Trajectory Generation, Control, and Safety with Denoising Diffusion Probabilistic Models
CoRR(2023)
摘要
We present a framework for safety-critical optimal control of physical systems based on denoising diffusion probabilistic models (DDPMs). The technology of control barrier functions (CBFs), encoding desired safety constraints, is used in combination with DDPMs to plan actions by iteratively denoising trajectories through a CBF-based guided sampling procedure. At the same time, the generated trajectories are also guided to maximize a future cumulative reward representing a specific task to be optimally executed. The proposed scheme can be seen as an offline and model-based reinforcement learning algorithm resembling in its functionalities a model-predictive control optimization scheme with receding horizon in which the selected actions lead to optimal and safe trajectories.
更多查看译文
关键词
denoising diffusion,trajectory,safety,models
AI 理解论文
溯源树
样例
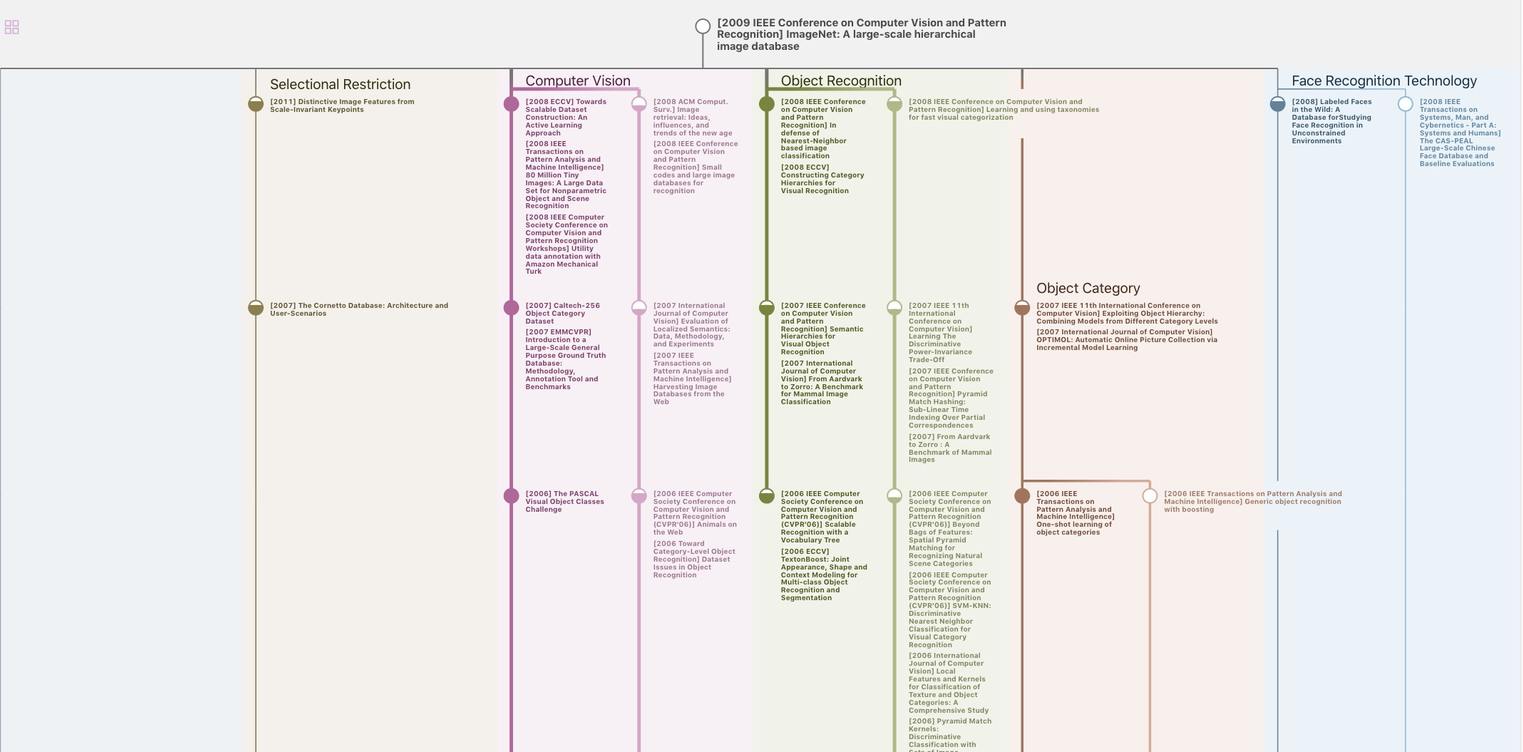
生成溯源树,研究论文发展脉络
Chat Paper
正在生成论文摘要