Tensorformer: Normalized Matrix Attention Transformer for High-quality Point Cloud Reconstruction
CoRR(2023)
摘要
Surface reconstruction from raw point clouds has been studied for decades in the computer graphics community, which is highly demanded by modeling and rendering applications nowadays. Classic solutions, such as Poisson surface reconstruction, require point normals as extra input to perform reasonable results. Modern transformer-based methods can work without normals, while the results are less fine-grained due to limited encoding performance in local fusion from discrete points. We introduce a novel normalized matrix attention transformer (Tensorformer) to perform high-quality reconstruction. The proposed matrix attention allows for simultaneous point-wise and channel-wise message passing, while the previous vector attention loses neighbor point information across different channels. It brings more degree of freedom in feature learning and thus facilitates better modeling of local geometries. Our method achieves state-of-the-art on two commonly used datasets, ShapeNetCore and ABC, and attains 4% improvements on IOU on ShapeNet. Our implementation will be released upon acceptance.
更多查看译文
关键词
Transformer,Point Cloud Reconstruction,Implicit Surface
AI 理解论文
溯源树
样例
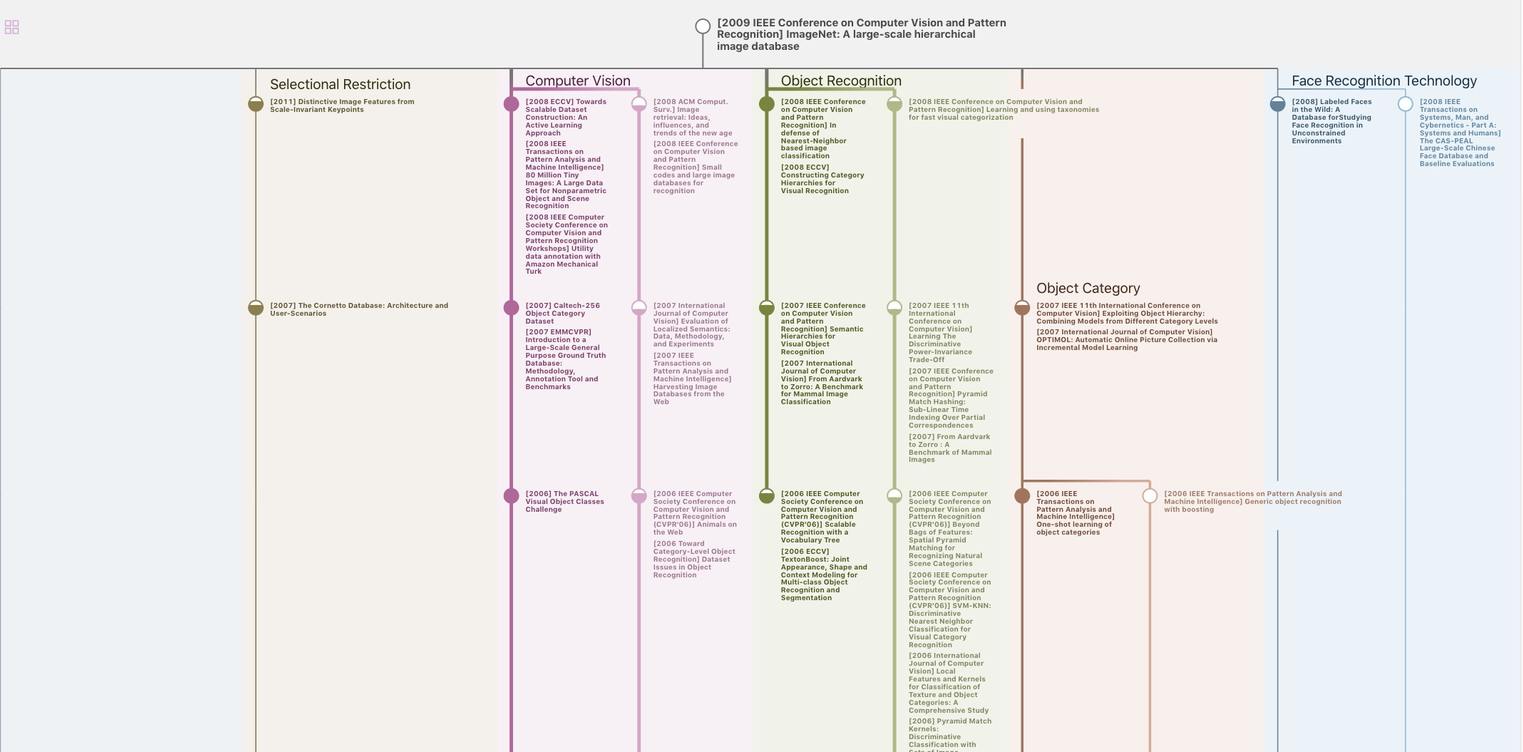
生成溯源树,研究论文发展脉络
Chat Paper
正在生成论文摘要