A deep learning approach based defect visualization in pulsed thermography
IAES International Journal of Artificial Intelligence(2022)
摘要
Non-destructive evaluation (NDE) is very essential in measuring the properties of materials and in turn detect flaws and irregularities. Pulsed thermography (PT) is one of the advanced NDE technique which is used for detecting and characterizing subsurface defects. Recently many methods have been reported to enhance the signal and defect visibility in PT. In this paper, a novel unsupervised deep learning-based auto-encoder (AE) approach is proposed for enhancing the signal-to-noise ratio (SNR) and visualize the defects clearly. A detailed theoretical background of AE and its application to PT is discussed. The SNR and defect detectability results are compared with the existing approaches namely, higher order statistics (HOS), principal component thermography (PCT) and partial least square regression (PLSR) thermography. Experimental results show that AE approach provides better SNR at the cost of defect detectability.
更多查看译文
关键词
defect visualization,deep learning approach,deep learning
AI 理解论文
溯源树
样例
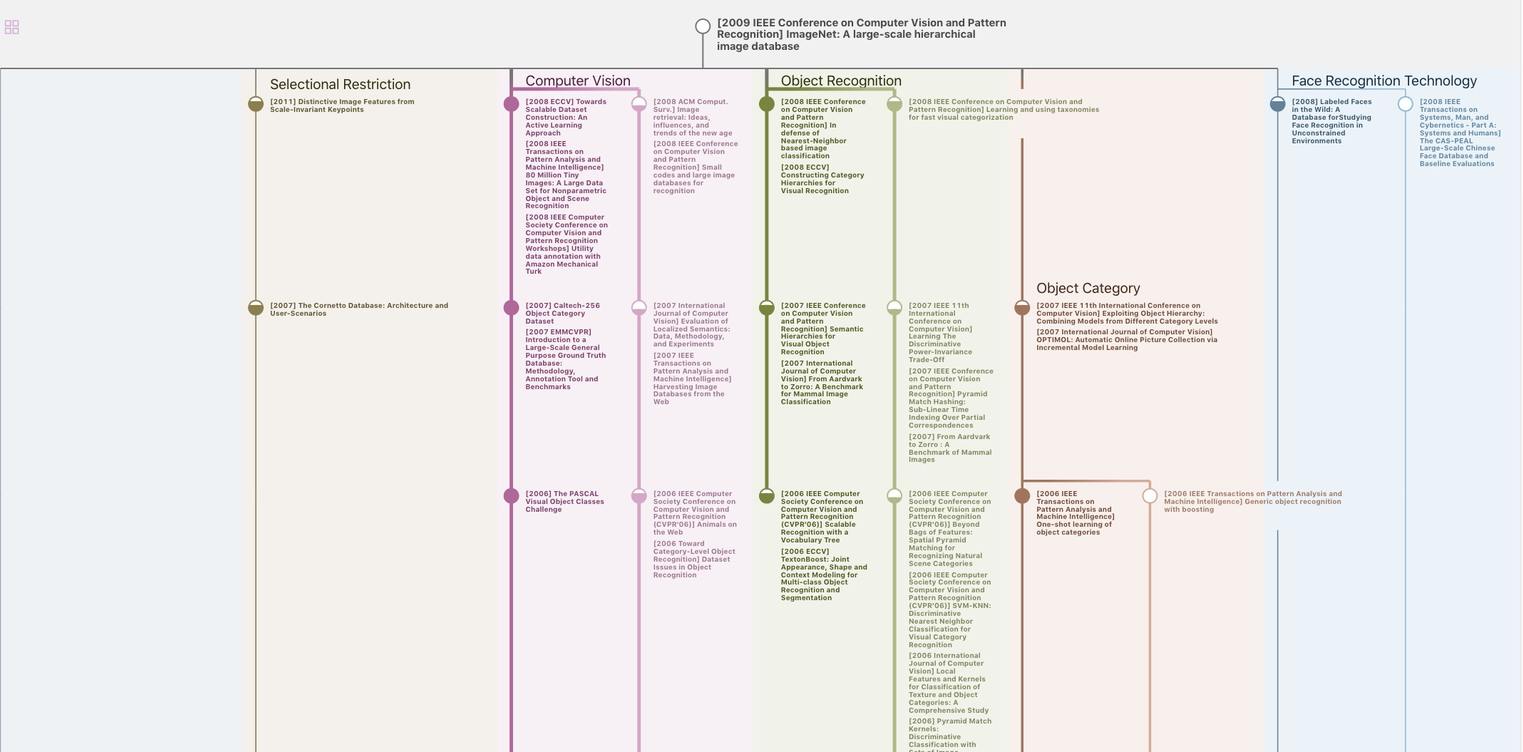
生成溯源树,研究论文发展脉络
Chat Paper
正在生成论文摘要