Differentially Private Video Activity Recognition
2024 IEEE/CVF Winter Conference on Applications of Computer Vision (WACV)(2023)
摘要
In recent years, differential privacy has seen significant advancements in image classification; however, its application to video activity recognition remains under-explored. This paper addresses the challenges of applying differential privacy to video activity recognition, which primarily stem from: (1) a discrepancy between the desired privacy level for entire videos and the nature of input data processed by contemporary video architectures, which are typically short, segmented clips; and (2) the complexity and sheer size of video datasets relative to those in image classification, which render traditional differential privacy methods inadequate. To tackle these issues, we propose Multi-Clip DP-SGD, a novel framework for enforcing video-level differential privacy through clip-based classification models. This method samples multiple clips from each video, averages their gradients, and applies gradient clipping in DP-SGD without incurring additional privacy loss. Moreover, we incorporate a parameter-efficient transfer learning strategy to make the model scalable for large-scale video datasets. Through extensive evaluations on the UCF-101 and HMDB-51 datasets, our approach exhibits impressive performance, achieving 81% accuracy with a privacy budget of epsilon=5 on UCF-101, marking a 76% improvement compared to a direct application of DP-SGD. Furthermore, we demonstrate that our transfer learning strategy is versatile and can enhance differentially private image classification across an array of datasets including CheXpert, ImageNet, CIFAR-10, and CIFAR-100.
更多查看译文
关键词
Algorithms,Video recognition and understanding,Algorithms,Explainable,fair,accountable,privacy-preserving,ethical computer vision
AI 理解论文
溯源树
样例
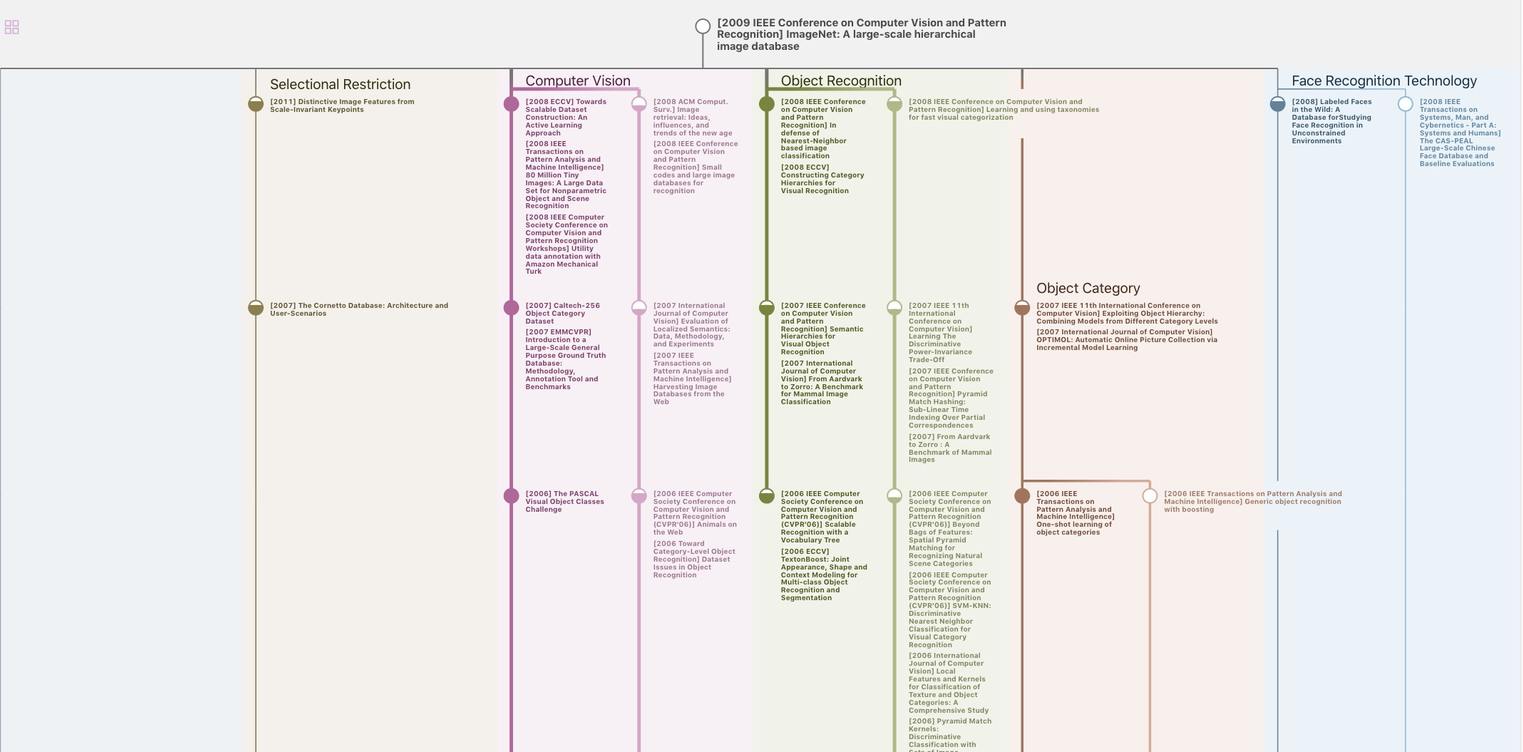
生成溯源树,研究论文发展脉络
Chat Paper
正在生成论文摘要