A Fully Interpretable First-Order TSK Fuzzy System and Its Training With Negative Entropic and Rule-Stability-Based Regularization
IEEE Transactions on Fuzzy Systems(2023)
摘要
While interpretable antecedent parts of first-order Takagi–Sugeno–Kang (TSK) fuzzy rules can be properly acquired by adopting some clustering methods, this study aims at avoiding the commonly used yet fully incomprehensive consequent parts and their intractable training, and simultaneously seeking for enhanced generalization performance by determining the weight of each rule. The central idea is to build a mathematically equivalent bridge between a Gaussian mixture model (GMM) and a fully interpretable first-order TSK fuzzy system called FIMG-TSK, with the help of Gaussian-mixture's mean. The resultant FIMG-TSK has a simple expected output expression without summation-to-one defuzzification, which will be helpful in inducing both smaller output variance and a negative entropic and rule-stability-based regularizer for enhancing the generalization performance. After revealing three factors affecting the output stability of FIMG-TSK, the negative entropic and rule-stability-based regularizer is designed through both these factors and the squared entropy to make the output variance of FIMG-TSK as small as possible. Accordingly, a novel training method, whose objective function takes the proposed regularizer as an additional term and hence compromises both accuracy and output stability of FIMG-TSK, is developed to quickly provide an analytical solution to the weight of each rule. The effectiveness of the proposed training method is manifested by the experimental results on ten regression datasets.
更多查看译文
关键词
Entropic and rule-stability-based regularizer, first-order Takagi-Sugeno-Kang (TSK) fuzzy system, fuzzy rules, Gaussian mixture model (GMM), generalization performance, interpretability
AI 理解论文
溯源树
样例
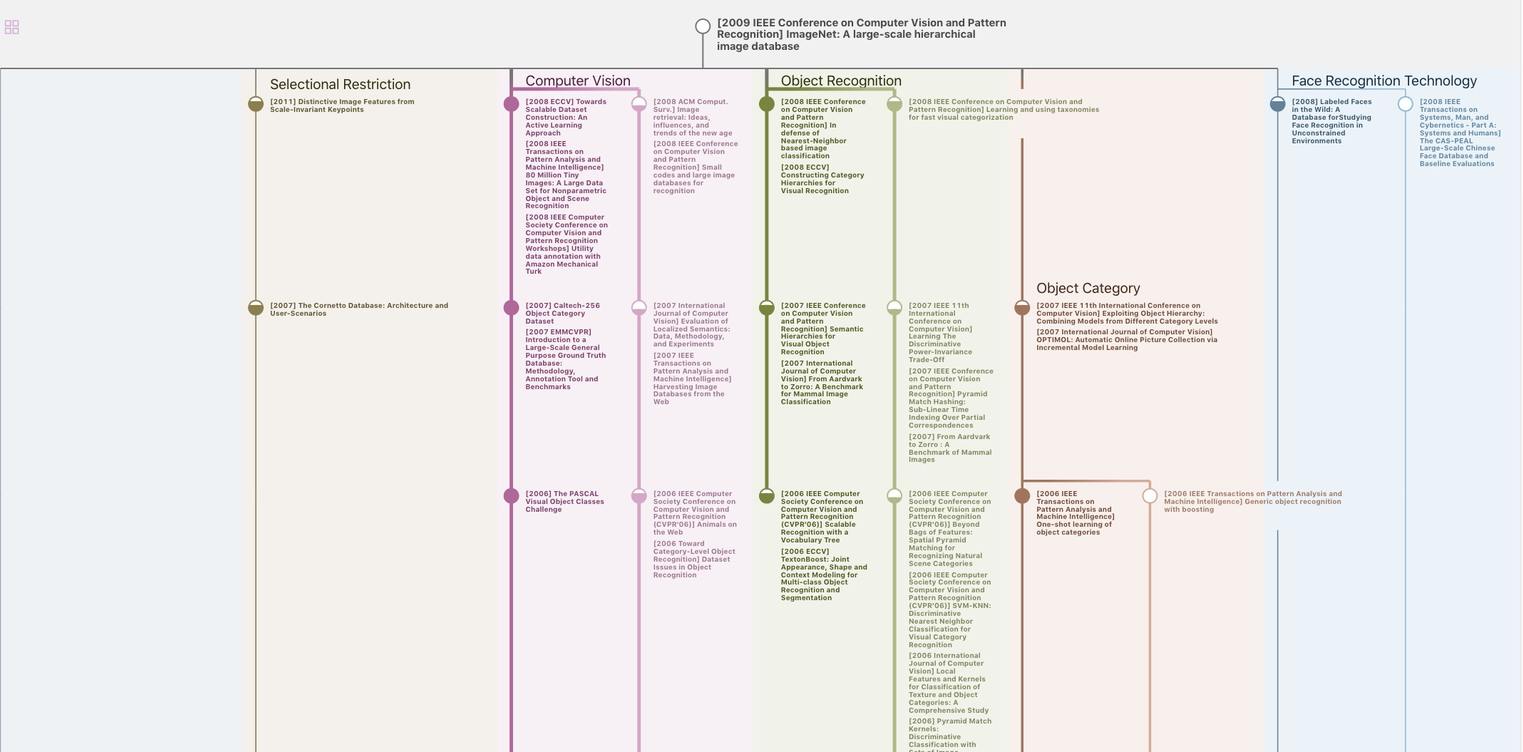
生成溯源树,研究论文发展脉络
Chat Paper
正在生成论文摘要