Mono-to-Stereo Through Parametric Stereo Generation.
CoRR(2023)
摘要
Generating a stereophonic presentation from a monophonic audio signal is a challenging open task, especially if the goal is to obtain a realistic spatial imaging with a specific panning of sound elements. In this work, we propose to convert mono to stereo by means of predicting parametric stereo (PS) parameters using both nearest neighbor and deep network approaches. In combination with PS, we also propose to model the task with generative approaches, allowing to synthesize multiple and equally-plausible stereo renditions from the same mono signal. To achieve this, we consider both autoregressive and masked token modelling approaches. We provide evidence that the proposed PS-based models outperform a competitive classical decorrelation baseline and that, within a PS prediction framework, modern generative models outshine equivalent non-generative counterparts. Overall, our work positions both PS and generative modelling as strong and appealing methodologies for mono-to-stereo upmixing. A discussion of the limitations of these approaches is also provided.
更多查看译文
关键词
parametric mono-to-stereo generation
AI 理解论文
溯源树
样例
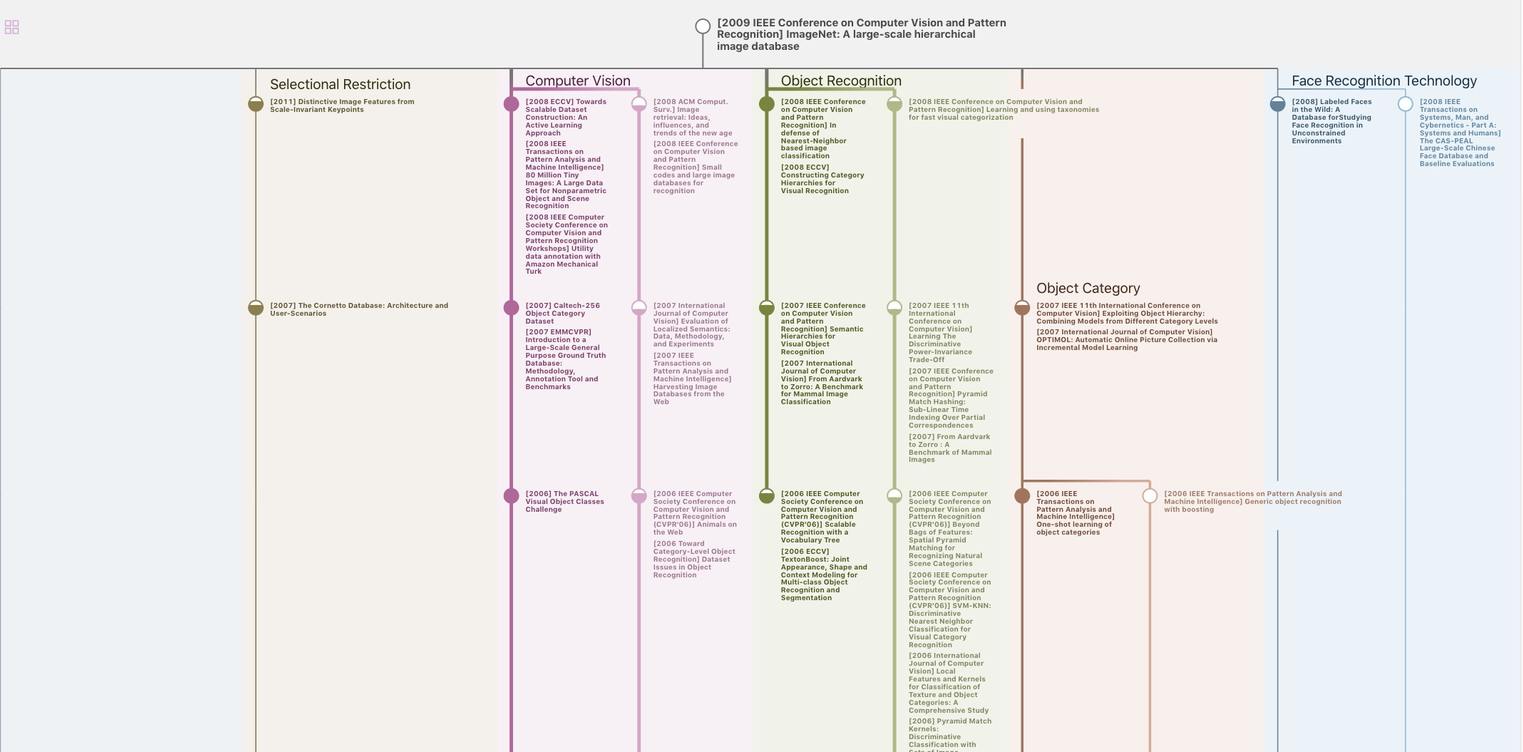
生成溯源树,研究论文发展脉络
Chat Paper
正在生成论文摘要