Computationally assisted quality control for public health data streams
CoRR(2023)
摘要
Irregularities in public health data streams (like COVID-19 Cases) hamper data-driven decision-making for public health stakeholders. A real-time, computer-generated list of the most important, outlying data points from thousands of daily-updated public health data streams could assist an expert reviewer in identifying these irregularities. However, existing outlier detection frameworks perform poorly on this task because they do not account for the data volume or for the statistical properties of public health streams. Accordingly, we developed FlaSH (Flagging Streams in public Health), a practical outlier detection framework for public health data users that uses simple, scalable models to capture these statistical properties explicitly. In an experiment where human experts evaluate FlaSH and existing methods (including deep learning approaches), FlaSH scales to the data volume of this task, matches or exceeds these other methods in mean accuracy, and identifies the outlier points that users empirically rate as more helpful. Based on these results, FlaSH has been deployed on data streams used by public health stakeholders.
更多查看译文
关键词
assisted quality control,quality control,health,data
AI 理解论文
溯源树
样例
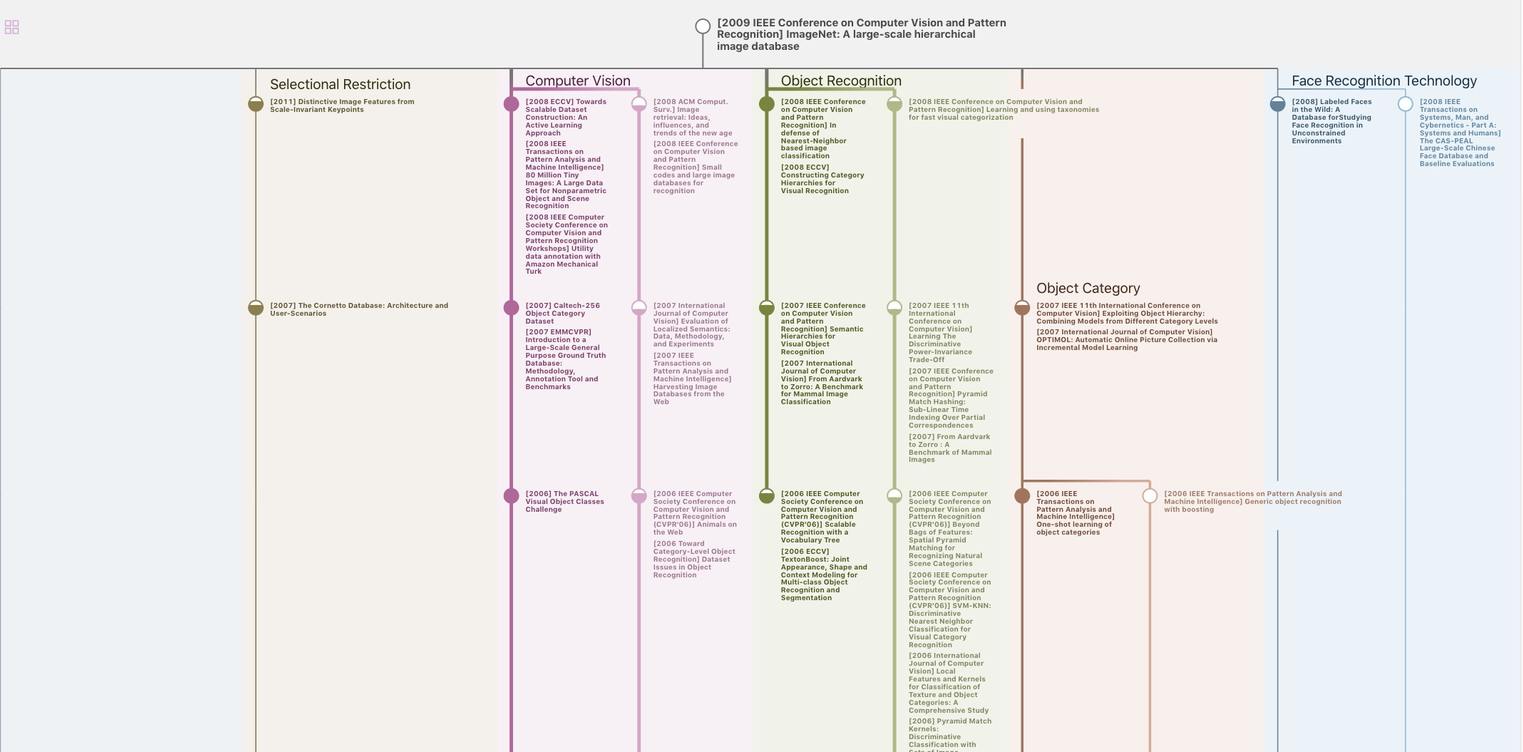
生成溯源树,研究论文发展脉络
Chat Paper
正在生成论文摘要