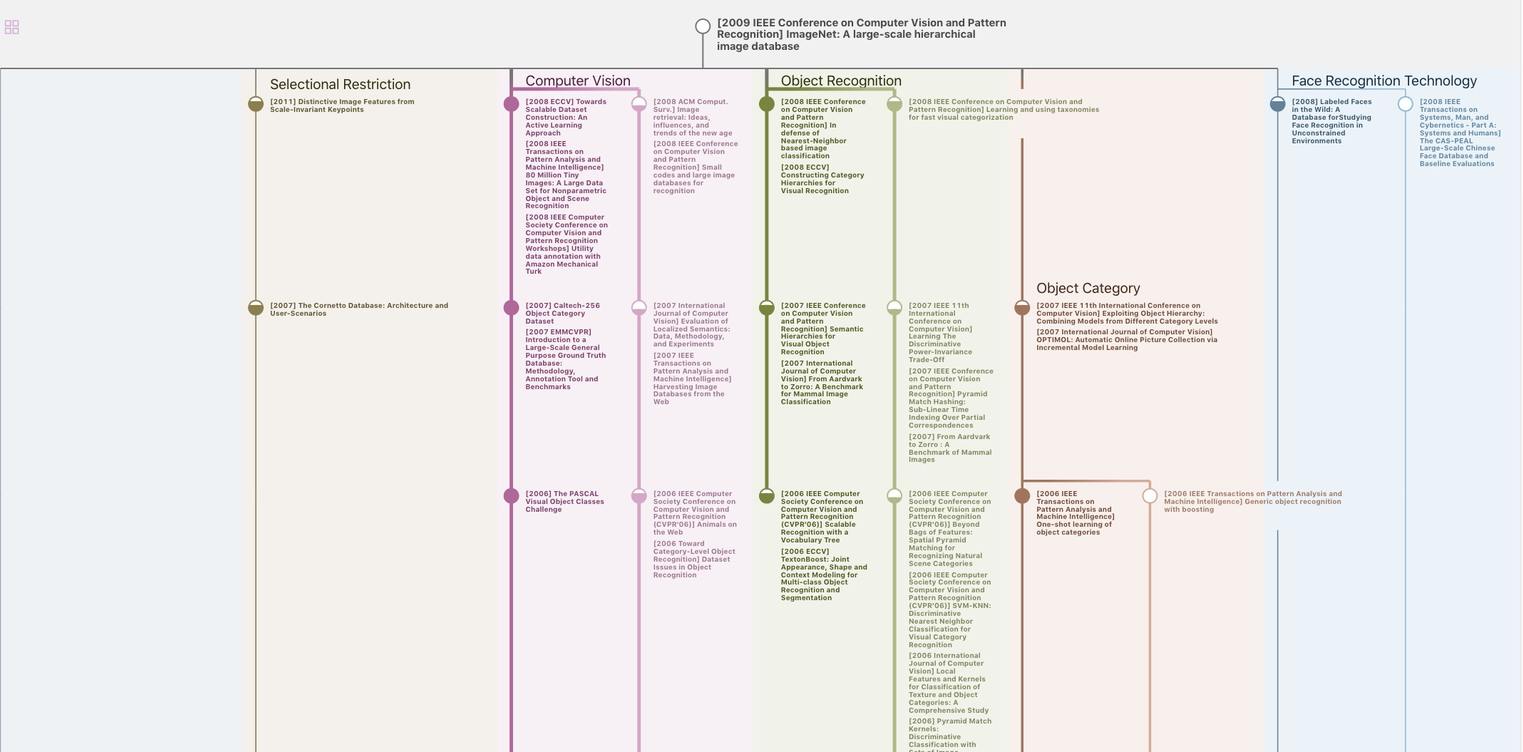
生成溯源树,研究论文发展脉络
Chat Paper
正在生成论文摘要