SHISRCNet: Super-Resolution and Classification Network for Low-Resolution Breast Cancer Histopathology Image
MEDICAL IMAGE COMPUTING AND COMPUTER ASSISTED INTERVENTION, MICCAI 2023, PT V(2023)
摘要
The rapid identification and accurate diagnosis of breast cancer, known as the killer of women, have become greatly significant for those patients. Numerous breast cancer histopathological image classification methods have been proposed. But they still suffer from two problems. (1) These methods can only hand high-resolution (HR) images. However, the low-resolution (LR) images are often collected by the digital slide scanner with limited hardware conditions. Compared with HR images, LR images often lose some key features like texture, which deeply affects the accuracy of diagnosis. (2) The existing methods have fixed receptive fields, so they can not extract and fuse multi-scale features well for images with different magnification factors. To fill these gaps, we present a Single Histopathological Image Super-Resolution Classification network (SHISRCNet), which consists of two modules: Super-Resolution (SR) and Classification (CF) modules. SR module reconstructs LR images into SR ones. CF module extracts and fuses the multi-scale features of SR images for classification. In the training stage, we introduce HR images into the CF module to enhance SHIS-RCNet's performance. Finally, through the joint training of these two modules, super-resolution and classified of LR images are integrated into our model. The experimental results demonstrate that the effects of our method are close to the SOTA methods with taking HR images as inputs.
更多查看译文
关键词
breast cancer,histopathological image,super-resolution,classification,joint training
AI 理解论文
溯源树
样例
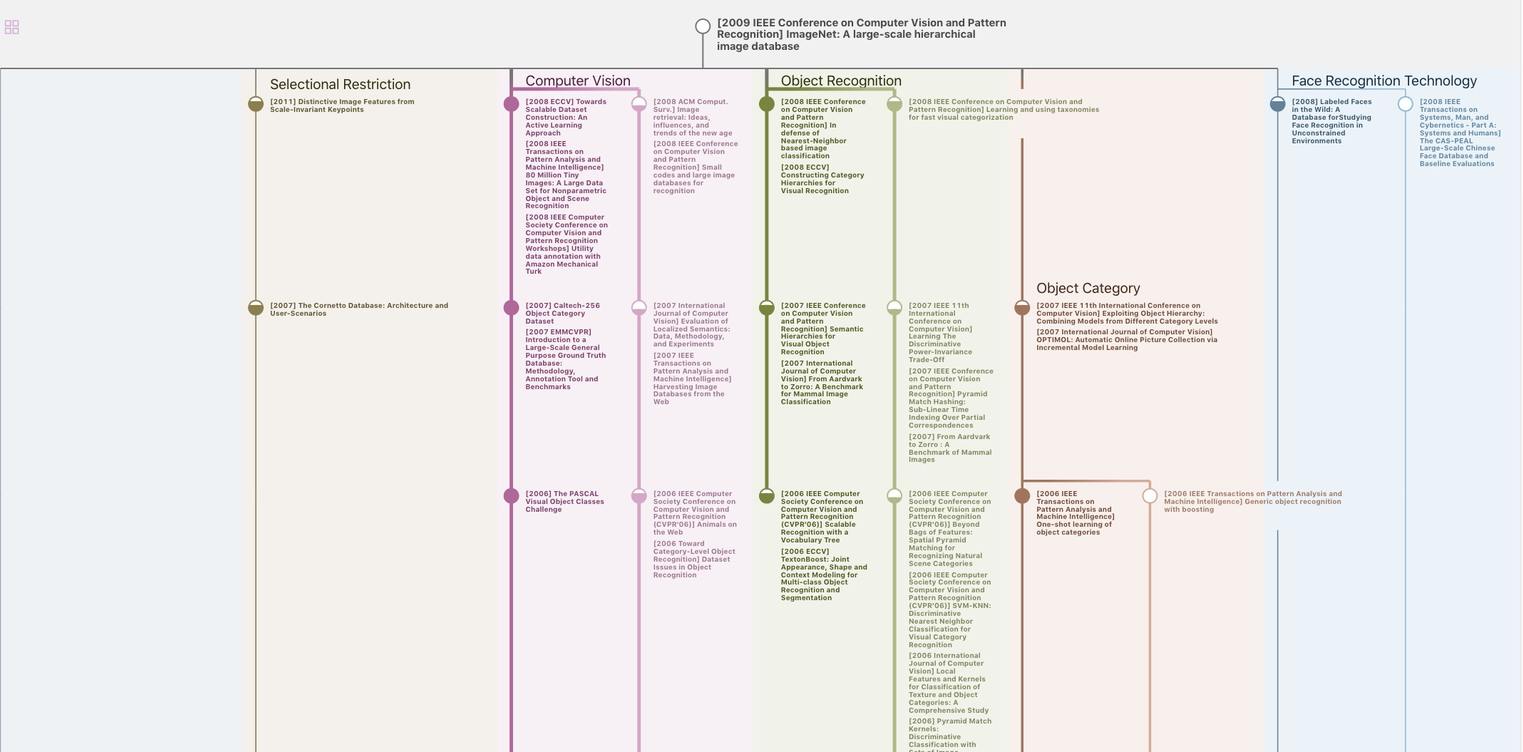
生成溯源树,研究论文发展脉络
Chat Paper
正在生成论文摘要