Federated Learning on Non-iid Data via Local and Global Distillation
CoRR(2023)
摘要
Most existing federated learning algorithms are based on the vanilla FedAvg scheme. However, with the increase of data complexity and the number of model parameters, the amount of communication traffic and the number of iteration rounds for training such algorithms increases significantly, especially in non-independently and homogeneously distributed scenarios, where they do not achieve satisfactory performance. In this work, we propose FedND: federated learning with noise distillation. The main idea is to use knowledge distillation to optimize the model training process. In the client, we propose a self-distillation method to train the local model. In the server, we generate noisy samples for each client and use them to distill other clients. Finally, the global model is obtained by the aggregation of local models. Experimental results show that the algorithm achieves the best performance and is more communication-efficient than state-of-the-art methods.
更多查看译文
关键词
federated learning,knowledge distillation
AI 理解论文
溯源树
样例
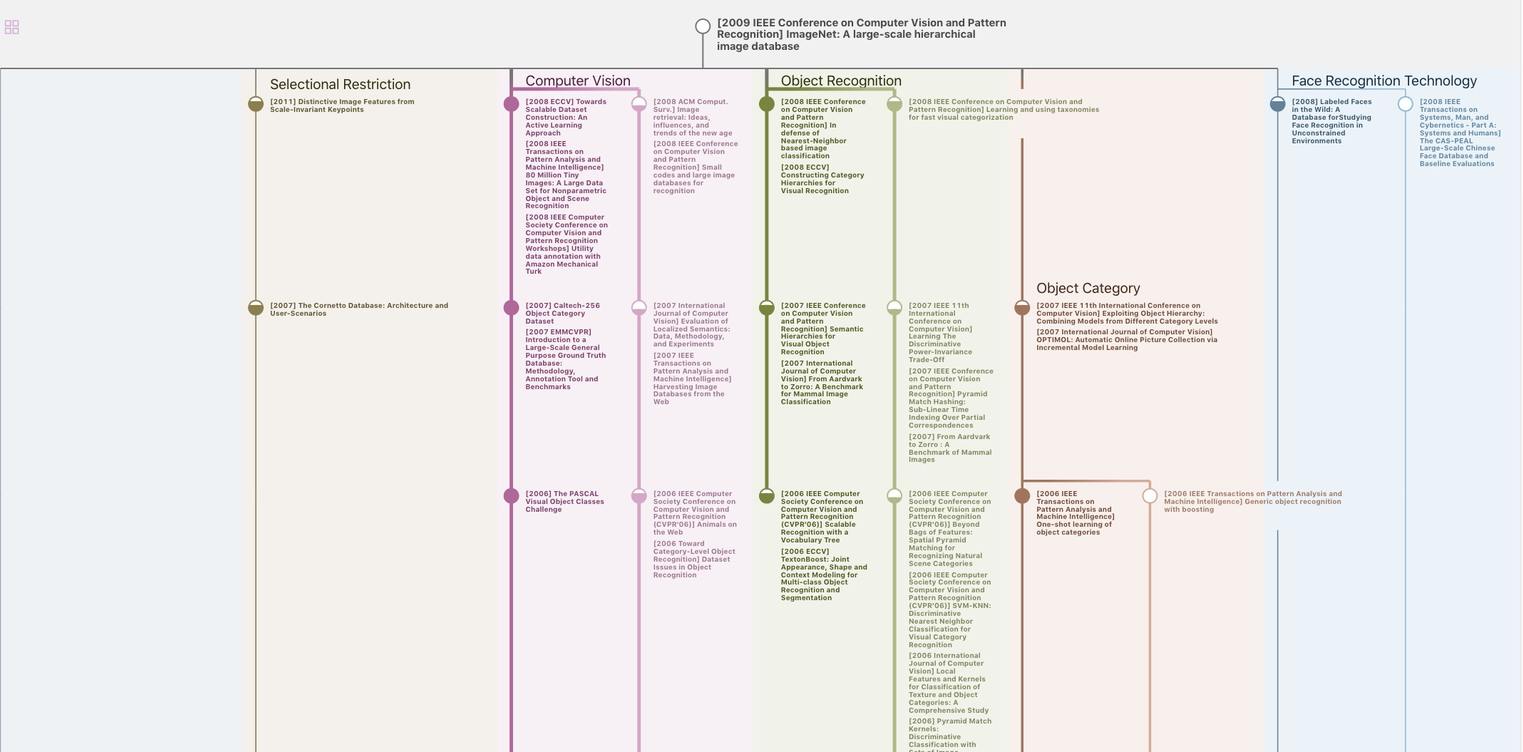
生成溯源树,研究论文发展脉络
Chat Paper
正在生成论文摘要