Detecting High-Risk Anomalies in Aircraft Dynamics Through Entropic Analysis of Time Series Data
AIAA AVIATION 2022 Forum(2022)
摘要
Despite recent efforts to move away from traditional threshold exceedance detection methods for aircraft state monitoring, modern aircraft still rely on safety thresholds to communicate to pilots the identification of an anomaly in the aircraft when a threshold is surpassed. Current anomaly detection methods mainly depend on uninterpretable machine learning models to learn complex patterns and relationships contained in the time series data of aircraft. Although these methods are capable of identifying known anomalies, their deficiency in interpretability presents a challenge when translating them to different aircraft. To overcome this deficiency, entropic analysis of aircraft dynamics seeks to characterize the complexity, or lack thereof, of the aircraft dynamics prior to the development of a risk scenario. This complexity characterization provides a more straightforward summary of state changes in the dynamics of flight variables. To build a foundation for entropic analysis, we analyzed the complexity of unstable approaches, an anomalous event present in many of today's aviation accidents. The analysis revealed a statistically significant difference in the complexity distribution of flight variables under a stable approach versus an unstable approach. These differences in complexity were especially notable minutes before an approach was identified as unstable. Moreover, the multiscale entropic analysis revealed the presence of signal complexity at multiple time scales across multiple time windows before landing. By capturing state changes and corrections in the aircraft dynamics using entropy, advanced, yet still interpretable, sensor systems based on entropic frameworks from this study can be constructed in the future using classical machine learning approaches.
更多查看译文
关键词
aircraft dynamics,entropic analysis,anomalies,series data,high-risk
AI 理解论文
溯源树
样例
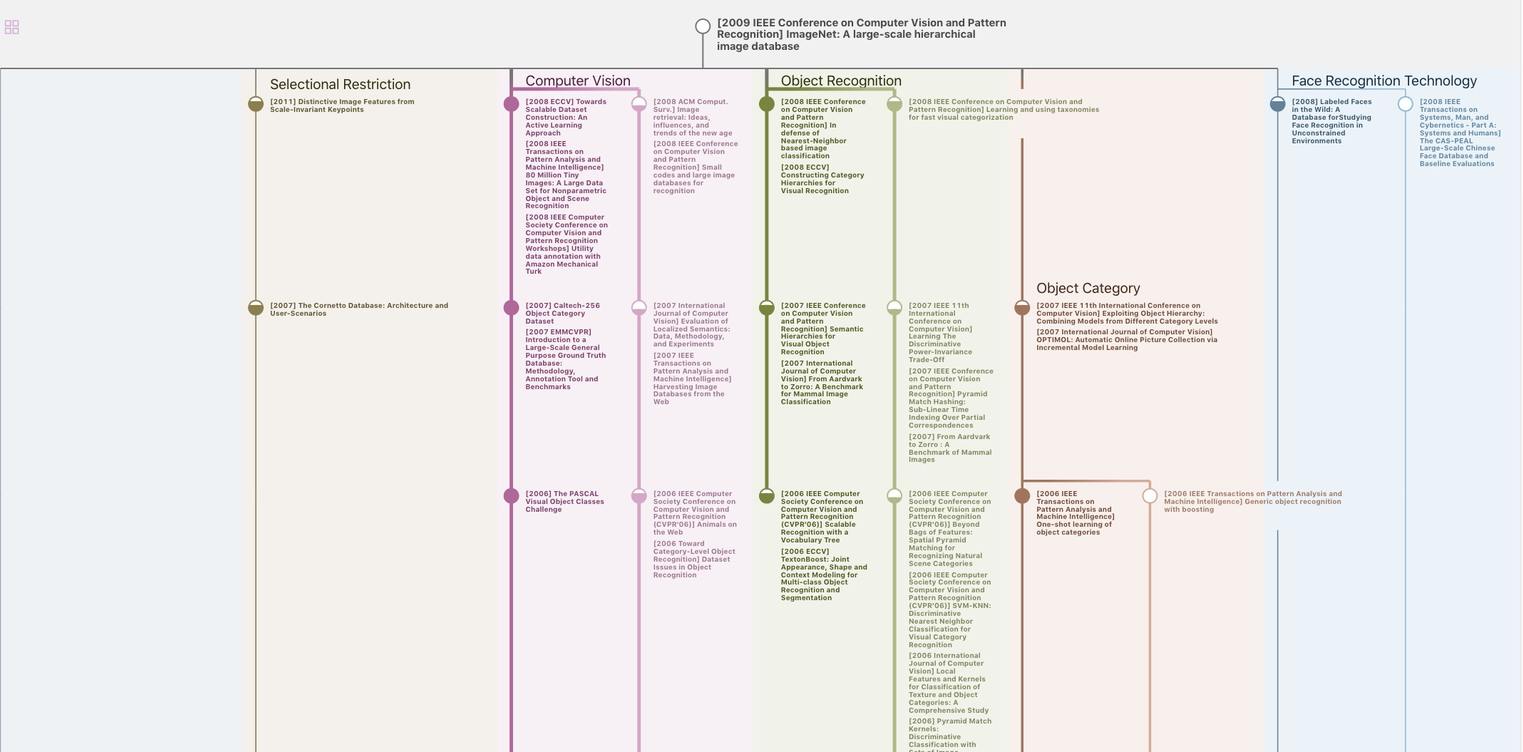
生成溯源树,研究论文发展脉络
Chat Paper
正在生成论文摘要