Physics-inspired spatiotemporal-graph AI ensemble for gravitational wave detection
CoRR(2023)
摘要
We introduce a novel method for gravitational wave detection that combines: 1) hybrid dilated convolution neural networks to accurately model both short- and long-range temporal sequential information of gravitational wave signals; and 2) graph neural networks to capture spatial correlations among gravitational wave observatories to consistently describe and identify the presence of a signal in a detector network. These spatiotemporal-graph AI models are tested for signal detection of gravitational waves emitted by quasi-circular, non-spinning and quasi-circular, spinning, non-precessing binary black hole mergers. For the latter case, we needed a dataset of 1.2 million modeled waveforms to densely sample this signal manifold. Thus, we reduced time-to-solution by training several AI models in the Polaris supercomputer at the Argonne Leadership Supercomputing Facility within 1.7 hours by distributing the training over 256 NVIDIA A100 GPUs, achieving optimal classification performance. This approach also exhibits strong scaling up to 512 NVIDIA A100 GPUs. We then created ensembles of AI models to process data from a three detector network, namely, the advanced LIGO Hanford and Livingston detectors, and the advanced Virgo detector. An ensemble of 2 AI models achieves state-of-the-art performance for signal detection, and reports seven misclassifications per decade of searched data, whereas an ensemble of 4 AI models achieves optimal performance for signal detection with two misclassifications for every decade of searched data. Finally, when we distributed AI inference over 128 GPUs in the Polaris supercomputer and 128 nodes in the Theta supercomputer, our AI ensemble is capable of processing a decade of gravitational wave data from a three detector network within 3.5 hours.
更多查看译文
关键词
gravitational wave detection,physics-inspired,spatiotemporal-graph
AI 理解论文
溯源树
样例
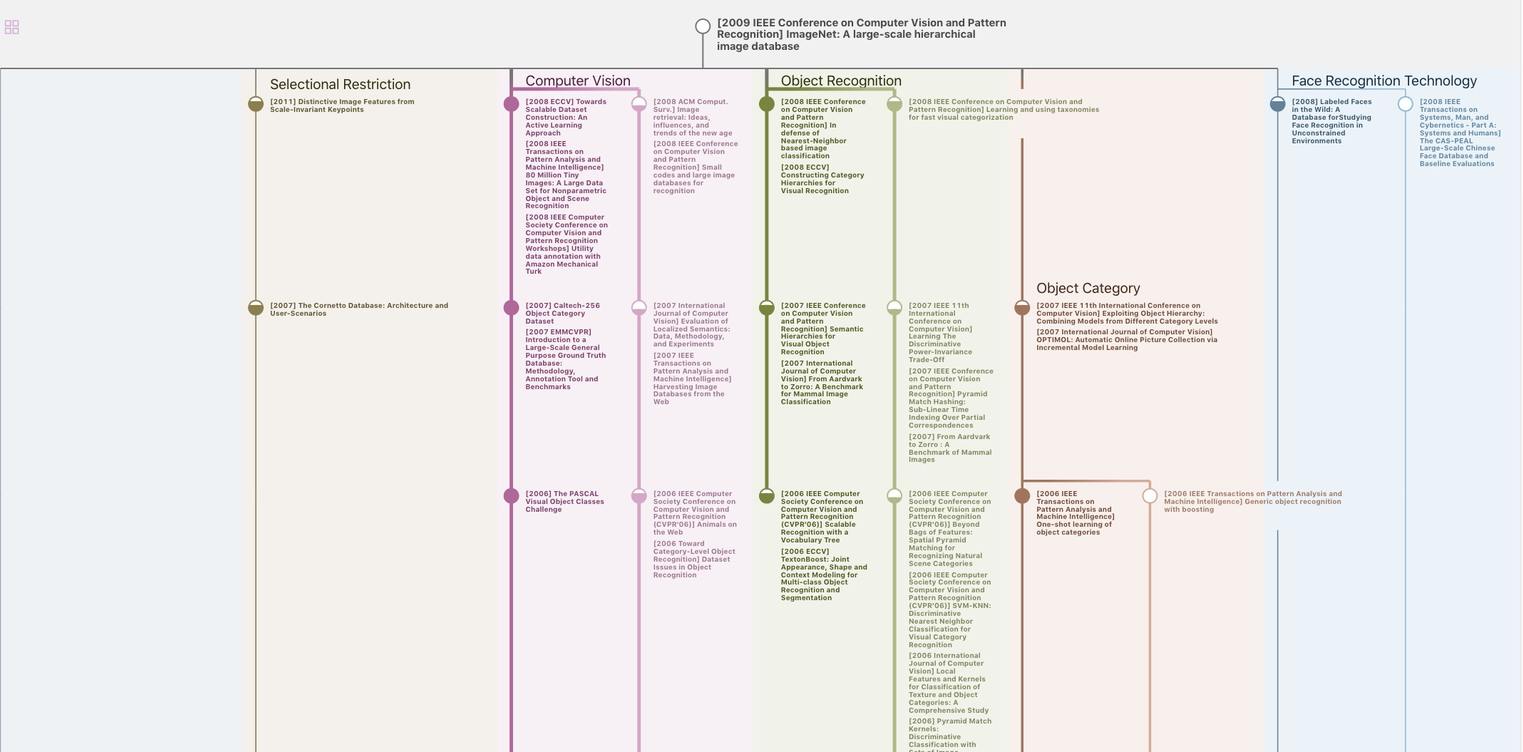
生成溯源树,研究论文发展脉络
Chat Paper
正在生成论文摘要