Constraining below-threshold radio source counts with machine learning
JOURNAL OF COSMOLOGY AND ASTROPARTICLE PHYSICS(2024)
摘要
We propose a machine -learning -based technique to determine the number density of radio sources as a function of their flux density, for use in next -generation radio surveys. The method uses a convolutional neural network trained on simulations of the radio sky to predict the number of sources in several flux bins. To train the network, we adopt a supervised approach wherein we simulate training data stemming from a large domain of possible number count models going down to fluxes a factor of 100 below the threshold for source detection. We test the model reconstruction capabilities as well as benchmark the expected uncertainties in the model predictions, observing good performance for fluxes down to a factor of ten below the threshold. This work demonstrates that the capabilities of simple deep learning models for radio astronomy can be useful tools for future surveys.
更多查看译文
关键词
surveys,Machine learning
AI 理解论文
溯源树
样例
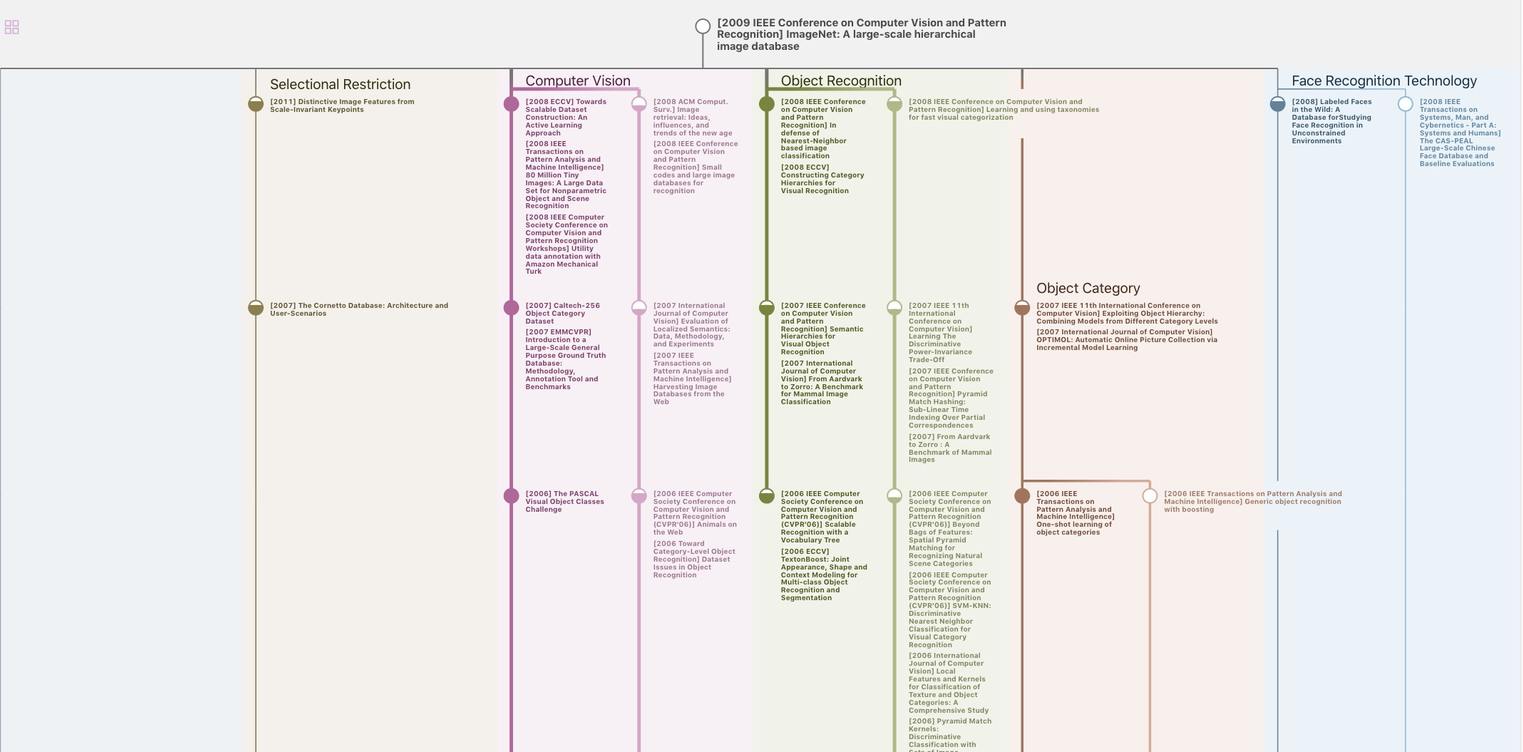
生成溯源树,研究论文发展脉络
Chat Paper
正在生成论文摘要