Cross-Modal Recurrent Semantic Comprehension for Referring Image Segmentation
IEEE Transactions on Circuits and Systems for Video Technology(2023)
摘要
Referring image segmentation aims to segment the target object from the image according to the description of language expression. Due to the diversity of language expressions, word sequences in different orders often express different semantic information. The previous methods focus more on matching different words to different visual regions in the image separately, ignoring the global semantic understanding of language expression based on the sequence structure. To address this problem, we redesign a new recurrent network structure for referring image segmentation, called Cross-Modal Recurrent Semantic Comprehension Network (CRSCNet), to obtain a more comprehensive global semantic understanding through iterative cross-modal semantic reasoning. Specifically, in each iteration, we first propose a Dynamic SepConv to extract relevant visual features guided by language and further propose Language Attentional Feature Modulation to improve the feature discriminability, then propose a Cross-Modal Semantic Reasoning module to perform global semantic reasoning by capturing both linguistic and visual information, and finally updates and corrects the visual features of the predicted object based on semantic information. Moreover, we further propose a Cross-Modal ASPP to capture richer visual information referred to in the global semantics of the language expression from larger receptive fields. Extensive experiments demonstrate that our proposed network significantly outperforms previous state-of-the-art methods on multiple datasets.
更多查看译文
关键词
Referring image segmentation,cross-modal recurrent network,global semantic reasoning
AI 理解论文
溯源树
样例
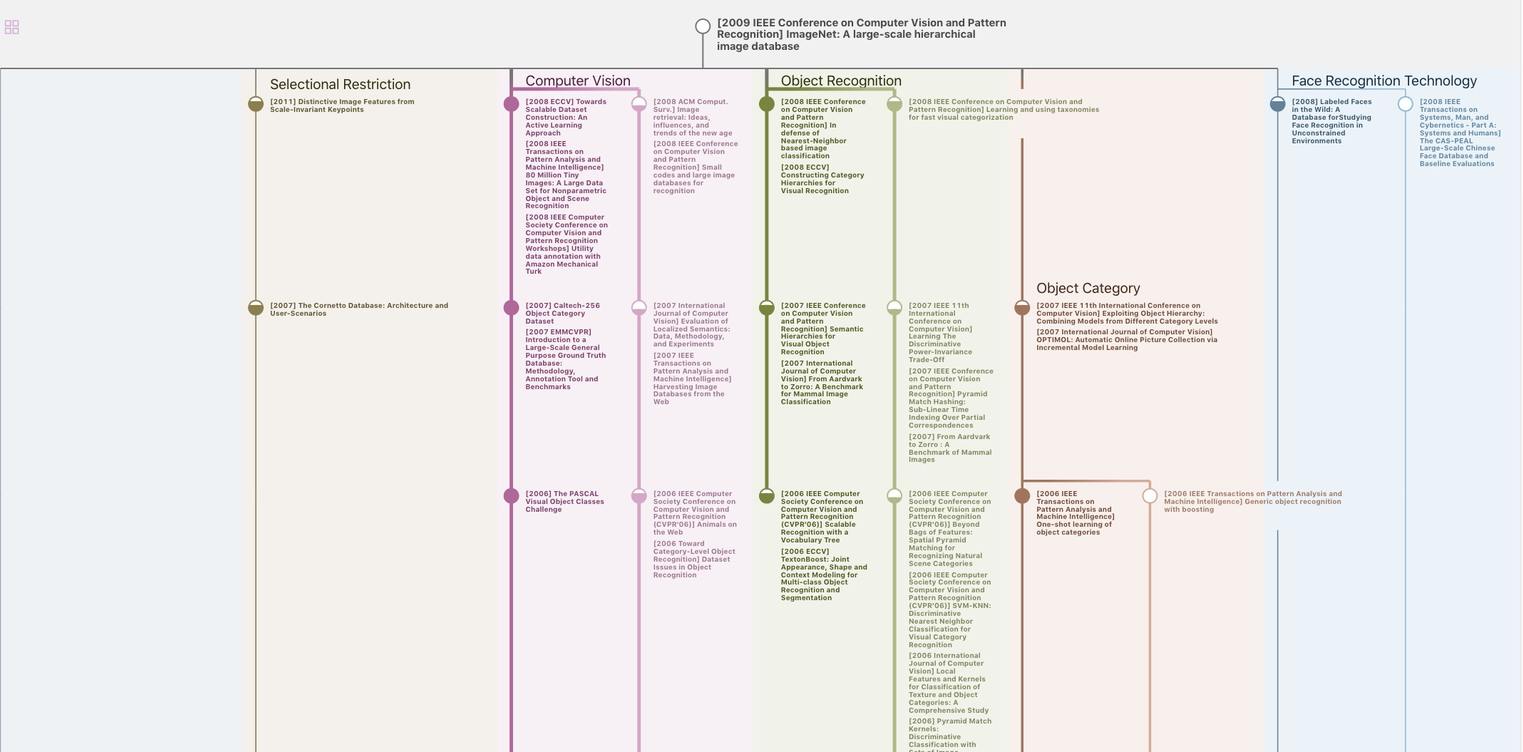
生成溯源树,研究论文发展脉络
Chat Paper
正在生成论文摘要