Weakly supervised scene text generation for low-resource languages
EXPERT SYSTEMS WITH APPLICATIONS(2024)
摘要
A large number of annotated training images is crucial for training successful scene text recognition models. However, collecting sufficient datasets can be a labor-intensive and costly process, particularly for low-resource languages. To address this challenge, auto-generating text data has shown promise in alleviating the problem. Unfortunately, existing scene text generation methods typically rely on a large amount of paired data, which is difficult to obtain for low-resource languages. In this paper, we propose a novel weakly supervised scene text generation method that leverages a few recognition-level labels as weak supervision. The proposed method can generate a large amount of scene text images with diverse backgrounds and font styles through cross -language generation. Our method disentangles the content and style features of scene text images, with the former representing textual information and the latter representing characteristics such as font, alignment, and background. To preserve the complete content structure of generated images, we introduce an integrated attention module. Furthermore, to bridge the style gap in the style of different languages, we incorporate a pre-trained font classifier. We evaluate our method using state-of-the-art scene text recognition models. Experiments demonstrate that our generated scene text significantly improves the scene text recognition accuracy and helps achieve higher accuracy when complemented with other generative methods.
更多查看译文
关键词
Scene text generation,Style transfer,Low-resource languages
AI 理解论文
溯源树
样例
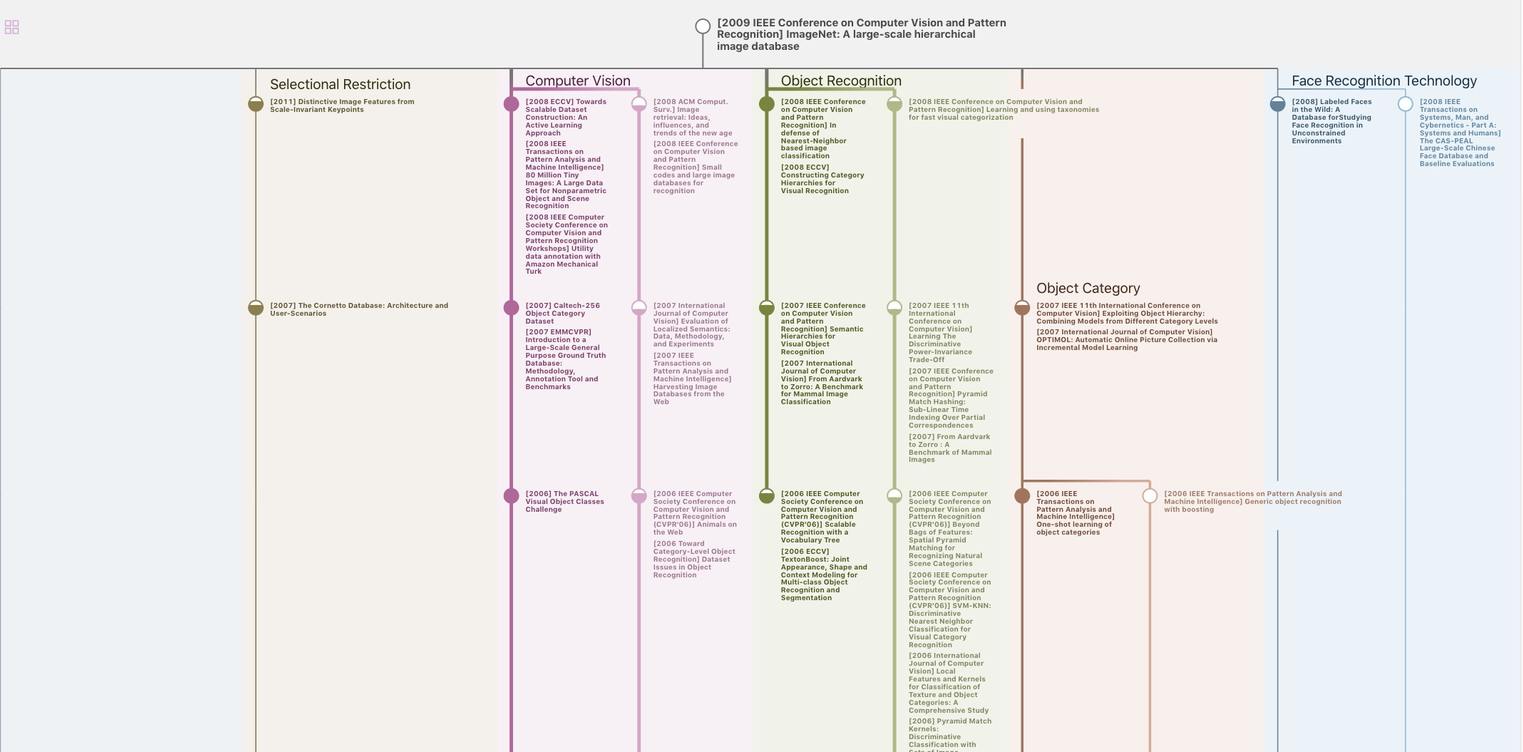
生成溯源树,研究论文发展脉络
Chat Paper
正在生成论文摘要