Microcrack segmentation of 3D CT images based on multi-task learning
Research Square (Research Square)(2022)
摘要
Abstract As a non-destructive testing technique, industrial CT (Computed Tomography) plays an important role in research and applications of the automatic defect detection for 3D CT images. In recent years, convolutional neural networks (CNNs) have shown great potential in image recognition and segmentation tasks. For industrial 3D CT image detection, how to segment microstructural cracks is the main task at present. The challenge of this task is to identify cracks with low grayscale contrast and small size under unbalanced sample conditions. To address these problems, we propose a crack segmentation method based on the multi-task deep convolutional neural networks. The network architecture consists of a segmentation task module for extracting cracks and a classification task module for improving accuracy, which share part feature maps. We introduce the ECA block based on attention mechanism from Chen et al. to improve the segmentation accuracy. Numerical experimental results show that the proposed method has the better performance compared with other methods. At the same time, the network proposed in this paper has good generalization ability. In the case of only using simulated images training, it still yields good prediction results for real CT images.
更多查看译文
关键词
3d ct images,ct images,segmentation,multi-task
AI 理解论文
溯源树
样例
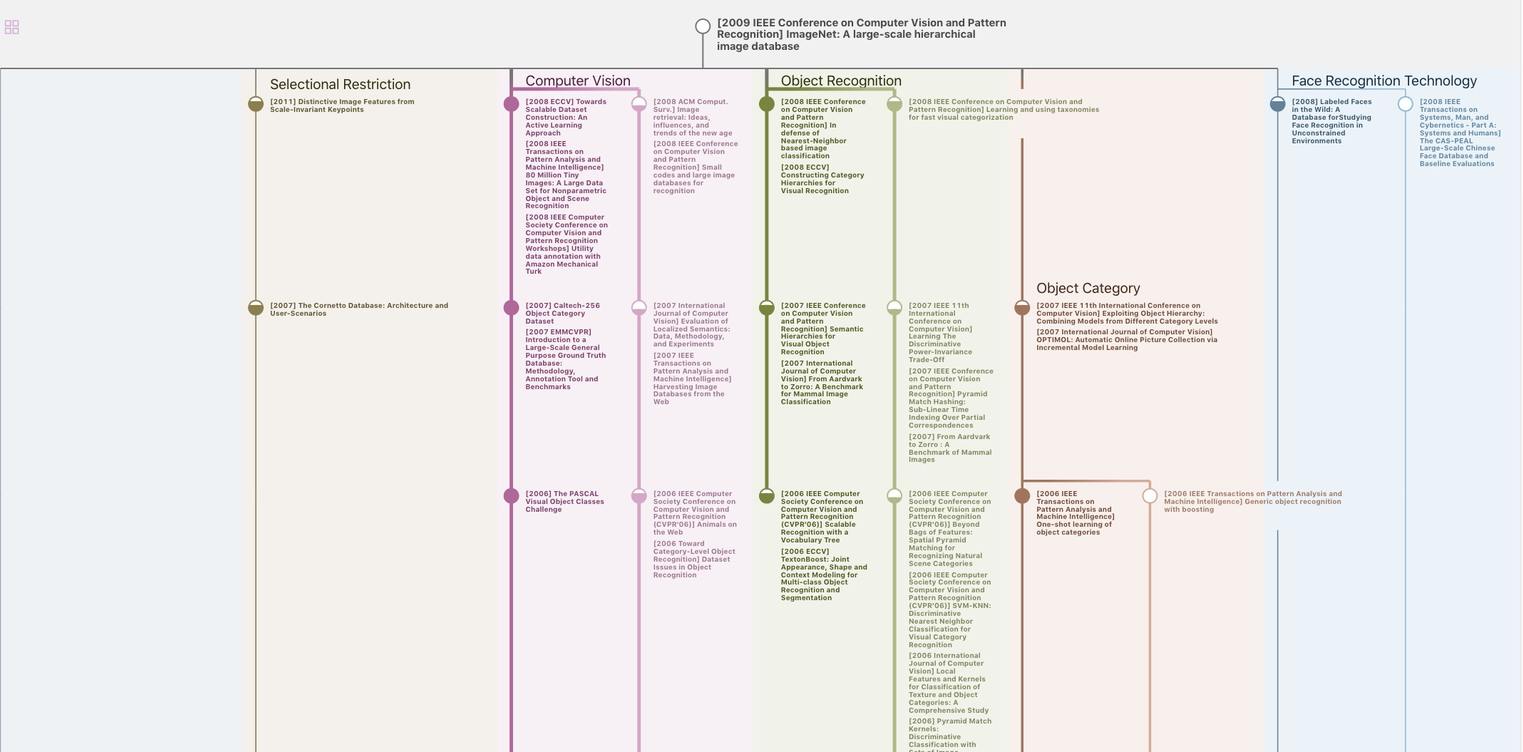
生成溯源树,研究论文发展脉络
Chat Paper
正在生成论文摘要