Testing Textural Information Base on LiDAR and Hyperspectral Data for Mapping Wetland Vegetation: A Case Study of Warta River Mouth National Park (Poland).
Remote Sensing(2023)
摘要
One of the key issues in wetland monitoring is mapping vegetation. Remote sensing and machine learning are used to acquire vegetation maps, which, due to the development of sensors and data processing and analysis methods, have increasingly high accuracy. The objectives of this study were to test: (i) which of the textural information (TI) features have the highest information potential for identifying wetland communities; and (ii) whether the use of TI improves the accuracy of wetland communities mapping using hyperspectral (HS) and Airborne Laser Scanning (ALS) data. The analysis indicated that the mean and entropy features of the Gray Level Co-occurrence Matrix had the highest potential to differentiate between various wetland communities. Adding these features to the dataset resulted in a small increase (0.005) in average F1 accuracy based on HS data and 0.011 for HS and ALS scenarios in wetland communities classification, and adding TI improved the delineation of patch boundaries. A higher increase was noted for forest and scrub vegetation (by 0.019 for the HS scenario and 0.022 for the HS and ALS scenario) and rushes (only for the HS and ALS scenario 0.017). It can be concluded that it is reasonable to use textural information for mapping wetland communities, especially for areas with a high proportion of scrub and forest and rushes vegetation included in the analysis.
更多查看译文
关键词
ALS, machine learning, classification, data fusion, floodplain, Permutation Importance, CatBoost, HySpex, GLCM
AI 理解论文
溯源树
样例
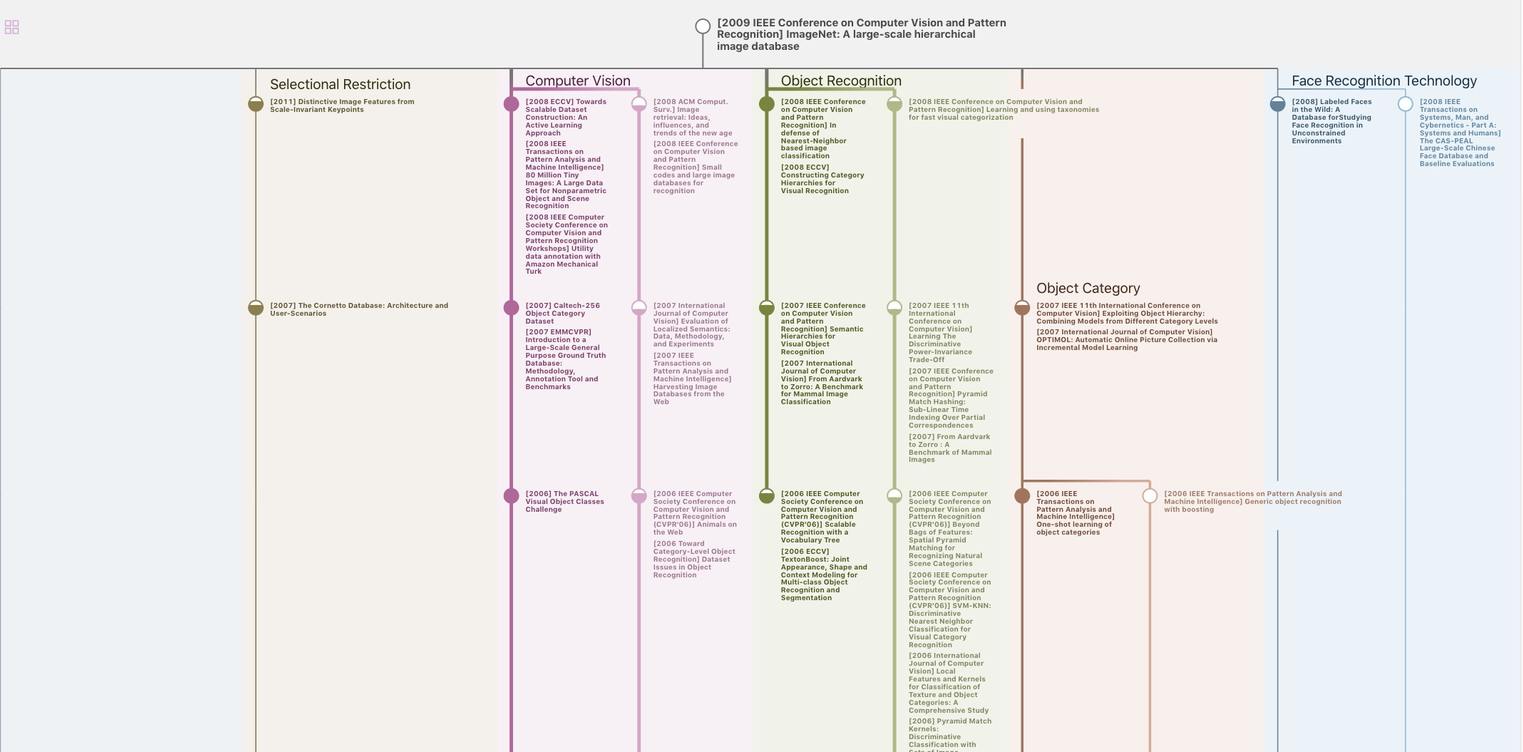
生成溯源树,研究论文发展脉络
Chat Paper
正在生成论文摘要