Learning Structure-Guided Diffusion Model for 2D Human Pose Estimation
CoRR(2023)
摘要
One of the mainstream schemes for 2D human pose estimation (HPE) is learning keypoints heatmaps by a neural network. Existing methods typically improve the quality of heatmaps by customized architectures, such as high-resolution representation and vision Transformers. In this paper, we propose \textbf{DiffusionPose}, a new scheme that formulates 2D HPE as a keypoints heatmaps generation problem from noised heatmaps. During training, the keypoints are diffused to random distribution by adding noises and the diffusion model learns to recover ground-truth heatmaps from noised heatmaps with respect to conditions constructed by image feature. During inference, the diffusion model generates heatmaps from initialized heatmaps in a progressive denoising way. Moreover, we further explore improving the performance of DiffusionPose with conditions from human structural information. Extensive experiments show the prowess of our DiffusionPose, with improvements of 1.6, 1.2, and 1.2 mAP on widely-used COCO, CrowdPose, and AI Challenge datasets, respectively.
更多查看译文
关键词
2d human pose estimation,diffusion model,learning,structure-guided
AI 理解论文
溯源树
样例
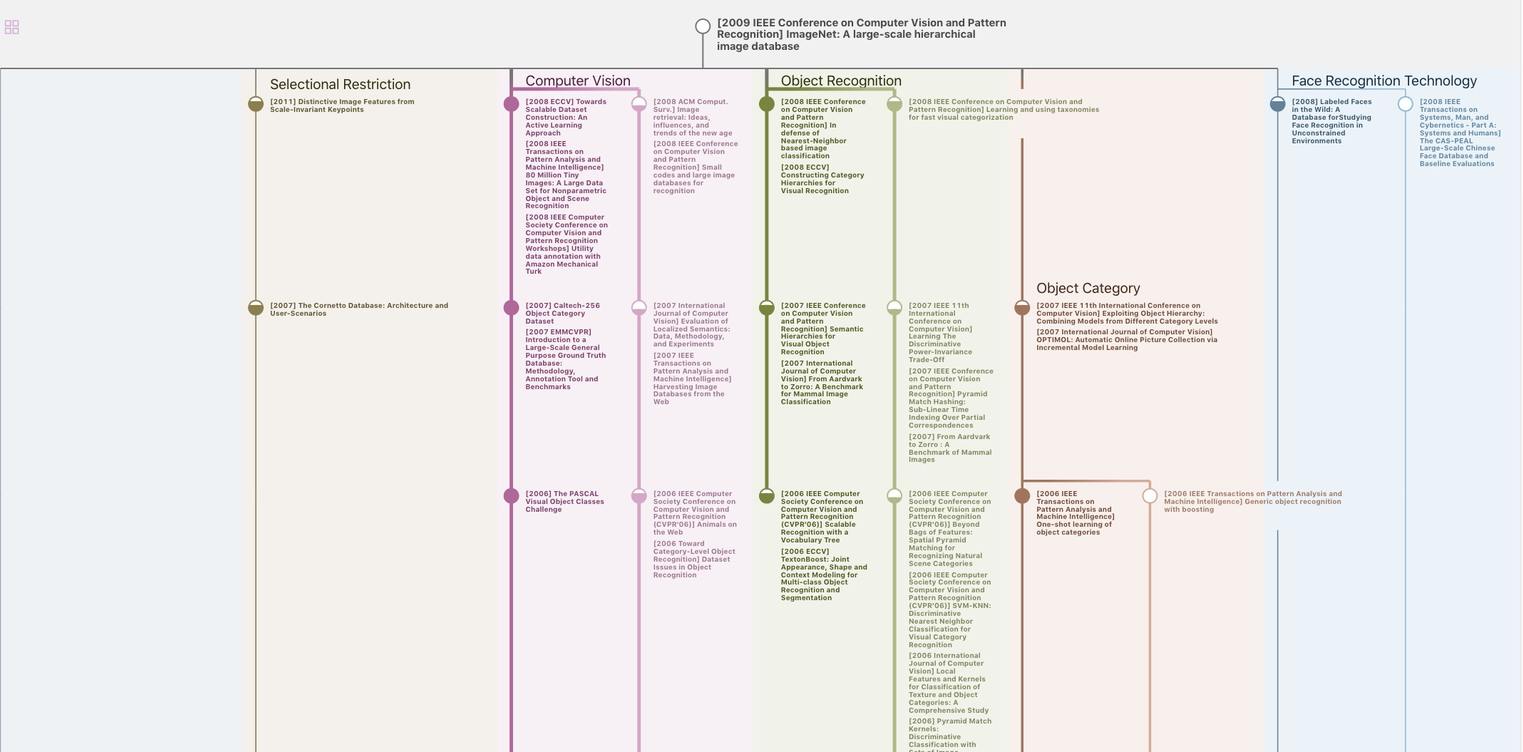
生成溯源树,研究论文发展脉络
Chat Paper
正在生成论文摘要