HybridMSSN: A Deep Learning Hyperspectral Image Classification Model
Research Square (Research Square)(2022)
摘要
Abstract With the goal of obtaining accuracy and optimum computation, Hyperspectral image (HI) classification models have been developed for best use in a variety of applications. Because HI contains a lot of spatial and spectral information, classification using merely a Convolutional Neural Network (CNN) isn't always enough. This paper solves these concerns by proposing a new Hybrid Multi-Scale Spinal Net (HybridMSSN). The HI is subjected to Principal Component Analysis (PCA) in order to isolate the most useful spectral bands and eliminate the superfluous ones. For feature learning and classification, the model uses multiscale CNNs and a Spinal Fully Connected Network (SFCN). Three 3D-CNNs are used to extract spectral and spatial features from the 3-dimensional HI, followed by a 2D-CNN for spatial feature learning. The model uses SFCNs to optimize computation, reducing the number of multiplications in the activation function of neural networks and resulting in a faster response. The multi-scale features acquired from the different 3D Convolution layers are flattened and transferred to independent SFCNs after Max Pooling, Dropout, and Batch Normalization. Similarly, features collected from the 2D convolution layer and Dropout are flattened and supplied to the SFCN. For categorization, the SFCNs' outputs are combined. Despite limited training samples, the existence of noise, and an imbalance of class concerns, investigations conducted on three standard datasets revealed significant accuracy gains when compared to four state-of-the-art models.
更多查看译文
关键词
classification,learning
AI 理解论文
溯源树
样例
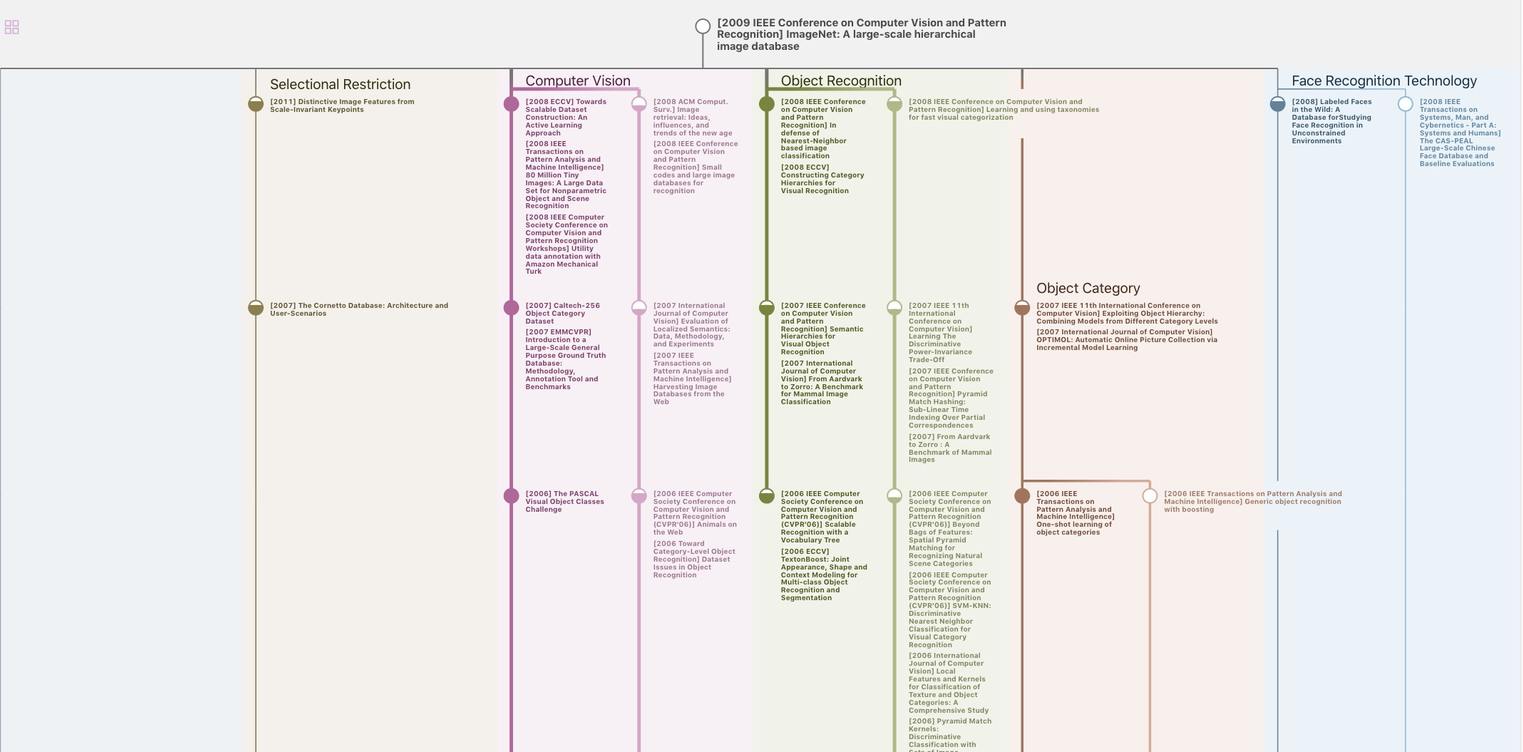
生成溯源树,研究论文发展脉络
Chat Paper
正在生成论文摘要