Hybrid scheme through read-first-LSTM encoder-decoder and broad learning system for bearings degradation monitoring and remaining useful life estimation
Advanced Engineering Informatics(2023)
摘要
This paper proposes a novel hybrid scheme through read-first-LSTM (RLSTM) encoder-decoder and broad learning system (BLS) for bearings degradation monitoring and remaining useful life (RUL) estimation, which aims to describe the nonlinear characteristics of the degradation process. Firstly, the raw signals are processed premier by complete ensemble empirical mode decomposition with adaptive noise (CEEMDAN) and a novel dimensionality reduction method composed of t-distribution stochastic neighbor embedding (t-SNE) and density-based spatial clustering of application with noise algorithm (DBSCAN). Then, the health indicator is constructed with the Hilbert-Huang transform (HHT) corresponding to the bearings’ natural fault frequency, which can be employed as the hybrid scheme training label. Linear rectification technology (LRT) and exponentially weighted moving average (EWMA) control chart are adapted to define the exact process of the degradation. Secondly, a novel RLSTM is proposed. And simultaneously, an encoder-decoder model, where RLSTM is utilized as an encoder, and LSTM is adopted as a decoder, is designed for degradation monitoring. Finally, a broad learning system (BLS), which differs from deep learning with a deeper structure, is established in a flat network to estimate the RUL of bearings. Compared with the state-of-the-art techniques, the better efficacy of the proposed hybrid scheme is illustrated using the PRONOSTIA platform dataset.
更多查看译文
关键词
Read-first-LSTM, Broad learning system, Feature extraction, Prognostics and health management, Remaining useful life
AI 理解论文
溯源树
样例
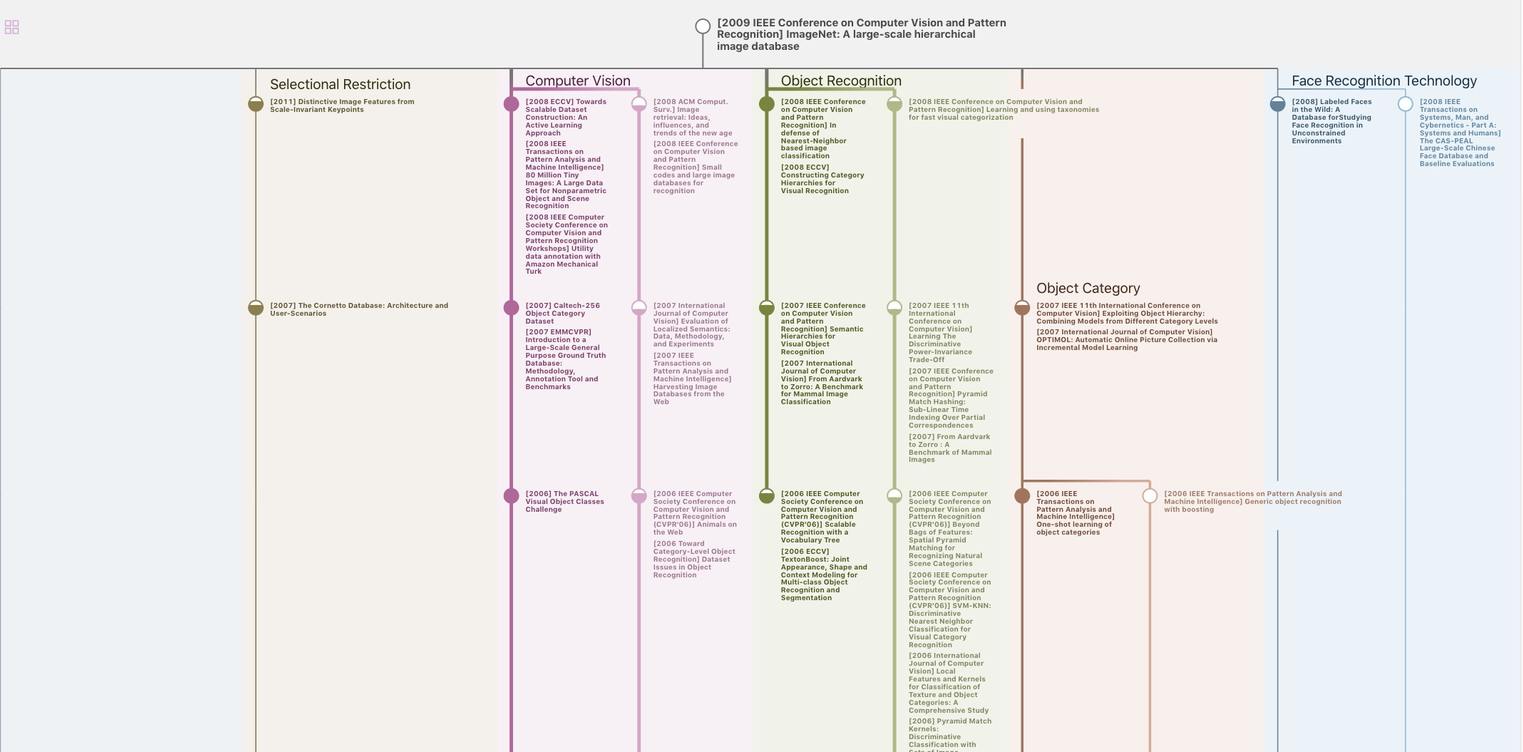
生成溯源树,研究论文发展脉络
Chat Paper
正在生成论文摘要