Abstract 1227: Molecular-based tumor grade predictor for breast cancer, clear cell renal cell carcinoma, and lung adenocarcinoma
Cancer Research(2022)
摘要
Abstract Classifying tumors based on histological grade is used for many cancers to determine a patient’s prognosis and select the appropriate treatment. Although low grade (G1) and high grade (G3/G4) tumors have been shown to predict patient survival in many cancers, an intermediate histological grade (G2) does not have clear prognostic significance. Therefore, to allow a more accurate assessment of a patient’s risk, recent studies have focused on utilizing gene expression data to improve prognostic stratification of tumors into either a high-risk or low-risk group. Here, we present a molecular-based classifier that can use gene expression data from both RNA sequencing (RNA-seq) and microarray profiling to achieve accurate prediction of tumor grade for breast cancer (BRCA), lung adenocarcinoma (LUAD), and clear cell renal cell carcinoma (ccRCC). The tumor grade predictor was developed by training a machine-learning algorithm to classify tumors into categories of low (mG1) and high (mG3/mG4) molecular grades for each cancer type using rank transformation of gene expression data from multiple datasets. This resulted in the revealing of features of the mG3/mG4 groups not shown by histological methods (i.e., the Warburg effect, proliferation signaling pathways, inactivation of tumor suppressors, fibrotic and immunosuppressive microenvironment, and the loss of normal cell morphology), allowing for a more accurate stratification between the high- and low-grade groups. Importantly, by using rank transformation to analyze data from both RNA-seq and microarray profiling, the predictor functioned independent of various sources of batch effect, resulting in the ability to work with single samples and not only datasets. Validation of the tumor grade classifier with pathological classification of G1 and G3/G4 samples from RNA-seq and microarray expression data into mG1 and mG3/mG4 groups showed a more accurate performance than other gene expression-based methods, which was demonstrated by it’s sensitivity-specificity value (AUC scores: BRCA - 0.95, LUAD - 0.89, ccRCC - 0.83). Further, cox-regression analysis showed that prognostic value of mG1 and mG3 groups were comparable to histological grades (BRCA - 0.71 vs. 0.71; LUAD - 0.66 vs. 0.62; ccRCC - 0.74 vs. 0.71), demonstrating the ability of the classifiers to successfully split G2 histological samples into molecular grades. In conclusion, we developed a tumor grade predictor that utilizes expression data from multiple datasets to accurately classify tumors for three cancer types. The resulting prognostic ability of the molecular grades demonstrates their capability of assessing the levels of risk for G2 samples, suggesting the potential for molecular-based tumor grade classifiers to be used in future clinical application. Citation Format: Zoya Antysheva, Maria V. Guryleva, Ivan Valiev, Nikita Kotlov, Viktor Svekolkin, Olga Gancharova, Vladimir Kushnarev, Yuriy Popov, Anna Belozerova, Dmitry Tabakov, Jessica H. Brown, Krystle Nomie, Alexander Bagaev, Nathan Fowler. Molecular-based tumor grade predictor for breast cancer, clear cell renal cell carcinoma, and lung adenocarcinoma [abstract]. In: Proceedings of the American Association for Cancer Research Annual Meeting 2022; 2022 Apr 8-13. Philadelphia (PA): AACR; Cancer Res 2022;82(12_Suppl):Abstract nr 1227.
更多查看译文
关键词
tumor grade predictor,renal cell carcinoma,lung adenocarcinoma,breast cancer,molecular-based
AI 理解论文
溯源树
样例
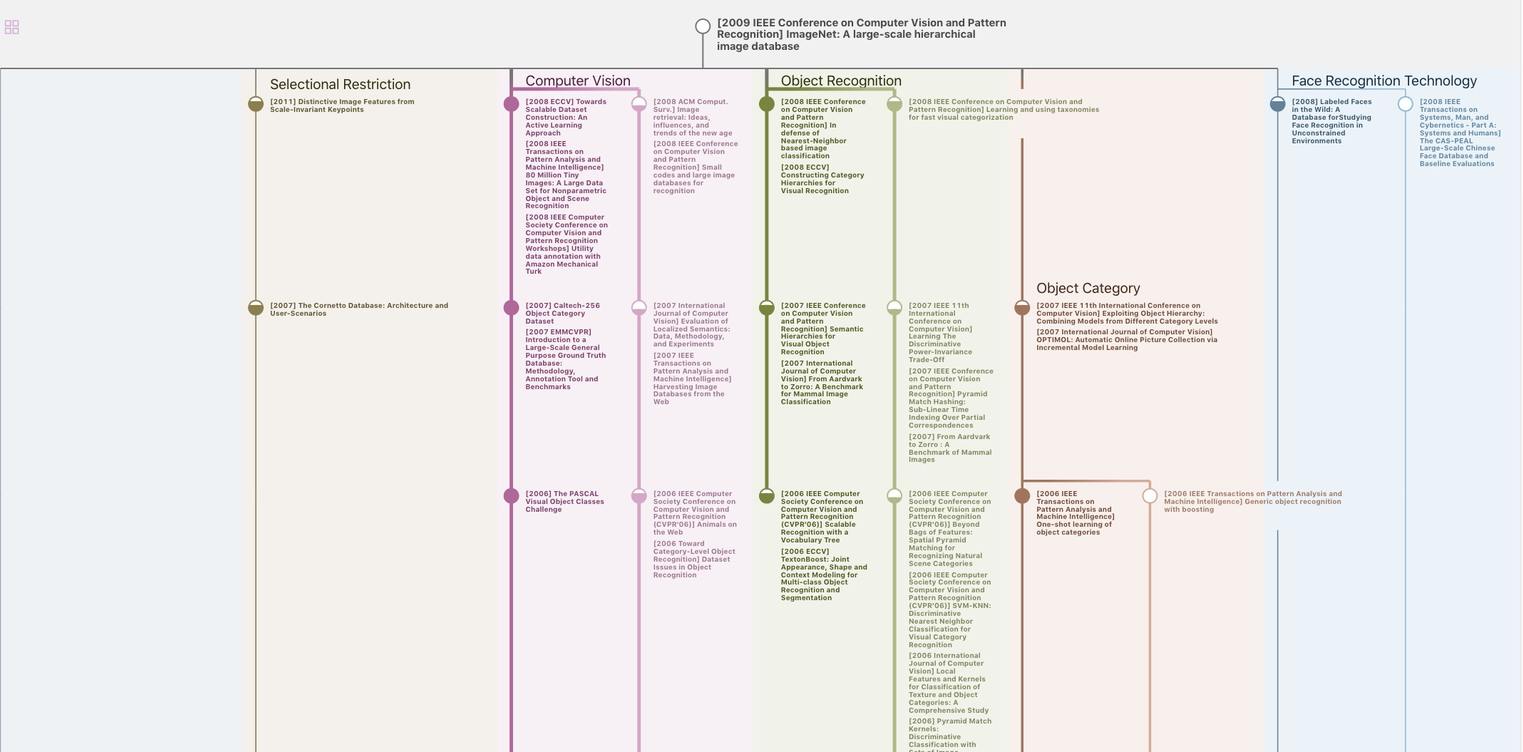
生成溯源树,研究论文发展脉络
Chat Paper
正在生成论文摘要