Multi-output Ensembles for Multi-step Forecasting
CoRR(2023)
摘要
This paper studies the application of ensembles composed of multi-output models for multi-step ahead forecasting problems. Dynamic ensembles have been commonly used for forecasting. However, these are typically designed for one-step-ahead tasks. On the other hand, the literature regarding the application of dynamic ensembles for multi-step ahead forecasting is scarce. Moreover, it is not clear how the combination rule is applied across the forecasting horizon. We carried out extensive experiments to analyze the application of dynamic ensembles for multi-step forecasting. We resorted to a case study with 3568 time series and an ensemble of 30 multi-output models. We discovered that dynamic ensembles based on arbitrating and windowing present the best performance according to average rank. Moreover, as the horizon increases, most approaches struggle to outperform a static ensemble that assigns equal weights to all models. The experiments are publicly available in a repository.
更多查看译文
关键词
multi-output,multi-step
AI 理解论文
溯源树
样例
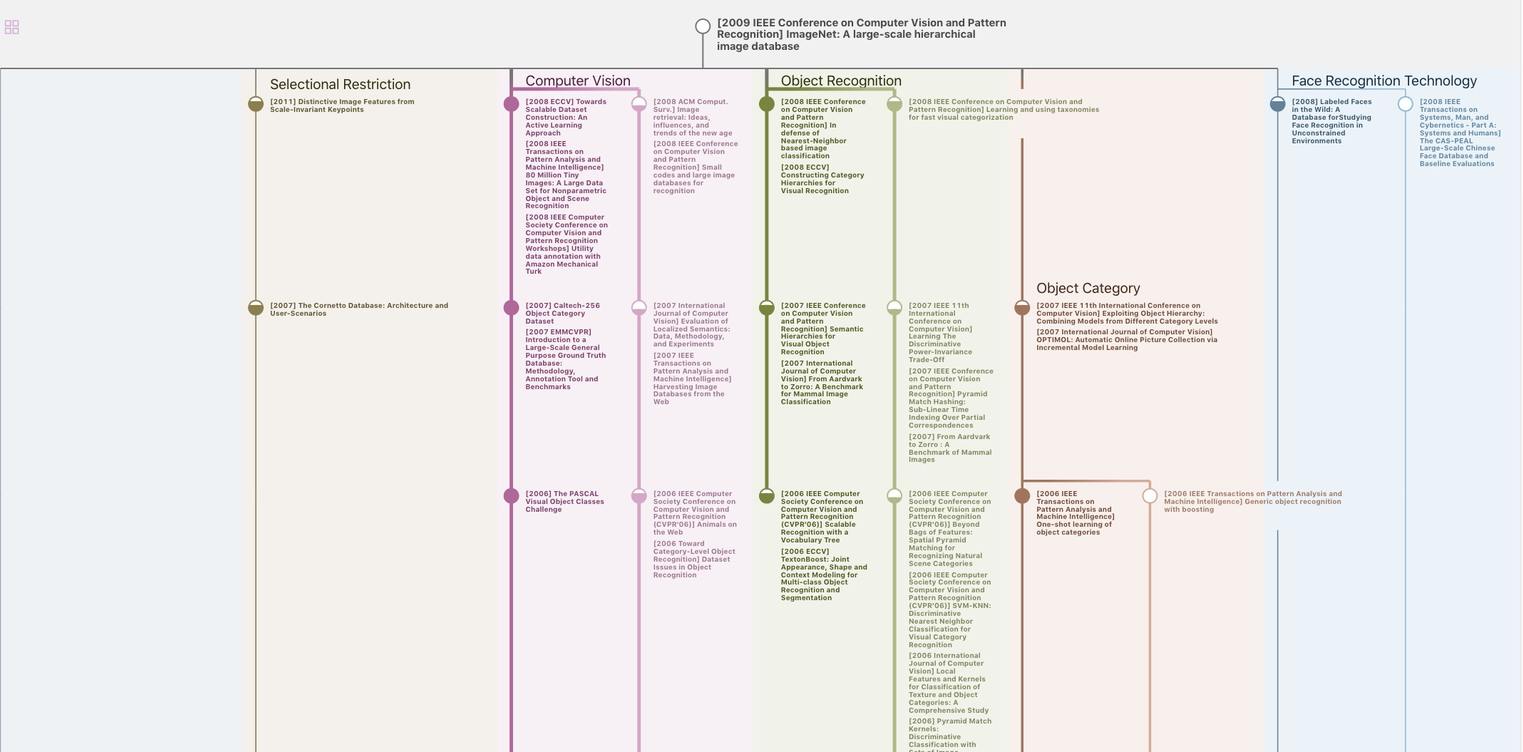
生成溯源树,研究论文发展脉络
Chat Paper
正在生成论文摘要