StarUnLink: identifying and mitigating signals from communications satellites in stellar spectral surveys
MONTHLY NOTICES OF THE ROYAL ASTRONOMICAL SOCIETY(2023)
摘要
A relatively new concern for the forthcoming massive spectroscopic sky surveys is the impact of contamination from low earth orbit satellites. Several hundred thousand of these satellites are licensed for launch in the next few years and it has been estimated that, in some cases, up to a few percent of spectra could be contaminated when using wide field, multi-fiber spectrographs. In this paper, a multi-staged approach is used to assess the practicality and limitations of identifying and minimizing the impact of satellite contamination in a WEAVE-like stellar spectral survey. We develop a series of convolutional-network based architectures to attempt identification, stellar parameter and chemical abundances recovery, and source separation of stellar spectra that we artificially contaminate with satellite (i.e. solar-like) spectra. Our results show that we are able to flag 67% of all contaminated sources at a precision level of 80% for low-resolution spectra and 96% for high-resolution spectra. Additionally, we are able to remove the contamination from the spectra and recover the clean spectra with a $<$1% reconstruction error. The errors in stellar parameter predictions reduce by up to a factor of 2-3 when either including contamination as an augmentation to a training set or by removing the contamination from the spectra, with overall better performance in the former case. The presented methods illustrate several machine learning mitigation strategies that can be implemented to improve stellar parameters for contaminated spectra in the WEAVE stellar spectroscopic survey and others like it.
更多查看译文
关键词
stellar spectral surveys,satellites,signals
AI 理解论文
溯源树
样例
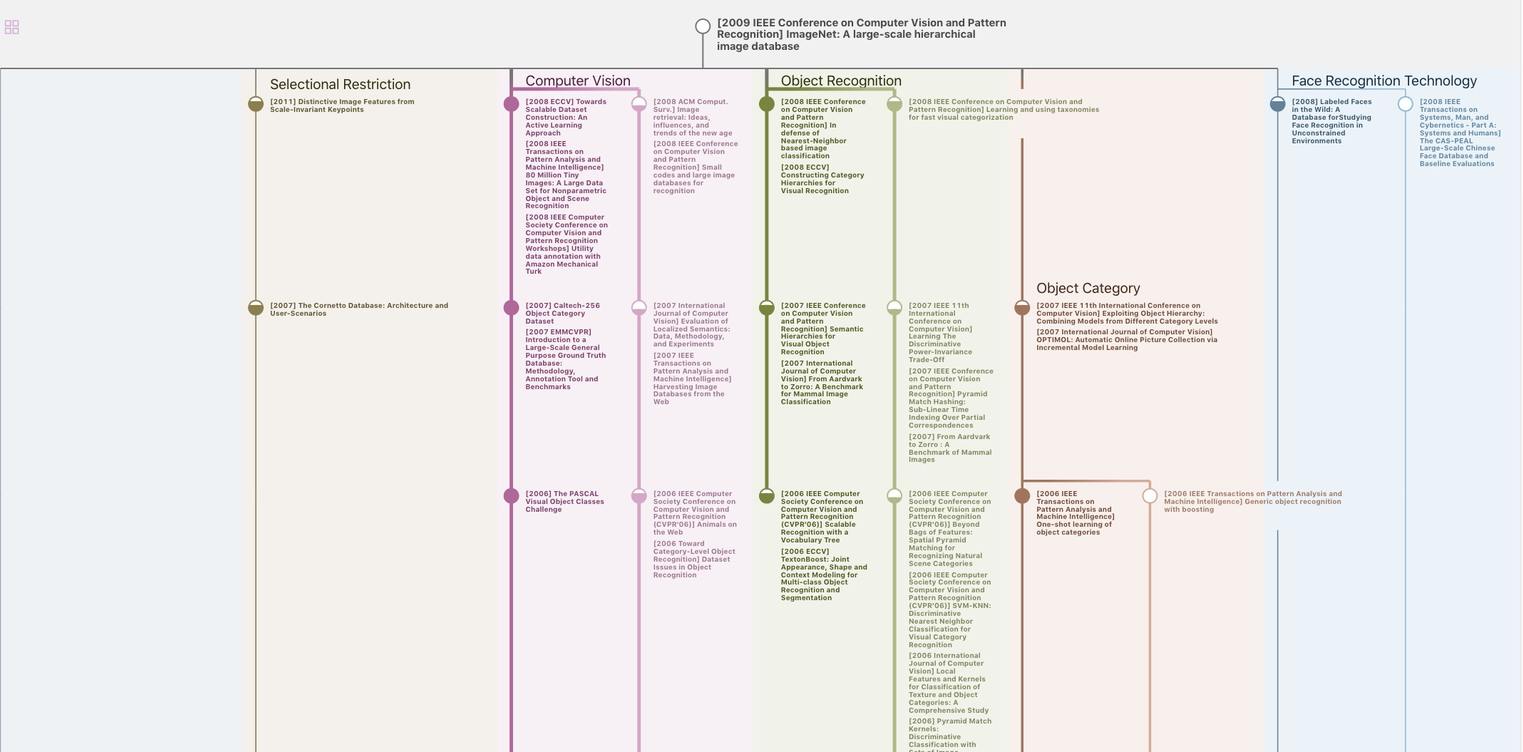
生成溯源树,研究论文发展脉络
Chat Paper
正在生成论文摘要