A Simple and Effective Baseline for Attentional Generative Adversarial Networks
CoRR(2023)
摘要
Synthesising a text-to-image model of high-quality images by guiding the generative model through the Text description is an innovative and challenging task. In recent years, AttnGAN based on the Attention mechanism to guide GAN training has been proposed, SD-GAN, which adopts a self-distillation technique to improve the performance of the generator and the quality of image generation, and Stack-GAN++, which gradually improves the details and quality of the image by stacking multiple generators and discriminators. However, this series of improvements to GAN all have redundancy to a certain extent, which affects the generation performance and complexity to a certain extent. We use the popular simple and effective idea (1) to remove redundancy structure and improve the backbone network of AttnGAN. (2) to integrate and reconstruct multiple losses of DAMSM. Our improvements have significantly improved the model size and training efficiency while ensuring that the model's performance is unchanged and finally proposed our \textbf{SEAttnGAN}. Code is avalilable at https://github.com/jmyissb/SEAttnGAN.
更多查看译文
关键词
attentional generative adversarial
AI 理解论文
溯源树
样例
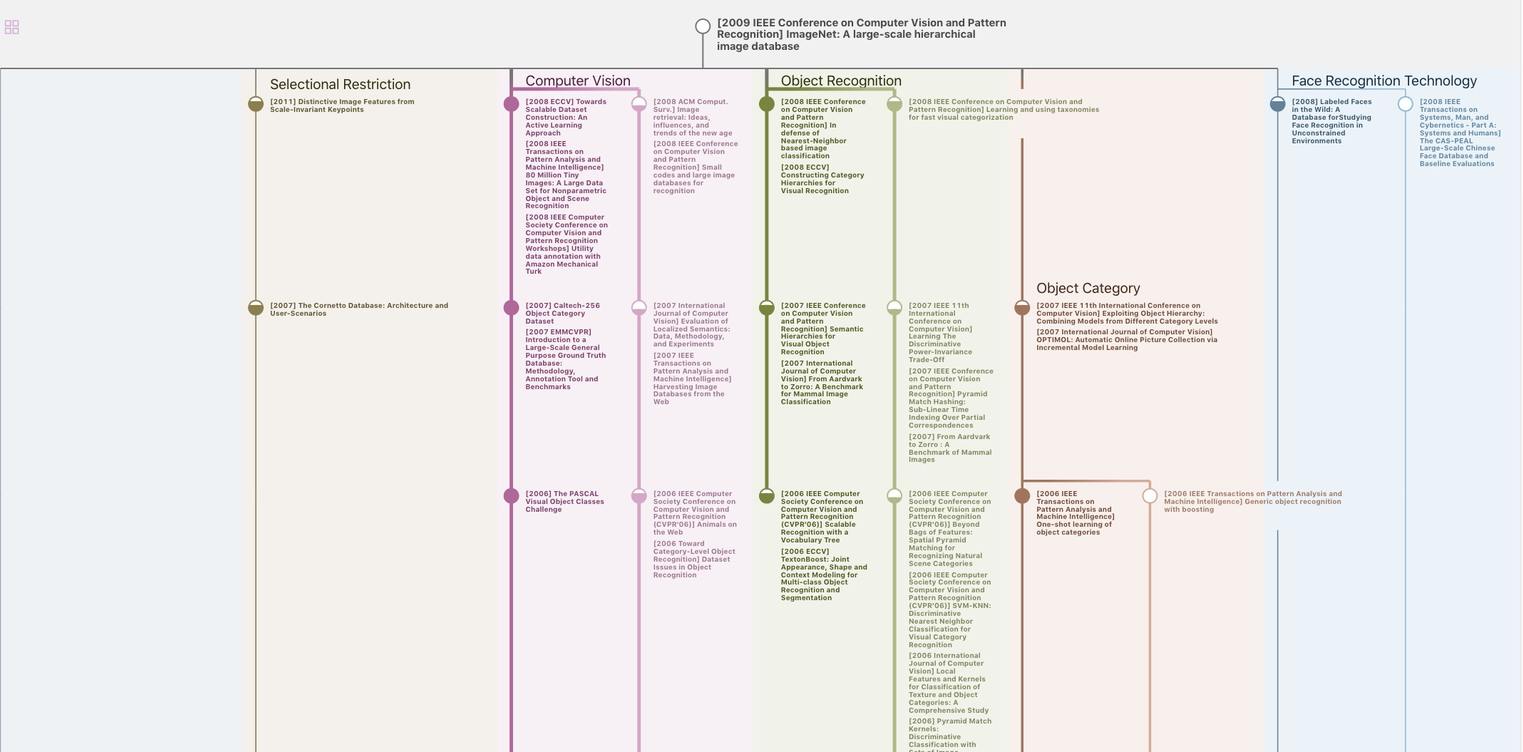
生成溯源树,研究论文发展脉络
Chat Paper
正在生成论文摘要