KnowPrefix-Tuning: A Two-Stage Prefix-Tuning Framework for Knowledge-Grounded Dialogue Generation
MACHINE LEARNING AND KNOWLEDGE DISCOVERY IN DATABASES: RESEARCH TRACK, ECML PKDD 2023, PT II(2023)
摘要
Existing knowledge-grounded conversation systems generate responses typically in a retrieve-then-generate manner. They require a large knowledge base and a strong knowledge retrieval component, which is time- and resource-consuming. In this paper, we address the challenge by leveraging the inherent knowledge encoded in the pre-trained language models (PLMs). We propose Knowledgeable Prefix Tuning (KnowPrefix-Tuning), a two-stage tuning framework, bypassing the retrieval process in a knowledge-grounded conversation system by injecting prior knowledge into the lightweight knowledge prefix. The knowledge prefix is a sequence of continuous knowledge-specific vectors that can be learned during training. In addition, we propose a novel interactive re-parameterization mechanism that allows the prefix to interact fully with the PLM during the optimization of response generation. Experimental results demonstrate that KnowPrefix-Tuning outperforms fine-tuning and other lightweight tuning approaches, and performs comparably with strong retrieval-based baselines while being 3x faster during inference (The code is available at https://github.com/fantast4ever/KnowPrefix-Tuning.)
更多查看译文
关键词
Dialogue generation,Parameter-efficient fine-tuning,Knowledge-grounded dialogue,Pre-trained language models
AI 理解论文
溯源树
样例
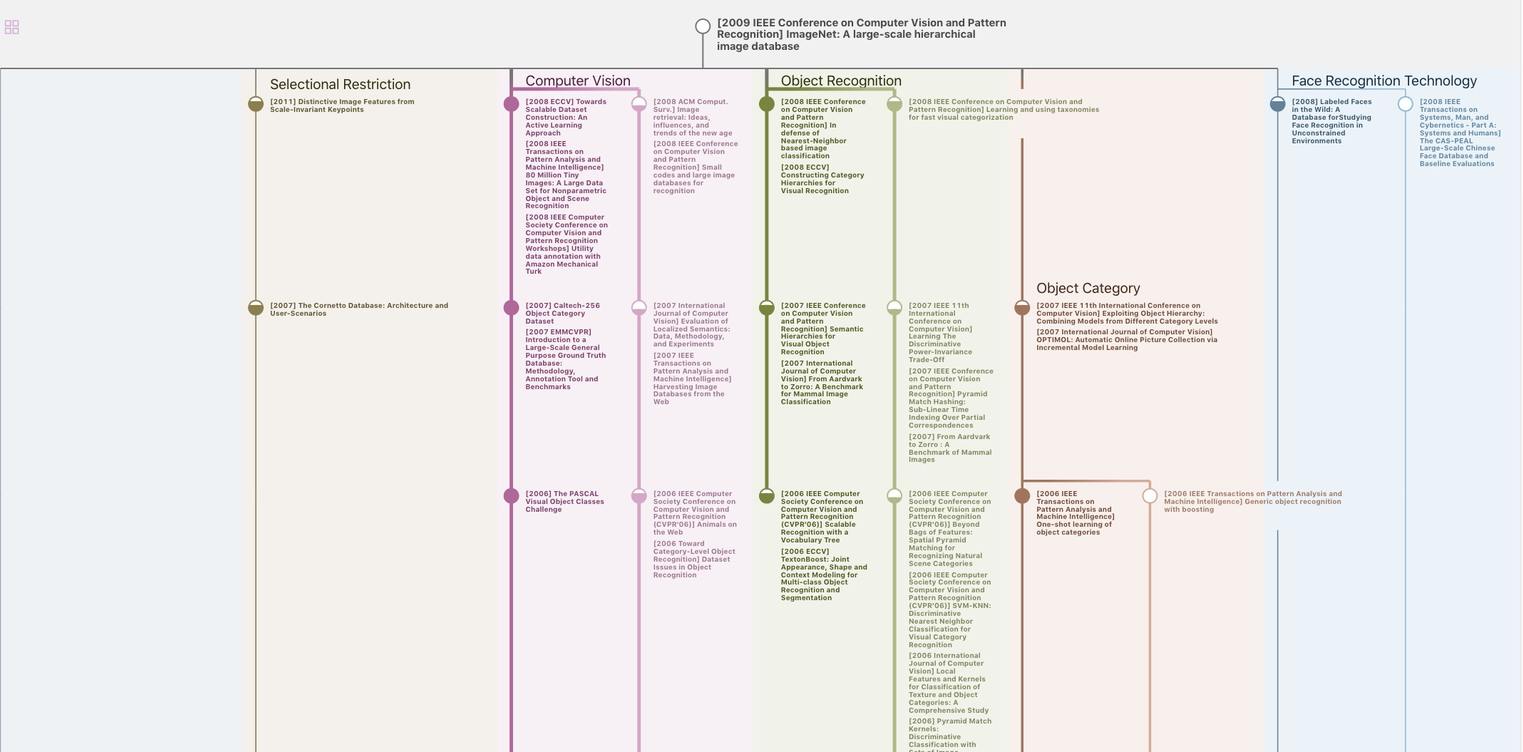
生成溯源树,研究论文发展脉络
Chat Paper
正在生成论文摘要