Adaptive Feature Selection With Augmented Attributes
IEEE Transactions on Pattern Analysis and Machine Intelligence(2023)
摘要
In many dynamic environment applications, with the evolution of data collection ways, the data attributes are incremental and the samples are stored with accumulated feature spaces gradually. For instance, in the neuroimaging-based diagnosis of neuropsychiatric disorders, with emerging of diverse testing ways, we get more brain image features over time. The accumulation of different types of features will unavoidably bring difficulties in manipulating the high-dimensional data. It is challenging to design an algorithm to select valuable features in this feature incremental scenario. To address this important but rarely studied problem, we propose a novel Adaptive Feature Selection method (AFS). It enables the reusability of the feature selection model trained on previous features and adapts it to fit the feature selection requirements on all features automatically. Besides, an ideal
$\ell _{0}$
-norm sparse constraint for feature selection is imposed with a proposed effective solving strategy. We present the theoretical analyses about the generalization bound and convergence behavior. After tackling this problem in a one-shot case, we extend it to the multi-shot scenario. Plenty of experimental results demonstrate the effectiveness of reusing previous features and the superior of
$\ell _{0}$
-norm constraint in various aspects, together with its effectiveness in discriminating schizophrenic patients from healthy controls.
更多查看译文
关键词
Augmented attributes,feature selection, $\ell _{0}$ ℓ 0 -norm,reusability
AI 理解论文
溯源树
样例
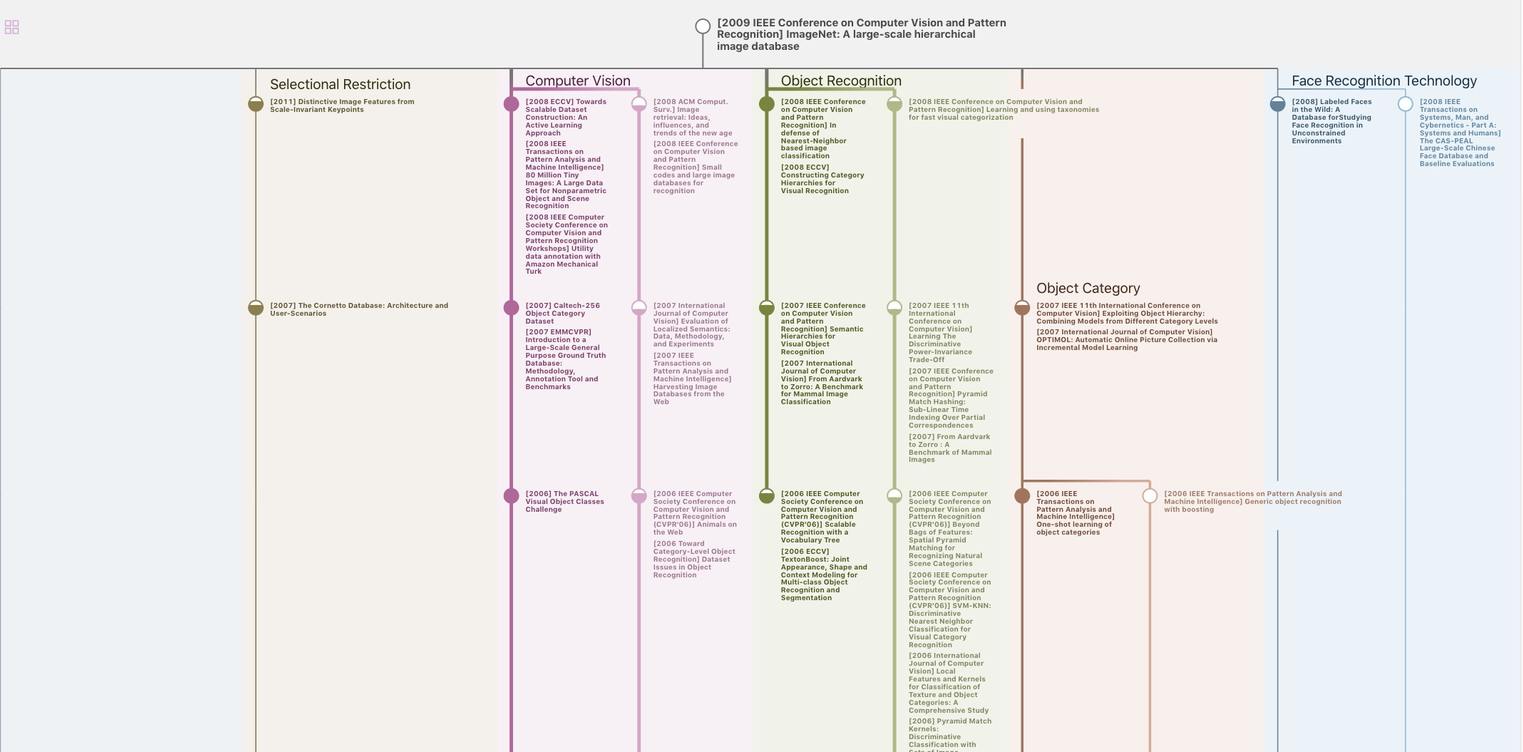
生成溯源树,研究论文发展脉络
Chat Paper
正在生成论文摘要