Cross-inferential networks for source-free unsupervised domain adaptation
2023 IEEE INTERNATIONAL CONFERENCE ON IMAGE PROCESSING, ICIP(2023)
摘要
One central challenge in source-free unsupervised domain adaptation (UDA) is the lack of an effective approach to evaluate the prediction results of the adapted network model in the target domain. To address this challenge, we propose to explore a new method called cross-inferential networks (CIN). Our main idea is that, when we adapt the network model to predict the sample labels from encoded features, we use these prediction results to construct new training samples with derived labels to learn a new examiner network that performs a different but compatible task in the target domain. Specifically, in this work, the base network model is performing image classification while the examiner network is tasked to perform relative ordering of triplets of samples whose training labels are carefully constructed from the prediction results of the base network model. Two similarity measures, cross-network correlation matrix similarity and attention consistency, are then developed to provide important guidance for the UDA process. Our experimental results on benchmark datasets demonstrate that our proposed CIN approach can significantly improve the performance of source-free UDA.
更多查看译文
关键词
Unsupervised domain adaptation,Attention,Correlation matrix,Consistency
AI 理解论文
溯源树
样例
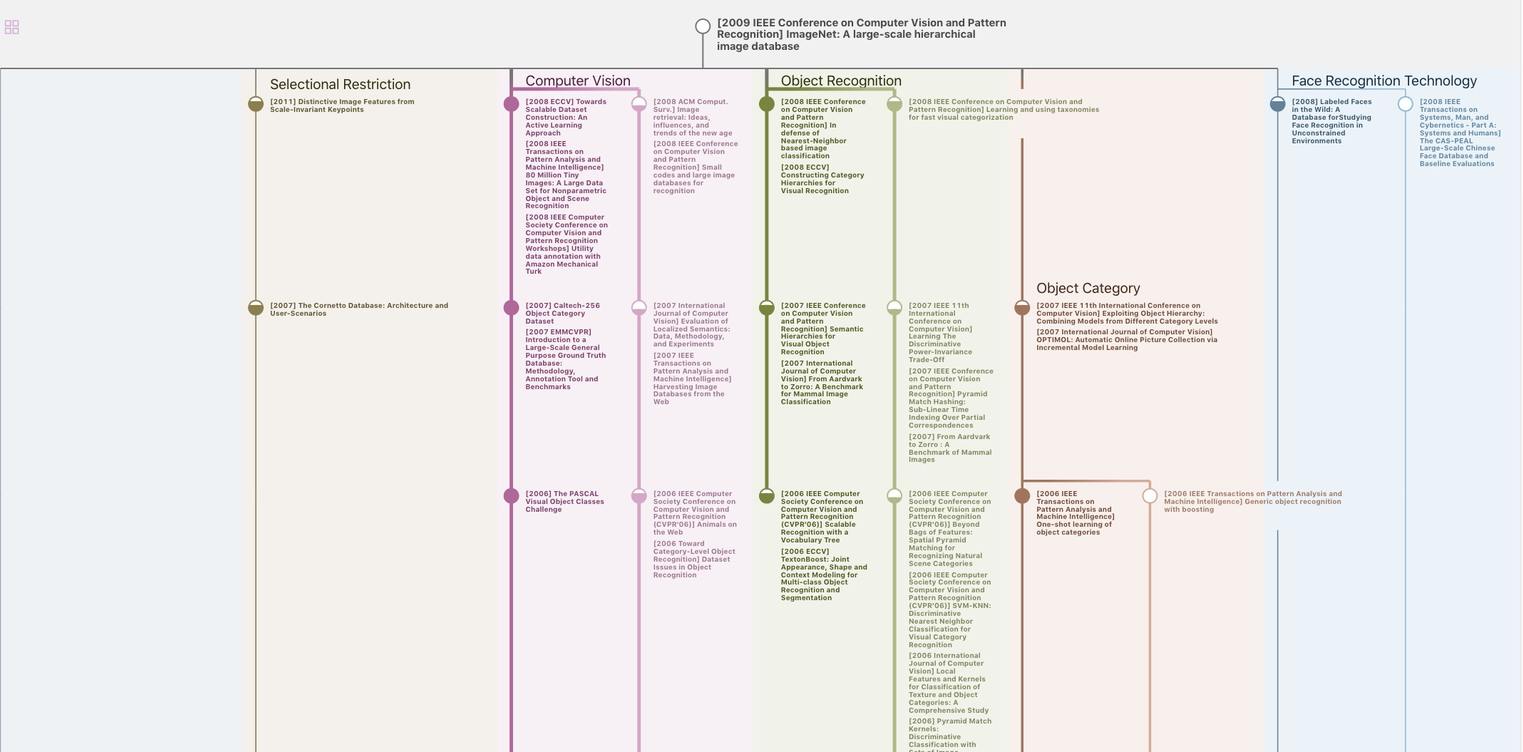
生成溯源树,研究论文发展脉络
Chat Paper
正在生成论文摘要