Characterizing the limits of shallow shotgun metagenomics for taxonomic profiling of human gut microbiota in clinical studies
crossref(2022)
摘要
Abstract Background Shallow shotgun metagenomics (SSM) has been recently suggested as a promising strategy to study human microbiota, providing nearly identical taxonomic profiles to deep shotgun metagenomics but at a sequencing cost as low as that of metabarcoding. To help clinical researchers determine whether shallow sequencing is appropriate for their projects, it is crucial to ascertain the accuracy of the information it provides, compared to deep sequencing. Here, we design a mapping-based workflow to build taxonomic profiles from SSM data and assess its accuracy at varying sequencing depths at both sample and cohort levels using extensive simulations and several public data sets. Results To identify genuinely present species and spuriously identified ones, we propose a novel data-driven filtering method based on machine learning techniques that largely outperforms basic filtering strategies based on pre defined thresholds, resulting in reliable taxonomic profiles at different sequencing depths, ranging from 50K to 10M reads/samples. Up to 90% of species with relative abundances higher than 4.10−4 were recovered correctly at 500K reads/sample showing that only information about rare taxa is lost at shallow depths. Furthermore, our results clearly show that SSM is able to correctly recover relevant biological signal from the confidently identified taxa, such as differences between groups of patients and diagnosis-like classification. Conclusions This study confirms that SSM is suitable for clinical research on human gut microbiota. We recommend that researchers should consider moving from 16S to SSM to limit biases in taxonomic profiles, or moving from deep to shallow sequencing, when functional analyses are not the main focus, to reduce costs and be able to include more patients in research projects.
更多查看译文
AI 理解论文
溯源树
样例
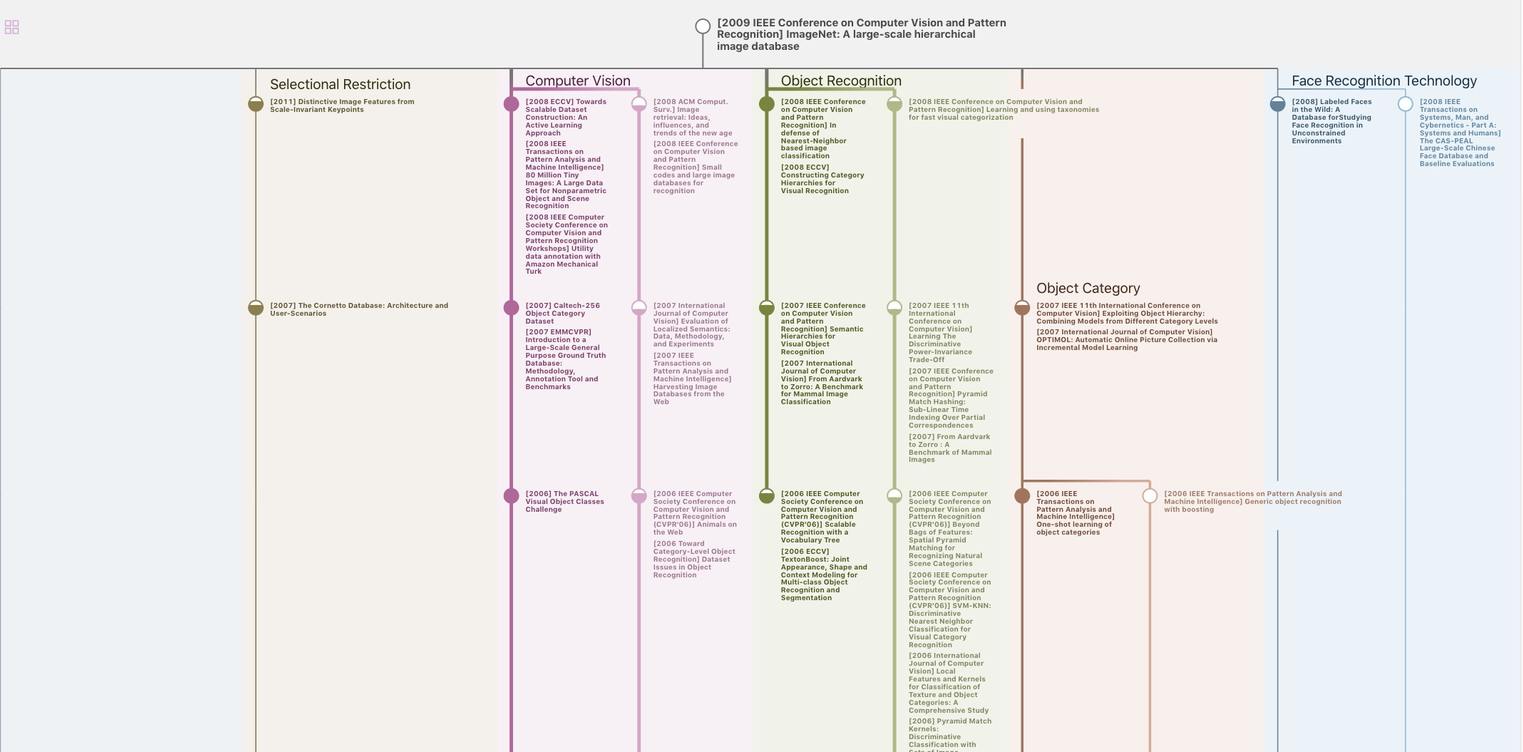
生成溯源树,研究论文发展脉络
Chat Paper
正在生成论文摘要