Multi-Dimensional Refinement Graph Convolutional Network with Robust Decouple Loss for Fine-Grained Skeleton-Based Action Recognition
CoRR(2023)
摘要
Graph convolutional networks have been widely used in skeleton-based action recognition. However, existing approaches are limited in fine-grained action recognition due to the similarity of inter-class data. Moreover, the noisy data from pose extraction increases the challenge of fine-grained recognition. In this work, we propose a flexible attention block called Channel-Variable Spatial-Temporal Attention (CVSTA) to enhance the discriminative power of spatial-temporal joints and obtain a more compact intra-class feature distribution. Based on CVSTA, we construct a Multi-Dimensional Refinement Graph Convolutional Network (MDR-GCN), which can improve the discrimination among channel-, joint- and frame-level features for fine-grained actions. Furthermore, we propose a Robust Decouple Loss (RDL), which significantly boosts the effect of the CVSTA and reduces the impact of noise. The proposed method combining MDR-GCN with RDL outperforms the known state-of-the-art skeleton-based approaches on fine-grained datasets, FineGym99 and FSD-10, and also on the coarse dataset NTU-RGB+D X-view version.
更多查看译文
关键词
action,recognition,multi-dimensional,fine-grained,skeleton-based
AI 理解论文
溯源树
样例
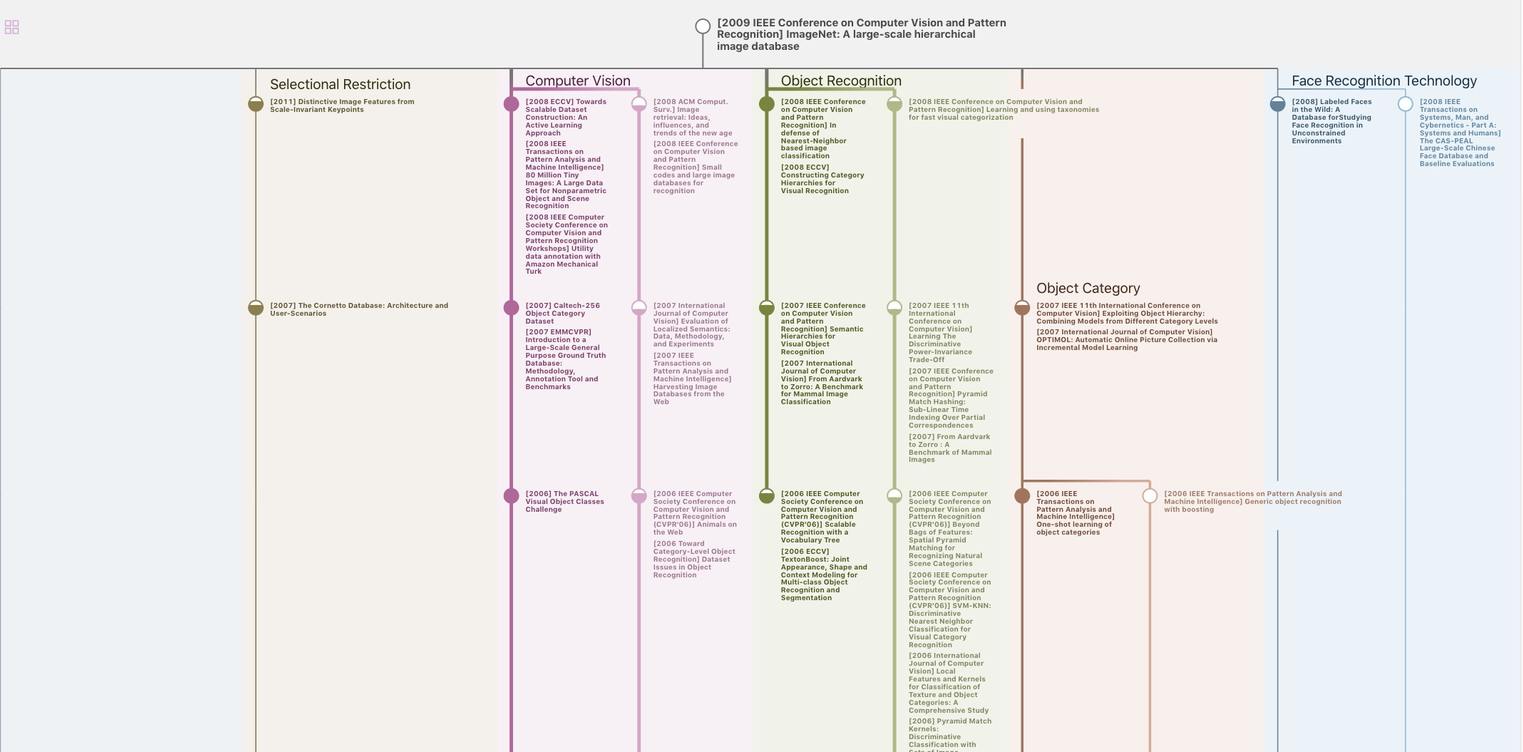
生成溯源树,研究论文发展脉络
Chat Paper
正在生成论文摘要