Efficient solutions of fermionic systems using artificial neural networks
Frontiers in Physics(2023)
摘要
In this study, we explore the similarities and differences between variational Monte Carlo techniques that employ conventional and artificial neural network representations of the ground-state wave function for fermionic systems. Our primary focus is on shallow neural network architectures, specifically the restricted Boltzmann machine, and we examine unsupervised learning algorithms that are appropriate for modeling complex many-body correlations. We assess the advantages and drawbacks of conventional and neural network wave functions by applying them to a range of circular quantum dot systems. Our findings, which include results for systems containing up to 90 electrons, emphasize the efficient implementation of these methods on both homogeneous and heterogeneous high-performance computing facilities.
更多查看译文
关键词
quantum dots,many-body physics,Monte Carlo methods,machine learning,Boltzmann machines
AI 理解论文
溯源树
样例
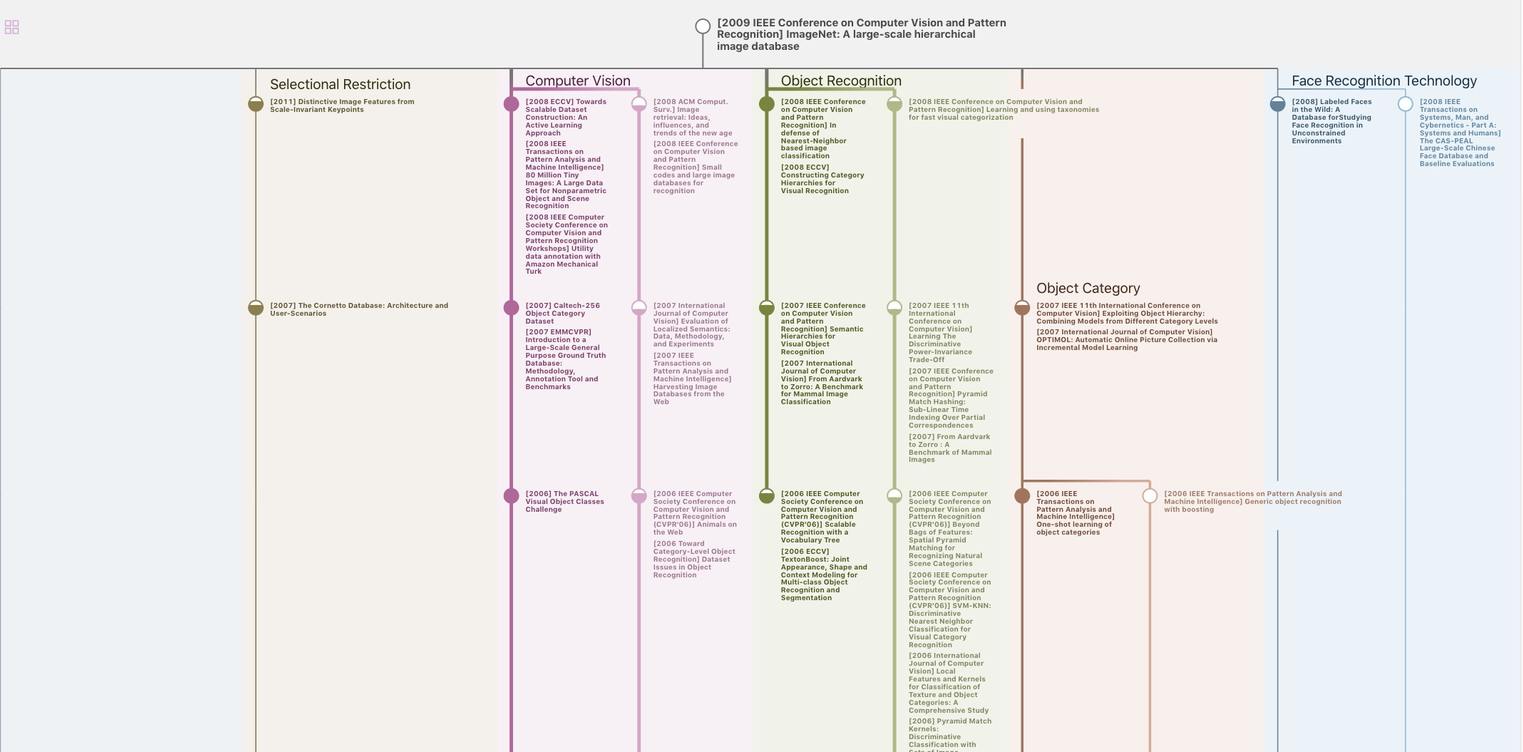
生成溯源树,研究论文发展脉络
Chat Paper
正在生成论文摘要