Assessment of alternative covariance functions for joint input-state estimation via Gaussian Process latent force models in structural dynamics
Mechanical Systems and Signal Processing(2024)
摘要
Digital technologies can be used to gather accurate information about the behavior of structural components for improving systems design, as well as for enabling advanced Structural Health Monitoring (SHM) strategies. New avenues for achieving automated and continuous structural assessment are opened up via development of virtualization approaches delivering so-called Digital Twins (DTs), i.e., digital mirrored representations of physical systems relying on fusion of simulation models and real-time monitoring data. In this framework, the main motivation of the work presented in this paper stems from the existing challenges in the implementation and deployment of a real-time predictive framework for virtualization of dynamic systems. Kalman-based filters are usually employed in this context to address the task of joint input-state prediction in structural dynamics. A Gaussian Process Latent Force Model (GPLFM) approach is exploited in this work to construct flexible data-driven a priori models for the unknown inputs, which are then coupled with a combined deterministic-stochastic state-space model of the structural component under study for Kalman-based input-state estimation. The use of Gaussian Process (GP) regression for this task overcomes the limitations of the conventionally adopted random-walk model, thus limiting the necessity of offline user-dependent calibration of this type of data assimilation methods. This paper proposes the use of alternative covariance functions for GP regression in structural dynamics. A theoretical analysis of the GPLFMs linked to the investigated covariance functions is offered. The outcome of this study provides insights into the applicability of each covariance type for GP-based input-state estimation. The proposed framework is validated via an illustrative simulated example, namely a 3 Degrees of Freedom (DOF) system subjected to an array of different loading scenarios. Additionally, the performance of the method is experimentally assessed on the task of joint input-state estimation during testing of a 3D-printed scaled Wind Turbine (WT) blade.
更多查看译文
关键词
Input-state estimation,Input modeling,Latent force model,Gaussian process,Covariance function
AI 理解论文
溯源树
样例
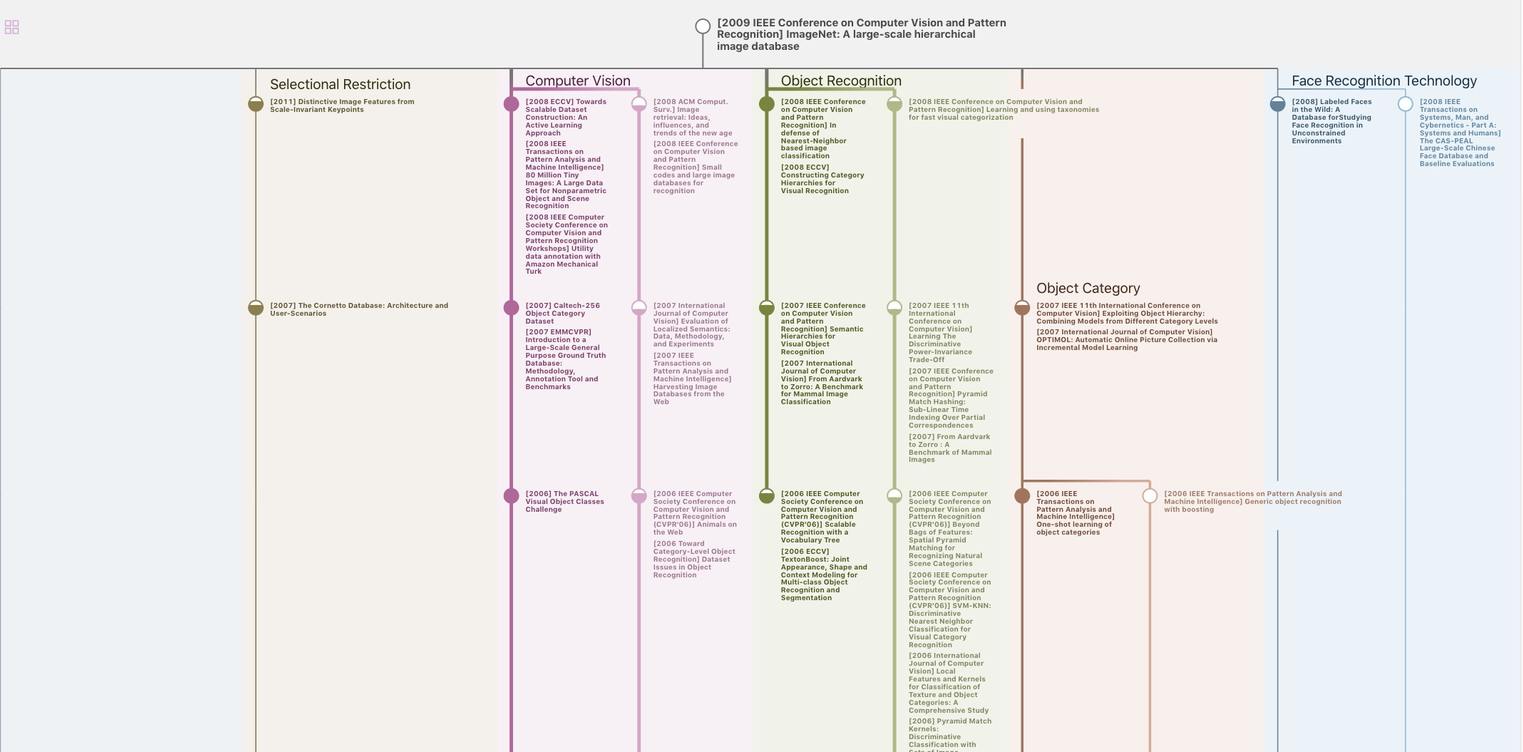
生成溯源树,研究论文发展脉络
Chat Paper
正在生成论文摘要