Evaluation of Catboost method for predicting weekly pan evaporation: Case study of subtropical and subhumid regions of India
crossref(2022)
摘要
Abstract Pan evaporation modelling and forecasting is needed to provide timely, continuously, and valuable water information to support water management. In this study we predict weekly pan evaporation using six machine learning approaches.The proposed models were:the multiple linear regression (MLR), the multiple nonlinear least-squares regression (MNLSR), the multivariate adaptive regression splines (MARS), the random forest regression (RF), the CatBoostand the M5 Model Tree (M5Tree).The algorithms were developed using data collected at Crop Research Center (CRC), Pantnagar- Uttarakhand, India. Stepwise regression was used as input variable selection algorithm for selecting the best relevant input from several meteorological variables. For models development we split the data into two subsets: the first three years reserved for model calibration i.e., validation, and the fourth-year data was used validation purpose.The rootmean square error (RMSE), Nash-Sutcliffe efficiency (NSE), Willmott index of agreement (WI), Pearson correlation coefficient (PCC), coefficient of determination (R2), and mean absolute error (MAE) were used to assess the effectiveness of weekly pan evaporation estimation models. CatBoost model based on four predictors was the most accurate at Pantnagar station, with NSE, WI, MAE, RMSE, PCC and R2 ranging from 0.9838 to 0.9877, 0.9960 to 0.9968, 0.1991 to 0.2078, 0.3643 to 0.3650 to 0.9955, 0.9925 and 0.9851 to 0.9911, respectively. Random Forest model has scored the second rank in terms of accuracy and effectiveness for mapping weekly pan evaporation followed by MARS, MNLSR, MLR, and M5Tree.
更多查看译文
AI 理解论文
溯源树
样例
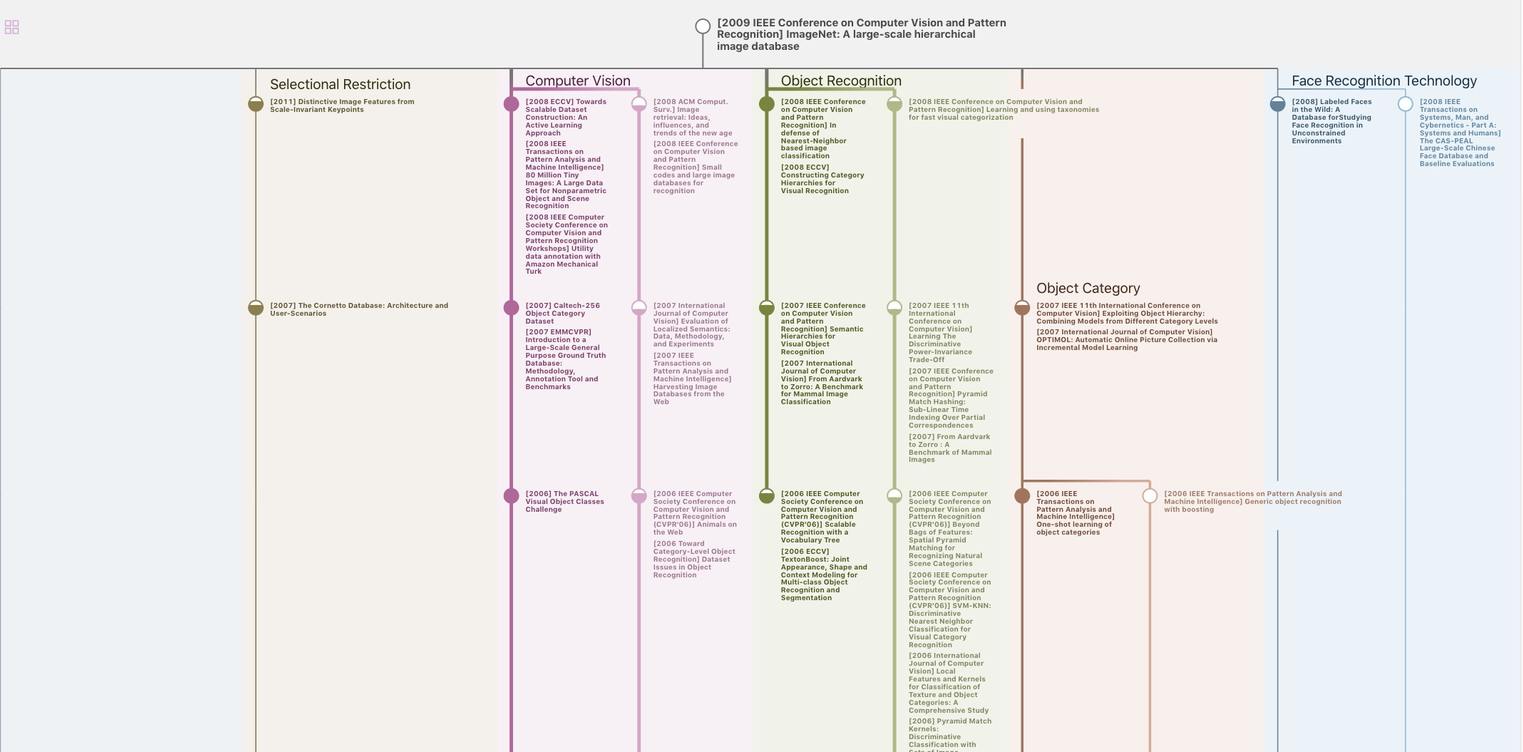
生成溯源树,研究论文发展脉络
Chat Paper
正在生成论文摘要