Restore Translation Using Equivariant Neural Networks.
CoRR(2023)
摘要
Invariance to spatial transformations such as translations and rotations is a desirable property and a basic design principle for classification neural networks. However, the commonly used convolutional neural networks (CNNs) are actually very sensitive to even small translations. There exist vast works to achieve exact or approximate transformation invariance by designing transformation-invariant models or assessing the transformations. These works usually make changes to the standard CNNs and harm the performance on standard datasets. In this paper, rather than modifying the classifier, we propose a pre-classifier restorer to recover translated (or even rotated) inputs to the original ones which will be fed into any classifier for the same dataset. The restorer is based on a theoretical result which gives a sufficient and necessary condition for an affine operator to be translational equivariant on a tensor space.
更多查看译文
关键词
equivariant neural networks,translation,neural networks
AI 理解论文
溯源树
样例
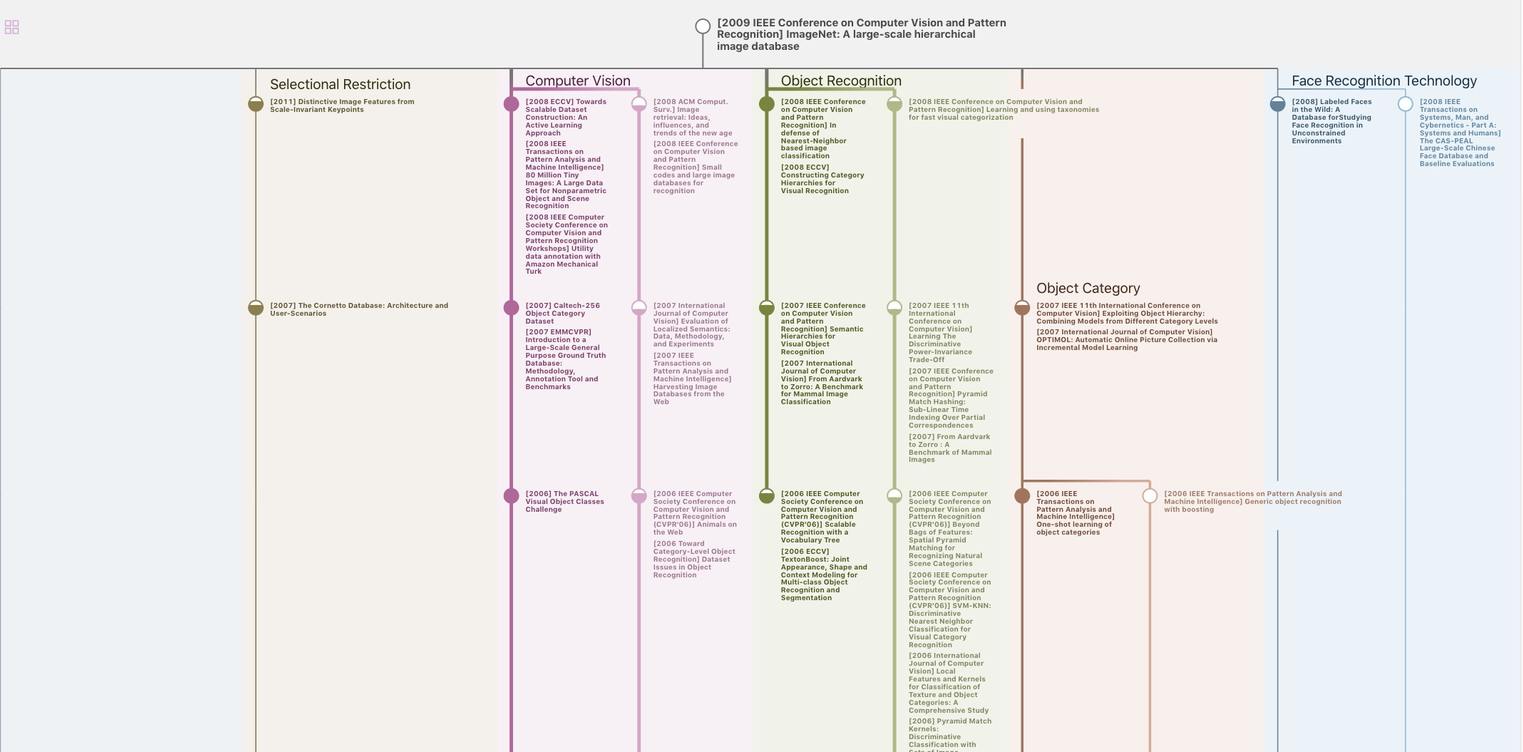
生成溯源树,研究论文发展脉络
Chat Paper
正在生成论文摘要