GraMMaR: Ground-aware Motion Model for 3D Human Motion Reconstruction
CoRR(2023)
摘要
Demystifying complex human-ground interactions is essential for accurate and realistic 3D human motion reconstruction from RGB videos, as it ensures consistency between the humans and the ground plane. Prior methods have modeled human-ground interactions either implicitly or in a sparse manner, often resulting in unrealistic and incorrect motions when faced with noise and uncertainty. In contrast, our approach explicitly represents these interactions in a dense and continuous manner. To this end, we propose a novel Ground-aware Motion Model for 3D Human Motion Reconstruction, named GraMMaR, which jointly learns the distribution of transitions in both pose and interaction between every joint and ground plane at each time step of a motion sequence. It is trained to explicitly promote consistency between the motion and distance change towards the ground. After training, we establish a joint optimization strategy that utilizes GraMMaR as a dual-prior, regularizing the optimization towards the space of plausible ground-aware motions. This leads to realistic and coherent motion reconstruction, irrespective of the assumed or learned ground plane. Through extensive evaluation on the AMASS and AIST++ datasets, our model demonstrates good generalization and discriminating abilities in challenging cases including complex and ambiguous human-ground interactions. The code will be released.
更多查看译文
关键词
3d human motion reconstruction,ground-aware
AI 理解论文
溯源树
样例
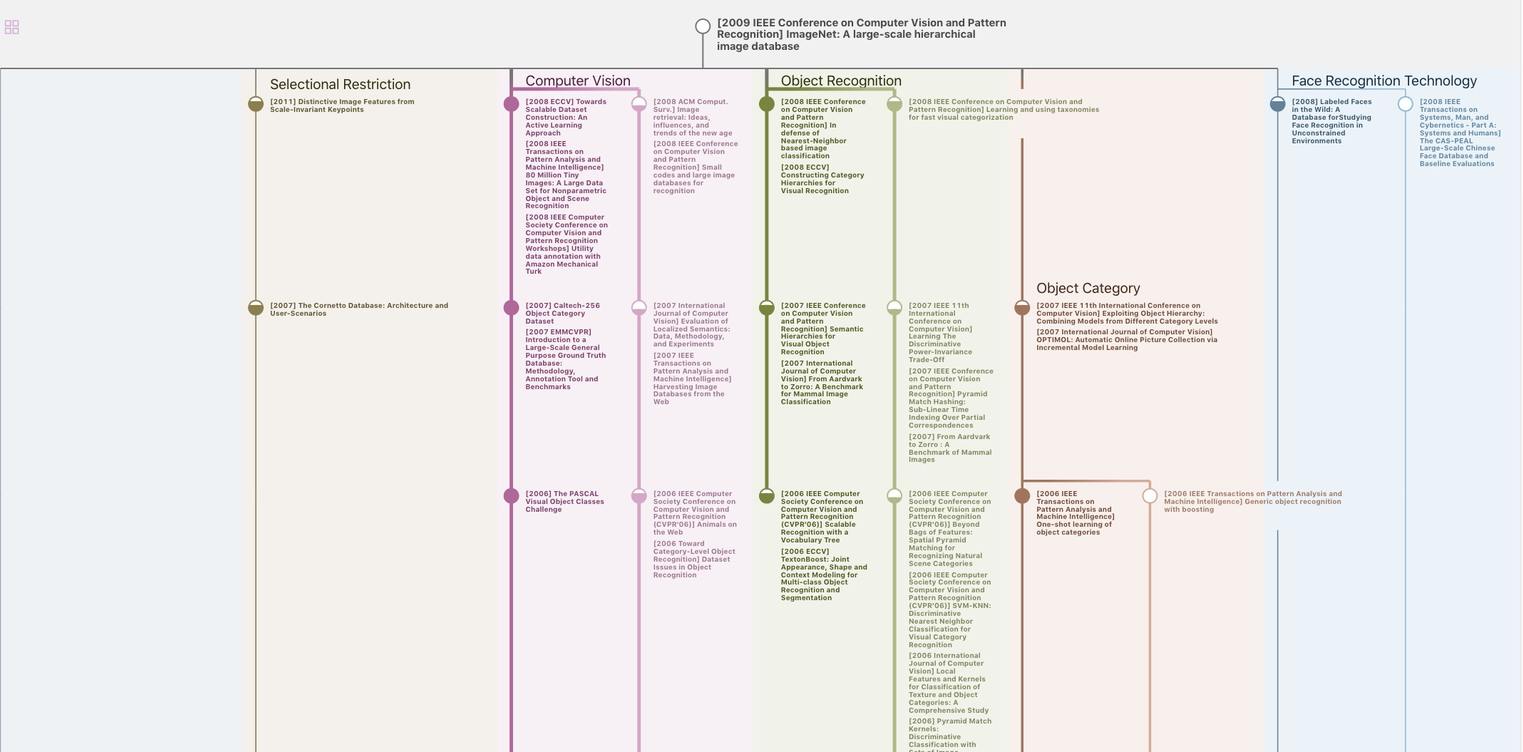
生成溯源树,研究论文发展脉络
Chat Paper
正在生成论文摘要