Epitopological Sparse Deep Learning via Network Link Prediction: A Brain-Inspired Training for Artificial Neural Networks
crossref(2022)
摘要
Sparse training (ST) aims to improve deep learning by replacing fully connected artificial neural networks (ANNs) with sparse ones. ST is promising but at an early stage, therefore it might benefit to borrow brain-inspired learning paradigms such as epitopological learning (EL) from complex network intelligence theory. EL is a field of network science that studies how to implement learning on networks by changing the shape of their connectivity structure (epitopological plasticity). EL was conceived together with the Cannistraci-Hebb (CH) learning theory according to which: the sparse local-community organization of many complex networks (such as the brain ones) is coupled to a dynamic local Hebbian learning process and contains already in its mere structure enough information to partially predict how the connectivity will evolve during learning. One way to implement EL is via link prediction: predicting the existence likelihood of each nonobserved link in a network. CH theory inspired a network automata rule for link prediction called CH3-L3 that was recently proven to be very effective for general purpose link prediction. Here, starting from CH3-L3 we propose a CH training (CHT) approach to implement epitopological sparse deep learning in ANNs. CHT consists of three parts: kick start pruning, to hint the link predictors; epitopological prediction, to shape the ANN topology; and weight refinement, to tune the synaptic weights values. Experiments on MNIST and CIFAR10 datasets compare the efficiency of CHT and other ST-based algorithms in speeding up the ANN training across epochs. While SET leverages random evolution and RigL adopts gradient information, CHT is the first algorithm in ST that learns to shape sparsity by using the sparse topological organization of the ANN.
更多查看译文
AI 理解论文
溯源树
样例
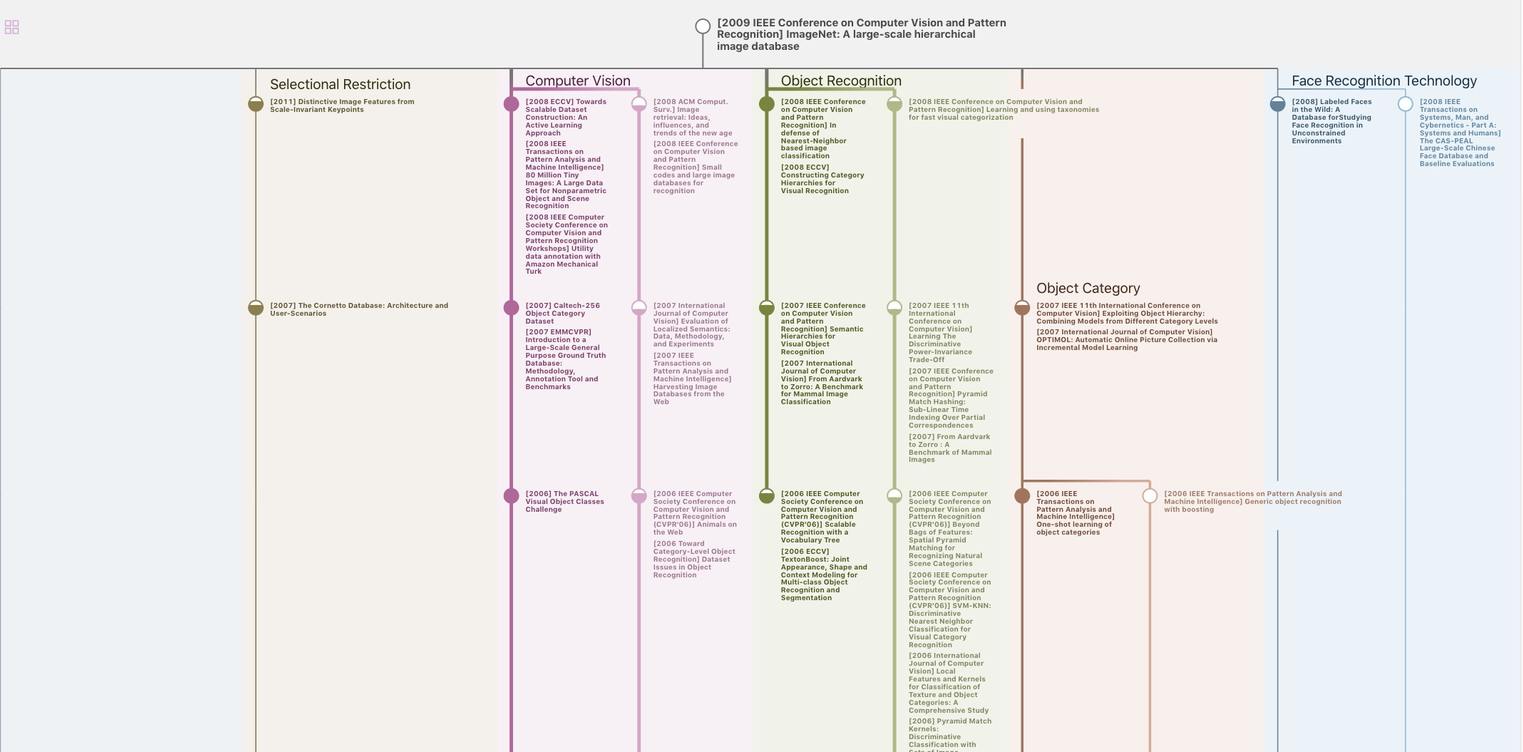
生成溯源树,研究论文发展脉络
Chat Paper
正在生成论文摘要