Leveraging Cross-Utterance Context For ASR Decoding
CoRR(2023)
摘要
While external language models (LMs) are often incorporated into the decoding stage of automated speech recognition systems, these models usually operate with limited context. Cross utterance information has been shown to be beneficial during second pass re-scoring, however this limits the hypothesis space based on the local information available to the first pass LM. In this work, we investigate the incorporation of long-context transformer LMs for cross-utterance decoding of acoustic models via beam search, and compare against results from n-best rescoring. Results demonstrate that beam search allows for an improved use of cross-utterance context. When evaluating on the long-format dataset AMI, results show a 0.7\% and 0.3\% absolute reduction on dev and test sets compared to the single-utterance setting, with improvements when including up to 500 tokens of prior context. Evaluations are also provided for Tedlium-1 with less significant improvements of around 0.1\% absolute.
更多查看译文
关键词
cross-utterance
AI 理解论文
溯源树
样例
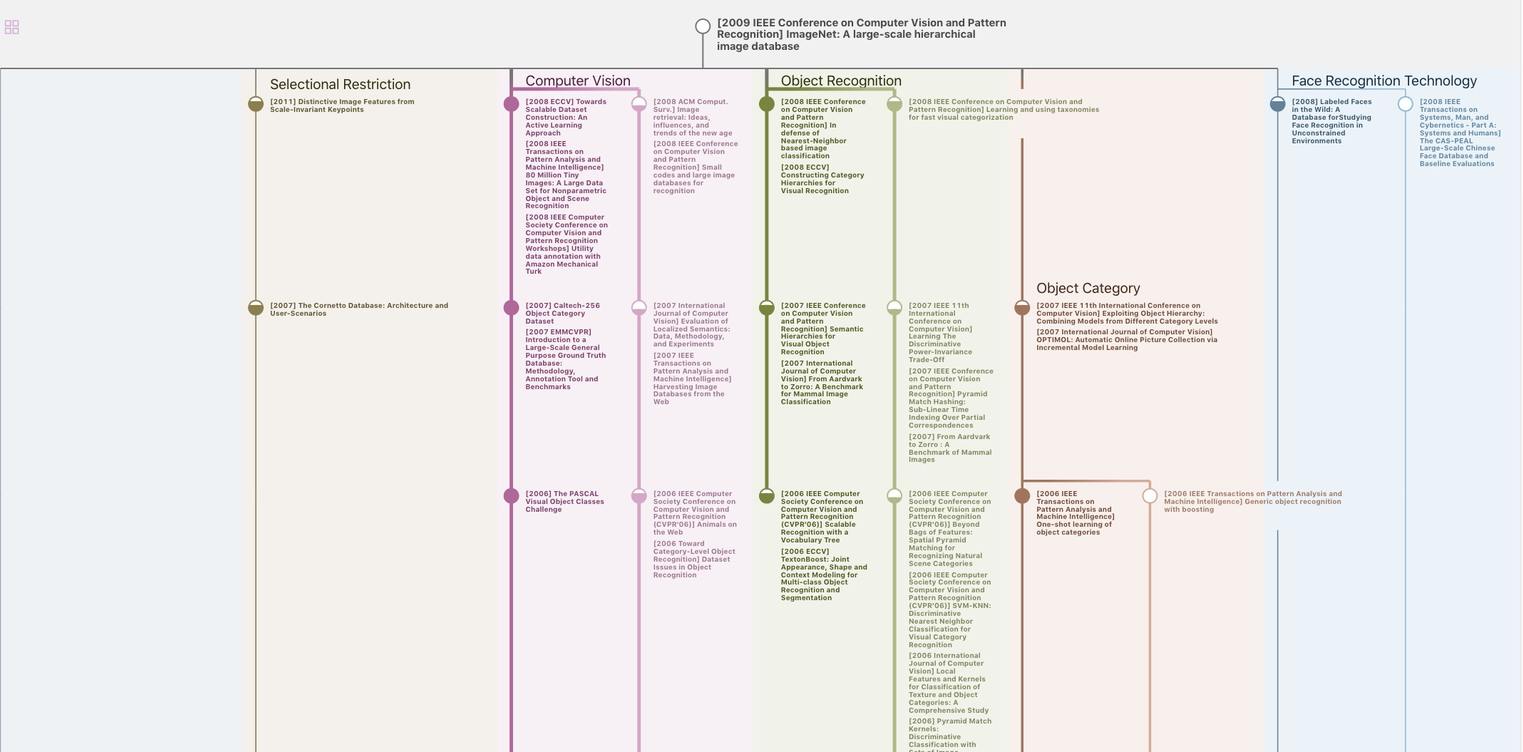
生成溯源树,研究论文发展脉络
Chat Paper
正在生成论文摘要