Rethinking Regularization with Random Label Smoothing
Neural Processing Letters(2024)
摘要
Regularization helps to improve machine learning techniques by penalizing the models during training. Such approaches act in either the input, internal, or output layers. Regarding the latter, label smoothing is widely used to introduce noise in the label vector, making learning more challenging. This work proposes a new label regularization method, Random Label Smoothing, that attributes random values to the labels while preserving their semantics during training. The idea is to change the entire label into fixed arbitrary values. Results show improvements in image classification and super-resolution tasks, outperforming state-of-the-art techniques for such purposes.
更多查看译文
关键词
Convolutional neural networks,Regularization,Label smoothing
AI 理解论文
溯源树
样例
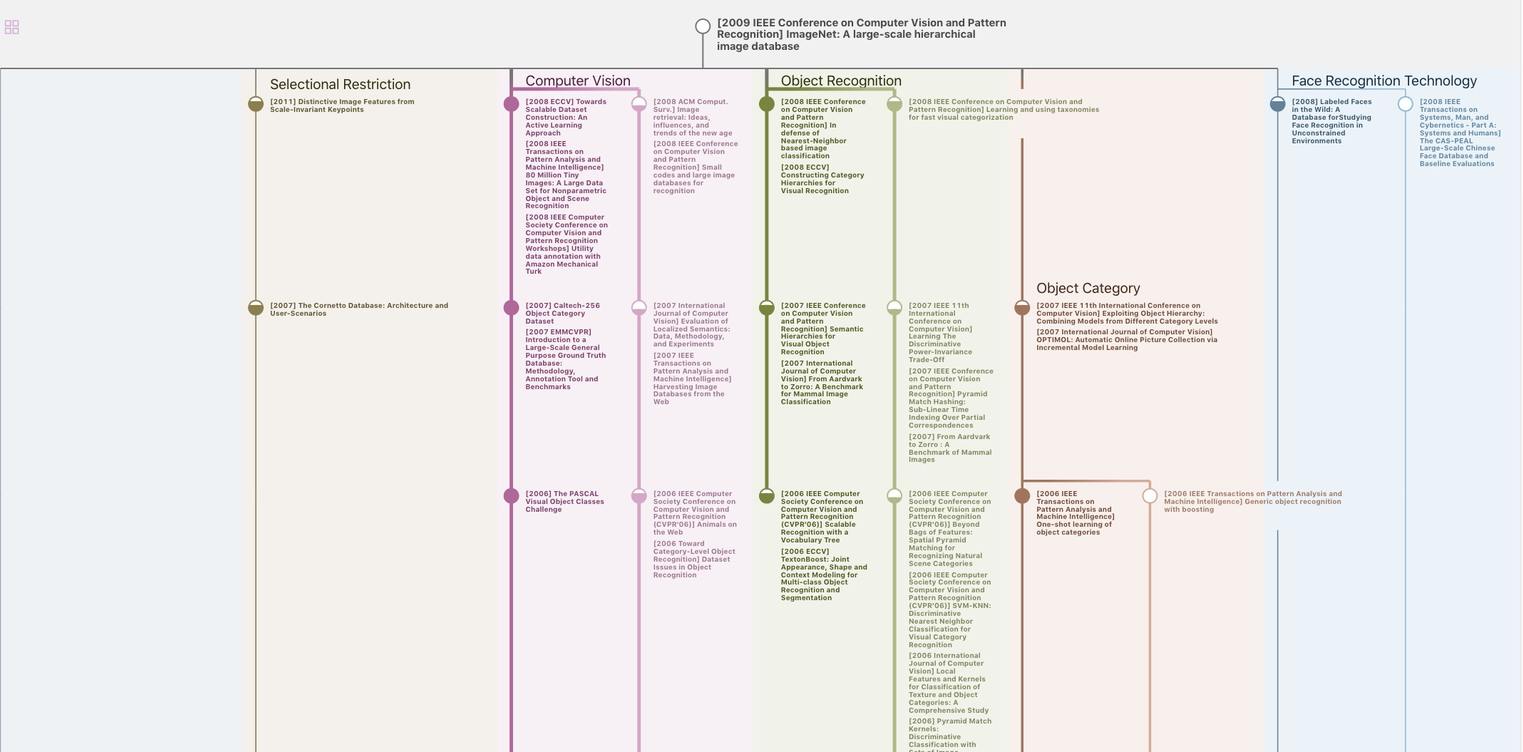
生成溯源树,研究论文发展脉络
Chat Paper
正在生成论文摘要